面向自然口语对话的深层次信息感知与表达方法研究
项目介绍
AI项目解读
基本信息
- 批准号:61375027
- 项目类别:面上项目
- 资助金额:78.0万
- 负责人:
- 依托单位:
- 学科分类:F0605.模式识别与数据挖掘
- 结题年份:2017
- 批准年份:2013
- 项目状态:已结题
- 起止时间:2014-01-01 至2017-12-31
- 项目参与者:杨明浩; 温正棋; 王晓慧; 郑鑫; 王欣; 赵凯; 吴锡欣; 张冉; 李昊;
- 关键词:
项目摘要
While state-of-the-art spoken dialog system can understand the linguistic information of user's input speech, lots of subtle emotional and paralinguistic information, such as user's intension, attitude, affective states, etc, is largely neglected. Such information, named deep information related to communicative intentions in this project, plays a very important role in spoken language communication among humans in daily social interactions. People express themselves not only through audio channel (prosody and voice quality), but also through visual channel (expressions, head movements and even body gestures). Hence the deep information is expressed in audio and visual bimodalities. This project aims to develop methods for the perception and generation of deep information related to communicative intentions in both audio and visual modalities to provide more natural human computer spoken dialog interaction. This project intends: 1) To systematically analyze the correlations between deep information and the semantic meaning of current spoken dialog context, the audio and visual expressions from both sides of the two interactive speakers; 2) To propose a method for deep information perception (cognitive appraisal) such that the communicative intentions could be recognized from user's input by considering the information from current dialog context, audio and visual features; 3) To build a model for deep information prediction (response prediction) which predicts the communicative intentions of system's response based on the understanding of communicative intentions from user's input; 4) To establish an algorism for deep information expression (expression control) which generates appropriate audio and visual speech outputs according to the desired communicative intentions of system's response output; and 5) To propose a framework for deep information processing that integrates the above three aspects including cognitive appraisal, response prediction and expression control to form an all pass circuit for human computer spoken dialog interaction. It is expected that findings of this project will enrich the understanding of the relationship between dialog context and audio visual expressions in human-computer speech interaction. Findings can also extend its application in the field of natural human-computer interaction, visual reality, and intelligent agent for spoken dialog.
现有的口语对话系统在信息处理时,忽视了音视觉所传递的意图侧重、情感态度等反映对话意图的"深层次信息",缺乏对其进行感知与表达的能力,导致系统输出平淡乏味,难以满足自然口语对话的要求。本项目拟系统地分析人们的自然口语对话过程;研究分析深层次信息与对话情境、语音视觉表现间的关系;提出用户输入的认知评估算法,建立融合对话情境、音视觉特征的深层次信息感知模型;提出系统响应的预测算法,建立深层次信息响应预测模型;提出系统输出的表达控制算法,实现深层次信息的音视觉表达生成;从语音和视觉多通道构建面向自然口语对话的深层次信息感知与表达方法(含认知评估、响应预测、表达控制),实现具有对话意图理解与表达能力的自然口语对话系统。相关成果将加深对言语交互过程中对话情境与音视觉表达间关系的理解,为在人机交互中建立更有效的音视觉感知与生成提供必要的理论基础,并积累相应的关键技术。本研究具有广泛的应用前景。
结项摘要
现有口语对话系统在处理时忽视了音视觉所传递的反映沟通意图的“深层次信息”,缺乏对其进行感知与表达的能力,难以满足自然口语对话的要求。本项目旨在从对话焦点入手,系统分析自然口语对话过程中信息表达的含义,研究对话焦点约束下的对话意图理解、对话意图的多模态表达的理解与呈现模型,研究新型的人机对话方法。.围绕上述目标,本项目取得的主要研究进展和成果如下:在对话焦点检测方面,提出了多模态的口语对话焦点感知和预测方法,实现由用户输入检测是否存在焦点;在对话意图理解方面,提出了基于多任务深度学习的用户意图理解模型,并将词向量模型用于对话系统意图分类,基于文本语音等多模态信息准确理解说话人意图;在对话建模管理方面,建立了语音图像对话管理模型,进行多模态深度融合内容理解及面向用户教授意图的答案反馈;在具有沟通意图表达功能的可视语音合成方面,提出了面向对话交互的焦点重音生成方法,利用双向长短时记忆网络构建音视觉参数映射模型,实现符合焦点重音表达需求的虚拟说话人脸像头动生成;在系统原型研制方面,构建了基于自我对话机制的用户教授意图的聊天机器人,研发了口语对话演示系统,实现了文本焦点及语音重音的自动检测、文本视觉语音融合的意图理解、凸显焦点意图表达的语音重音生成及虚拟人生成。.在国内外重点学术刊物和会议上发表学术论文46篇,其中SCI检索4篇,EI检索34篇,期刊论文6篇,CCF A类顶级会议论文3篇;获教育部科技进步二等奖,会议最佳论文奖,全球极客大赛“AI仿声验声攻防赛”第一名;培养毕业博士4人,毕业硕士12人;申请国家发明专利1项;科技成果转化93万元人民币。.本项目研究加深了对言语交互过程中话语意图与音视觉表达关系的理解,为人机交互中多模态意图感知理解、凸显意图的可视语音生成积累了关键技术。随着人工智能发展,本项目成果可应用在智能语音助手、智能音箱、聊天机器人、虚拟现实中等,具有广泛应用前景。
项目成果
期刊论文数量(6)
专著数量(0)
科研奖励数量(6)
会议论文数量(40)
专利数量(1)
User behavior fusion in dialog management with multi-modal history cues
具有多模式历史线索的对话管理中的用户行为融合
- DOI:10.1007/s11042-014-2161-5
- 发表时间:2014-07
- 期刊:Multimedia Tools and Applications
- 影响因子:3.6
- 作者:Minghao Yang;Jianhua Tao;Linlin Chao;Hao Li;Dawei Zhang;Hao Che;Tingli Gao;Bin Liu
- 通讯作者:Bin Liu
语音合成中基于稳定段边界的不定长基元选取算法
- DOI:--
- 发表时间:2014
- 期刊:软件学报
- 影响因子:--
- 作者:王欣;吴志勇;蔡莲红
- 通讯作者:蔡莲红
Emotional head motion predicting from prosodic and linguistic features
根据韵律和语言特征预测情绪头部运动
- DOI:10.1007/s11042-016-3405-3
- 发表时间:2016
- 期刊:Multimedia Tools and Applications
- 影响因子:3.6
- 作者:Minghao Yang;Jinlin Jiang;Jianhua Tao;Kaihui Mu;Hao Li
- 通讯作者:Hao Li
Acoustic to articulatory mapping with deep neural network
使用深度神经网络进行声学到发音映射
- DOI:10.1007/s11042-014-2183-z
- 发表时间:2015-11
- 期刊:Multimedia Tools and Applications
- 影响因子:3.6
- 作者:Zhiyong Wu;Kai Zhao;Xixin Wu;Xinyu Lan;Helen Meng
- 通讯作者:Helen Meng
汉语重音的凸显度分析与合成
- DOI:--
- 发表时间:2015
- 期刊:声学学报
- 影响因子:--
- 作者:孟凡博;吴志勇;贾珈;蔡莲红
- 通讯作者:蔡莲红
数据更新时间:{{ journalArticles.updateTime }}
{{
item.title }}
{{ item.translation_title }}
- DOI:{{ item.doi || "--"}}
- 发表时间:{{ item.publish_year || "--" }}
- 期刊:{{ item.journal_name }}
- 影响因子:{{ item.factor || "--"}}
- 作者:{{ item.authors }}
- 通讯作者:{{ item.author }}
数据更新时间:{{ journalArticles.updateTime }}
{{ item.title }}
- 作者:{{ item.authors }}
数据更新时间:{{ monograph.updateTime }}
{{ item.title }}
- 作者:{{ item.authors }}
数据更新时间:{{ sciAawards.updateTime }}
{{ item.title }}
- 作者:{{ item.authors }}
数据更新时间:{{ conferencePapers.updateTime }}
{{ item.title }}
- 作者:{{ item.authors }}
数据更新时间:{{ patent.updateTime }}
其他文献
基于TIGGE数据的中期降水预报在江苏省的应用效果评估
- DOI:10.13476/j.cnki.nsbdqk.2017.06.003
- 发表时间:2017
- 期刊:南水北调与水利科技
- 影响因子:--
- 作者:杜雅玲;陆桂华;吴志勇;何海
- 通讯作者:何海
基于多重分形的降雨时空解集研究
- DOI:--
- 发表时间:--
- 期刊:四川大学学报(工程科学版)
- 影响因子:--
- 作者:周玉良;金菊良;吴志勇;陆桂华
- 通讯作者:陆桂华
遗传算法在多维Fuzzing技术中的应用
- DOI:--
- 发表时间:--
- 期刊:小型微型计算机系统
- 影响因子:--
- 作者:吴志勇;王红川;孙乐昌;陈韬;张旻
- 通讯作者:张旻
近50年来中国干旱化特征分析
- DOI:--
- 发表时间:2010
- 期刊:水利水电技术
- 影响因子:--
- 作者:陆桂华;闫桂霞;吴志勇;何海
- 通讯作者:何海
笼养蓝冠噪鹛行为节律及时间分配研究
- DOI:--
- 发表时间:2017
- 期刊:四川动物
- 影响因子:--
- 作者:刘涛;吴志勇;刘斌;张微微;石金泽
- 通讯作者:石金泽
其他文献
{{
item.title }}
{{ item.translation_title }}
- DOI:{{ item.doi || "--" }}
- 发表时间:{{ item.publish_year || "--"}}
- 期刊:{{ item.journal_name }}
- 影响因子:{{ item.factor || "--" }}
- 作者:{{ item.authors }}
- 通讯作者:{{ item.author }}
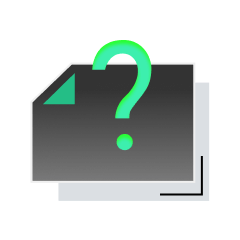
内容获取失败,请点击重试
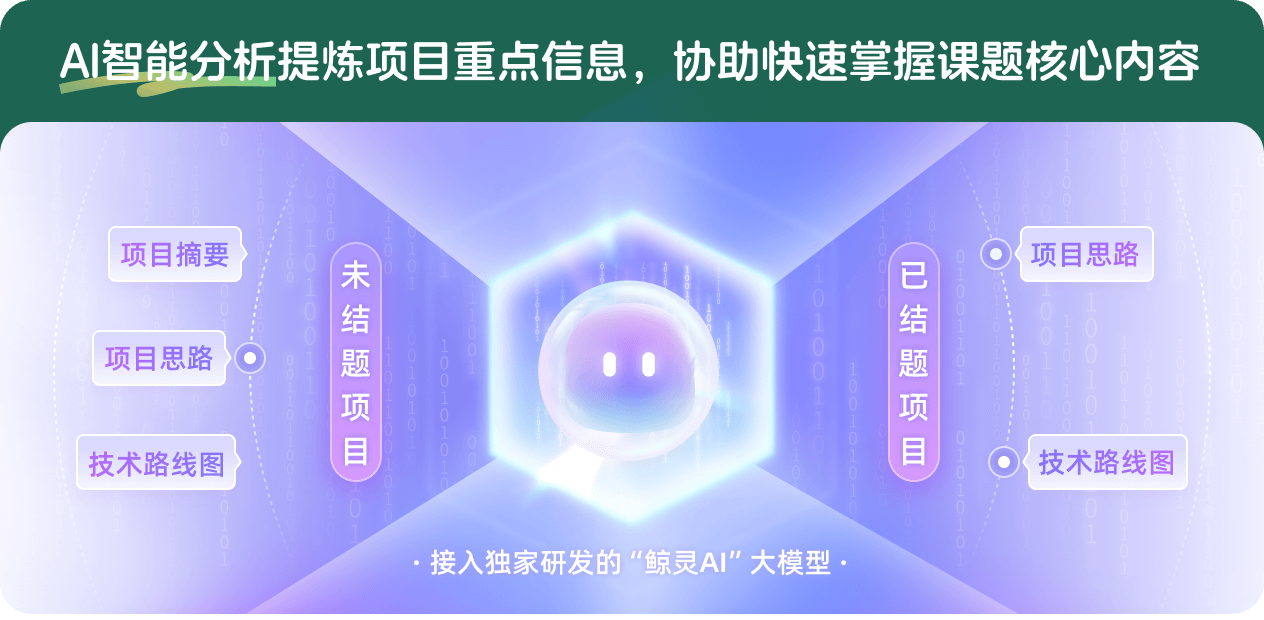
查看分析示例
此项目为已结题,我已根据课题信息分析并撰写以下内容,帮您拓宽课题思路:
AI项目摘要
AI项目思路
AI技术路线图
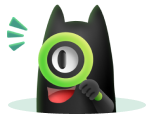
请为本次AI项目解读的内容对您的实用性打分
非常不实用
非常实用
1
2
3
4
5
6
7
8
9
10
您认为此功能如何分析更能满足您的需求,请填写您的反馈:
吴志勇的其他基金
面向智能语音交互的语音副语言信息解耦表征学习与可控语音生成研究
- 批准号:
- 批准年份:2020
- 资助金额:59 万元
- 项目类别:面上项目
音视融合的韵律模式的个性化研究
- 批准号:60805008
- 批准年份:2008
- 资助金额:20.0 万元
- 项目类别:青年科学基金项目
相似国自然基金
{{ item.name }}
- 批准号:{{ item.ratify_no }}
- 批准年份:{{ item.approval_year }}
- 资助金额:{{ item.support_num }}
- 项目类别:{{ item.project_type }}
相似海外基金
{{
item.name }}
{{ item.translate_name }}
- 批准号:{{ item.ratify_no }}
- 财政年份:{{ item.approval_year }}
- 资助金额:{{ item.support_num }}
- 项目类别:{{ item.project_type }}