Develop&validate SuperAlarm to detect patient deterioration with few false alarms
发展
基本信息
- 批准号:8943567
- 负责人:
- 金额:$ 53.13万
- 依托单位:
- 依托单位国家:美国
- 项目类别:
- 财政年份:2015
- 资助国家:美国
- 起止时间:2015-07-20 至 2019-04-30
- 项目状态:已结题
- 来源:
- 关键词:
项目摘要
DESCRIPTION (provided by applicant): Multi-parameter patient monitoring in intensive care units (ICU) remains unsatisfactory as evidenced by the well-known alarm fatigue problem. It has been reported that a critical care patient could generate 700 alarms per day. At UCSF, a daily average of 187 audible alarms per bed occurred in ICUs during one month of assessment. Improving signal processing algorithms, fine-tuning alarm thresholds, and downgrading some alarms to an inaudible category are some ways to address alarm fatigue. However, these interventions attempt to solve the problem within the context of conventional patient monitoring practice (i.e., the focus is on an individual alarm while ignoring the relationships among alarms, the contextual information established by other data available in electronic health record (EHR), and the sequential patterns of all of these variables). In fact, we argue that alarm fatigue reflecs a deeper challenge for critical care clinicians who are overloaded with increasingly available raw data but do not have appropriate tools to leverage the potential of these data to treat their patients. As a first step to support clinicians to overcome data overload, our goal is to precisely
detect gross patient state changes by recognizing combinatorial and sequential patterns among individual alarms, physiological variables, and EHR data. Achieving this goal will lead to developing additional decision support tools to understand the causes and select potential interventions for the detected patient state changes. Our group has done preliminary studies that demonstrate the feasibility of achieving this goal. In particular, we have evolved a specific algorithm to identify co-occurring monitor alarms, which frequently precede in-hospital cardiopulmonary arrests (CPA) but rarely occur among control patients, to a data fusion framework. This framework is capable of recognizing predictive combinations of a much richer set of variables including lab tests and additional physiological variables not available from monitors. We term these combinations SuperAlarm patterns. By construction, SuperAlarm triggers will occur much less frequently than monitor alarms, yet be more precise in detecting patient state changes. Thus, the objective of this application is to develop and validate further algorithm improvement under this SuperAlarm data fusion framework using prospective data. We will pursue the following three aims: 1) To enrich SuperAlarm patterns by novel analysis of Electrocardiographic (ECG) signals; 2) To develop sequential pattern recognition methods for sequences of SuperAlarm triggers. 3) To conduct prospective data collection to develop and validate SuperAlarm model.
描述(由适用提供):重症监护病房(ICU)中的多参数患者监测仍然不令人满意,这是众所周知的警报疲劳问题所证明的。据报道,重症监护患者每天可以发出700个警报。在UCSF,在评估的一个月中,ICU中每天平均每天有187张可听见的警报。改善信号处理算法,微调警报阈值并将某些警报降低到不可察觉的类别是解决警报疲劳的某些方法。但是,这些干预措施试图在传统的患者监测实践的背景下解决该问题(即,重点是个人警报,同时忽略了警报之间的关系,电子健康记录中其他可用数据(EHR)中其他数据建立的上下文信息以及所有这些变量的顺序模式)。实际上,我们认为,警报疲劳反射了对重症监护临床医生的更深层次挑战,这些临床医生的原始数据越来越多,但没有适当的工具来利用这些数据的潜力来治疗患者。作为支持临床医生克服数据超载的第一步,我们的目标是确切
通过识别单个警报,物理变量和EHR数据之间的组合和顺序模式来检测患者状态的变化。实现这一目标将导致开发其他决策支持工具,以了解原因并为检测到的患者国家变化选择潜在的干预措施。我们的小组进行了初步研究,证明了实现这一目标的可行性。特别是,我们已经进化了一种特定的算法,以识别同时发生的监测警报,该警报经常在院内心肺骤停(CPA)之前(CPA),但在对照患者之间很少发生到数据融合框架。该框架能够识别一组更丰富的变量的预测组合,包括实验室测试和监视器无法获得的其他物理变量。我们将这些组合称为“超级武器”模式。通过施工,超级武器触发器的发生频率要比监视警报少得多,但是在检测患者状态变化时要精确。这是本应用程序的目的是使用前瞻性数据在此SuperAlarm数据融合框架下开发和验证进一步的算法改进。我们将追求以下三个目标:1)通过新型心电图(ECG)信号分析来丰富超级武器模式; 2)开发用于超级触发器序列的顺序模式识别方法。 3)进行前瞻性数据收集以开发和验证超级武器模型。
项目成果
期刊论文数量(0)
专著数量(0)
科研奖励数量(0)
会议论文数量(0)
专利数量(0)
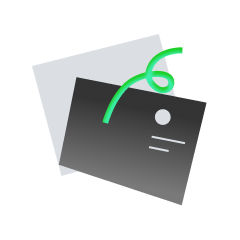
暂无数据
数据更新时间:2024-06-01
Xiao Hu的其他基金
Novel Algorithm and Data Strategies to detect and Predict atrial fibrillation for post-stroke patients (NADSP)
用于检测和预测中风后患者心房颤动的新算法和数据策略 (NADSP)
- 批准号:1056110810561108
- 财政年份:2023
- 资助金额:$ 53.13万$ 53.13万
- 项目类别:
Integrate Dynamic System Model and Machine Learning for Calibration-Free Noninvasive ICP
集成动态系统模型和机器学习,实现免校准无创 ICP
- 批准号:1060023910600239
- 财政年份:2020
- 资助金额:$ 53.13万$ 53.13万
- 项目类别:
Learning to Predict Delayed Cerebral Ischemia with Novel Continuous Cerebral Arterial State Index
学习用新型连续脑动脉状态指数预测迟发性脑缺血
- 批准号:1040637810406378
- 财政年份:2020
- 资助金额:$ 53.13万$ 53.13万
- 项目类别:
Learning to Predict Delayed Cerebral Ischemia with Novel Continuous Cerebral Arterial State Index
学习用新型连续脑动脉状态指数预测迟发性脑缺血
- 批准号:1059971710599717
- 财政年份:2020
- 资助金额:$ 53.13万$ 53.13万
- 项目类别:
Integrate Dynamic System Model and Machine Learning for Calibration-Free Noninvasive ICP
集成动态系统模型和机器学习,实现免校准无创 ICP
- 批准号:1021968310219683
- 财政年份:2020
- 资助金额:$ 53.13万$ 53.13万
- 项目类别:
Learning to Predict Delayed Cerebral Ischemia with Novel Continuous Cerebral Arterial State Index
学习用新型连续脑动脉状态指数预测迟发性脑缺血
- 批准号:1025134810251348
- 财政年份:2020
- 资助金额:$ 53.13万$ 53.13万
- 项目类别:
Integrate Dynamic System Model and Machine Learning for Calibration-Free Noninvasive ICP
集成动态系统模型和机器学习,实现免校准无创 ICP
- 批准号:1022876810228768
- 财政年份:2020
- 资助金额:$ 53.13万$ 53.13万
- 项目类别:
Integrate Dynamic System Model and Machine Learning for Calibration-Free Noninvasive ICP
集成动态系统模型和机器学习,实现免校准无创 ICP
- 批准号:97645119764511
- 财政年份:2018
- 资助金额:$ 53.13万$ 53.13万
- 项目类别:
Develop&validate SuperAlarm to detect patient deterioration with few false alarms
发展
- 批准号:92686869268686
- 财政年份:2015
- 资助金额:$ 53.13万$ 53.13万
- 项目类别:
ICP Elevation Alerting Based on a Predictive Model Hosting Platform
基于预测模型托管平台的 ICP 海拔警报
- 批准号:87327118732711
- 财政年份:2012
- 资助金额:$ 53.13万$ 53.13万
- 项目类别:
相似国自然基金
采用复合防护材料的水下多介质耦合作用下重力坝抗爆机理研究
- 批准号:51779168
- 批准年份:2017
- 资助金额:59.0 万元
- 项目类别:面上项目
采用数值计算求解一类半代数系统全部整数解
- 批准号:11671377
- 批准年份:2016
- 资助金额:48.0 万元
- 项目类别:面上项目
采用pinball loss的MEE算法研究
- 批准号:11401247
- 批准年份:2014
- 资助金额:23.0 万元
- 项目类别:青年科学基金项目
采用路径算法和管网简化的城市内涝近实时模拟
- 批准号:41301419
- 批准年份:2013
- 资助金额:25.0 万元
- 项目类别:青年科学基金项目
采用ε近似算法的盲信道均衡
- 批准号:60172058
- 批准年份:2001
- 资助金额:16.0 万元
- 项目类别:面上项目
相似海外基金
Applying Computational Phenotypes To Assess Mental Health Disorders Among Transgender Patients in the United States
应用计算表型评估美国跨性别患者的心理健康障碍
- 批准号:1060472310604723
- 财政年份:2023
- 资助金额:$ 53.13万$ 53.13万
- 项目类别:
Brain Digital Slide Archive: An Open Source Platform for data sharing and analysis of digital neuropathology
Brain Digital Slide Archive:数字神经病理学数据共享和分析的开源平台
- 批准号:1073556410735564
- 财政年份:2023
- 资助金额:$ 53.13万$ 53.13万
- 项目类别:
Toward Accurate Cardiovascular Disease Prediction in Hispanics/Latinos: Modeling Risk and Resilience Factors
实现西班牙裔/拉丁裔的准确心血管疾病预测:风险和弹性因素建模
- 批准号:1085231810852318
- 财政年份:2023
- 资助金额:$ 53.13万$ 53.13万
- 项目类别:
Unified, Scalable, and Reproducible Neurostatistical Software
统一、可扩展且可重复的神经统计软件
- 批准号:1072550010725500
- 财政年份:2023
- 资助金额:$ 53.13万$ 53.13万
- 项目类别:
Single viewpoint panoramic imaging technology for colonoscopy
肠镜单视点全景成像技术
- 批准号:1058016510580165
- 财政年份:2023
- 资助金额:$ 53.13万$ 53.13万
- 项目类别: