EAGER: A Comprehensive Approach for Generating, Sharing, Searching, and Using High-Resolution Terrain Parameters
EAGER:生成、共享、搜索和使用高分辨率地形参数的综合方法
基本信息
- 批准号:2334945
- 负责人:
- 金额:$ 22.5万
- 依托单位:
- 依托单位国家:美国
- 项目类别:Standard Grant
- 财政年份:2023
- 资助国家:美国
- 起止时间:2023-10-01 至 2025-09-30
- 项目状态:未结题
- 来源:
- 关键词:
项目摘要
Terrain parameters quantitatively describe a landscape's surface properties (for example, slope or topographic wetness). Terrain parameters hold significant potential for advancing climate-related science and engineering efforts. As terrain parameters can be generated at different spatial resolutions, they are valuable resources for scientists working on soil moisture prediction, fire propagation, estimation of soil carbon content, soil respiration, and hydrology. These applications are critical for understanding land-atmosphere interactions and mitigating the impacts of climate change across ecosystems and landscapes. However, the process of deriving terrain parameters from digital elevation models is accurate, but expensive in terms of computational resources and time. For more efficient generation of terrain parameters, this project implements a flexible workflow to generate terrain parameter datasets at different resolutions for different regions of interest. All the products of this project (data, metadata, and software) are stored in an open-access commons to ensure they are Findable, Accessible, Interoperable, and Reusable (FAIR). The team of researchers promotes increased participation of underrepresented students, particularly women, through mentoring students in Systers (the organization for women in Electrical Engineering and Computer Science at the University of Tennessee Knoxville) and the collaboration with the Women in Data Science (WiDS) at Stanford.Terrain parameters are derived from Digital Elevation Models. High-resolution terrain parameters enable accurate spatial analyses and decision-making in climate-related science and engineering domains, but generating high-resolution data is computationally expensive, hindering the usability of terrain parameters for multiple applications. The project addresses this challenge to make terrain parameters available for climate study in three ways. First, the project implements a workflow to generate 15 terrain parameters at any resolution (from 30 km to 3 m) while preserving performance and accuracy. Performance is evaluated by measuring wall times and memory usage cloud platforms. The accuracy is validated by comparing the data with the derived terrain parameters. Second, the project uses the workflow and exploits data parallelism to generate large high-resolution datasets (i.e., down to 3 m) for North America (i.e., Canada, the United States, and Mexico). The project deliverable comprises rich metadata annotating the parameter values and Jupyter Notebooks for data search and access, reproducible data generation, accuracy validation, and performance measurement. Third, by bringing together an interdisciplinary research team of leading scientists with experience in data science and soil moisture dynamics, the project facilitates collaboration among federal agencies (including NSF, NASA, and USDA, among others) and institutions to pursue interdisciplinary research, share insights, and deliver innovative solutions for climate-related issues.This award reflects NSF's statutory mission and has been deemed worthy of support through evaluation using the Foundation's intellectual merit and broader impacts review criteria.
地形参数定量地描述了景观的表面特性(例如,坡度或地形湿度)。地形参数具有推进与气候相关的科学和工程工作的巨大潜力。由于可以在不同的空间分辨率下生成地形参数,因此对于从事土壤水分预测,火灾繁殖,土壤碳含量,土壤呼吸和水文学的估计的科学家来说,它们是宝贵的资源。这些应用对于理解土地 - 大气相互作用以及减轻生态系统和景观气候变化的影响至关重要。但是,从数字高程模型中得出地形参数的过程是准确的,但在计算资源和时间方面昂贵。为了更有效地生成地形参数,该项目实现了灵活的工作流程,以在不同分辨率的不同分辨率下生成地形参数数据集。该项目的所有产品(数据,元数据和软件)都存储在开放式公共场中,以确保它们可以找到,可访问,可互操作和可重复使用(公平)。研究人员团队通过指导Systers(田纳西·诺克斯维尔大学电气工程和计算机科学的女性组织)和与Stanford的妇女合作的指导学生(Terrain.Terrain参数来自数字高度标准模型),从而促进了代表性不足的学生,尤其是女性的参与。高分辨率的地形参数可以在与气候相关的科学和工程领域中进行准确的空间分析和决策,但是生成高分辨率数据在计算上是昂贵的,从而阻碍了多个应用程序的地形参数的可用性。该项目解决了这一挑战,以使地形参数以三种方式用于气候研究。首先,该项目实现工作流程,以任何分辨率(从30 km到3 m)生成15个地形参数,同时保持性能和准确性。通过测量墙壁时间和内存使用云平台来评估性能。通过将数据与派生的地形参数进行比较来验证精度。其次,该项目使用工作流程并利用数据并行性来为北美(即加拿大,美国和墨西哥)生成大型高分辨率数据集(即降至3 m)。该项目可交付的元数据包括注释参数值和jupyter笔记本,以进行数据搜索和访问,可再现的数据生成,准确性验证和性能测量。 Third, by bringing together an interdisciplinary research team of leading scientists with experience in data science and soil moisture dynamics, the project facilitates collaboration among federal agencies (including NSF, NASA, and USDA, among others) and institutions to pursue interdisciplinary research, share insights, and deliver innovative solutions for climate-related issues.This award reflects NSF's statutory mission and has been deemed worthy of support通过使用基金会的智力优点和更广泛影响的评论标准进行评估。
项目成果
期刊论文数量(0)
专著数量(0)
科研奖励数量(0)
会议论文数量(0)
专利数量(0)
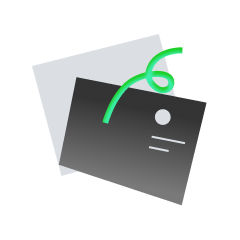
暂无数据
数据更新时间:2024-06-01
Michela Taufer其他文献
Enhancing Scientific Research with FAIR Digital Objects in the National Science Data Fabric
利用国家科学数据结构中的 FAIR 数字对象加强科学研究
- DOI:
- 发表时间:20232023
- 期刊:
- 影响因子:0
- 作者:Michela Taufer;Heberth Martinez;Jakob Luettgau;Lauren Whitnah;G. Scorzelli;P. Newell;Aashish Panta;P. Bremer;Douglas Fils;Christine R. Kirkpatrick;V. Pascucci;Kathryn Mohror;J. ShalfMichela Taufer;Heberth Martinez;Jakob Luettgau;Lauren Whitnah;G. Scorzelli;P. Newell;Aashish Panta;P. Bremer;Douglas Fils;Christine R. Kirkpatrick;V. Pascucci;Kathryn Mohror;J. Shalf
- 通讯作者:J. ShalfJ. Shalf
Integrating FAIR Digital Objects (FDOs) into the National Science Data Fabric (NSDF) to Revolutionize Dataflows for Scientific Discovery
将 FAIR 数字对象 (FDO) 集成到国家科学数据结构 (NSDF) 中,彻底改变科学发现的数据流
- DOI:
- 发表时间:
- 期刊:
- 影响因子:0
- 作者:Michela Taufer;Heberth Martinez;Jakob Luettgau;Lauren Whitnah;†. GiorgioScorzelli;†. PaniaNewel;Aashish Panta;Timo Bremer;§. DougFils;¶. ChristineR.Kirkpatrick;Nina McCurdy;V. Pascucci;U. Knoxville;†. U.Utah;R. LLNL ‡;Research CenterMichela Taufer;Heberth Martinez;Jakob Luettgau;Lauren Whitnah;†. GiorgioScorzelli;†. PaniaNewel;Aashish Panta;Timo Bremer;§. DougFils;¶. ChristineR.Kirkpatrick;Nina McCurdy;V. Pascucci;U. Knoxville;†. U.Utah;R. LLNL ‡;Research Center
- 通讯作者:Research CenterResearch Center
共 2 条
- 1
Michela Taufer的其他基金
Collaborative Research: SHF: Small: Model-driven Design and Optimization of Dataflows for Scientific Applications
协作研究:SHF:小型:科学应用数据流的模型驱动设计和优化
- 批准号:23311522331152
- 财政年份:2023
- 资助金额:$ 22.5万$ 22.5万
- 项目类别:Standard GrantStandard Grant
SHF: Small: Methods, Workflows, and Data Commons for Reducing Training Costs in Neural Architecture Search on High-Performance Computing Platforms
SHF:小型:降低高性能计算平台上神经架构搜索训练成本的方法、工作流程和数据共享
- 批准号:22237042223704
- 财政年份:2022
- 资助金额:$ 22.5万$ 22.5万
- 项目类别:Standard GrantStandard Grant
Collaborative Research: Elements: SENSORY: Software Ecosystem for kNowledge diScOveRY - a data-driven framework for soil moisture applications
协作研究:要素:SENSORY:知识发现的软件生态系统 - 土壤湿度应用的数据驱动框架
- 批准号:21038452103845
- 财政年份:2021
- 资助金额:$ 22.5万$ 22.5万
- 项目类别:Standard GrantStandard Grant
Collaborative Research: PPoSS: Planning: Performance Scalability, Trust, and Reproducibility: A Community Roadmap to Robust Science in High-throughput Applications
协作研究:PPoSS:规划:性能可扩展性、信任和可重复性:高通量应用中稳健科学的社区路线图
- 批准号:20289232028923
- 财政年份:2020
- 资助金额:$ 22.5万$ 22.5万
- 项目类别:Standard GrantStandard Grant
Collaborative Research: EAGER: Advancing Reproducibility in Multi-Messenger Astrophysics
合作研究:EAGER:提高多信使天体物理学的可重复性
- 批准号:20419772041977
- 财政年份:2020
- 资助金额:$ 22.5万$ 22.5万
- 项目类别:Standard GrantStandard Grant
SHF: Medium: Collaborative Research: ANACIN-X: Analysis and modeling of Nondeterminism and Associated Costs in eXtreme scale applications
SHF:中:协作研究:ANACIN-X:极端规模应用中的非确定性和相关成本的分析和建模
- 批准号:19008881900888
- 财政年份:2019
- 资助金额:$ 22.5万$ 22.5万
- 项目类别:Continuing GrantContinuing Grant
Collaborative: EAGER: Exploring and Advancing the State of the Art in Robust Science in Gravitational Wave Physics
合作:EAGER:探索和推进引力波物理学稳健科学的最新技术
- 批准号:18413991841399
- 财政年份:2018
- 资助金额:$ 22.5万$ 22.5万
- 项目类别:Standard GrantStandard Grant
Collaborative: EAGER: Exploring and Advancing the State of the Art in Robust Science in Gravitational Wave Physics
合作:EAGER:探索和推进引力波物理学稳健科学的最新技术
- 批准号:18233721823372
- 财政年份:2018
- 资助金额:$ 22.5万$ 22.5万
- 项目类别:Standard GrantStandard Grant
SHF:Medium:Collaborative Research:A comprehensive methodology to pursue reproducible accuracy in ensemble scientific simulations on multi- and many-core platforms
SHF:中:协作研究:在多核和众核平台上追求集合科学模拟的可重复精度的综合方法
- 批准号:18415521841552
- 财政年份:2018
- 资助金额:$ 22.5万$ 22.5万
- 项目类别:Standard GrantStandard Grant
BIGDATA: IA: Collaborative Research: In Situ Data Analytics for Next Generation Molecular Dynamics Workflows
BIGDATA:IA:协作研究:下一代分子动力学工作流程的原位数据分析
- 批准号:18417581841758
- 财政年份:2018
- 资助金额:$ 22.5万$ 22.5万
- 项目类别:Standard GrantStandard Grant
相似国自然基金
面向大规模新能源消纳的综合能源系统分布式动态安全协同优化方法
- 批准号:52377107
- 批准年份:2023
- 资助金额:50 万元
- 项目类别:面上项目
粤港澳大湾区大型综合医院功能与节能协同下的布局设计方法研究
- 批准号:52378025
- 批准年份:2023
- 资助金额:50 万元
- 项目类别:面上项目
基于变分推断和物理约束的机器学习综合方法校准南极冰架流变参数
- 批准号:42376230
- 批准年份:2023
- 资助金额:51 万元
- 项目类别:面上项目
激励幅度受限的非常规阵列波束综合方法研究
- 批准号:62301414
- 批准年份:2023
- 资助金额:30 万元
- 项目类别:青年科学基金项目
基于点云扫描规划的机电综合管线工程三维重建方法与施工质量智能检测研究
- 批准号:52308311
- 批准年份:2023
- 资助金额:30 万元
- 项目类别:青年科学基金项目
相似海外基金
Conference: Advancing AI in Science Education (AASE): A Comprehensive Approach to Equity, Inclusion, and Three-Dimensional Learning
会议:推进科学教育中的人工智能 (AASE):公平、包容和三维学习的综合方法
- 批准号:23329642332964
- 财政年份:2024
- 资助金额:$ 22.5万$ 22.5万
- 项目类别:Standard GrantStandard Grant
Comprehensive and personalized assessment of acute coronary syndrome by multiomic approach and artificial intelligence strategy
利用多组学方法和人工智能策略对急性冠脉综合征进行全面、个性化的评估
- 批准号:EP/X040267/1EP/X040267/1
- 财政年份:2023
- 资助金额:$ 22.5万$ 22.5万
- 项目类别:Research GrantResearch Grant
Maternal Fetal Medicine Units Network: University of California, San Francisco
母胎医学单位网络:加州大学旧金山分校
- 批准号:1068287210682872
- 财政年份:2023
- 资助金额:$ 22.5万$ 22.5万
- 项目类别:
Cardiovascular risk from comprehensive evaluation of the CT calcium score exam
CT钙评分检查综合评估心血管风险
- 批准号:1085374210853742
- 财政年份:2023
- 资助金额:$ 22.5万$ 22.5万
- 项目类别: