时空大数据可视分析中信息混淆问题研究
项目介绍
AI项目解读
基本信息
- 批准号:61872066
- 项目类别:面上项目
- 资助金额:65.0万
- 负责人:
- 依托单位:
- 学科分类:F0209.计算机图形学与虚拟现实
- 结题年份:2022
- 批准年份:2018
- 项目状态:已结题
- 起止时间:2019-01-01 至2022-12-31
- 项目参与者:黄俊铭; 刘鹏飞; 赵志丹; 王庆; 陈玲姣; 高见; 韩梅; 魏骊睿; 张雨薇;
- 关键词:
项目摘要
Visual analytics has its unique advantages in analyzing and exhibiting the complex spatio-temporal big data: Presenting the information embedded within the data with visual encoding; providing abundant computer human interfaces to aid the analysts in effective analysis. One of the most significant issues in dealing with the huge amount of data is: How to choose the proper amount of information during analysis so that it won't cause confusion with too excessive or too little information displayed. Presenting too little information can result in the loss of valuable information, meanwhile presenting too much may lead to visual clutter. This project aims to systematically study the problem of information confusion in visual analysis of spatio-temporal big data based on semantic technology, information relation degree, and information potential theories. It mainly focuses on the three aspects of how to select the proper data for the limited display space and how to compute the information density in the context of large scale spatial and temporal data analytics: 1) The information confusion model for spatial and temporal big data visual analytics; 2) The visual representation algorithms of information confusion; 3) The evaluation of the information confusion models. This research is dedicated to exploring the quantification of information density, which can help to select the proper amount data for visualization, thus best improve the information granule while decrease the visual complexity with limited computing power. The goal of this research is to meet the users' requirements of identifiability, accuracy, and real time performance.
可视化在复杂时空大数据相关分析和表现方面具有独特优势,可以将数据中内蕴的属性信息进行视觉呈现,并借助丰富的人机交互手段辅助用户进行有效的分析。面对海量数据规模,一个很重要的问题就是如何在分析中展示合适的信息量,过多与过少都会造成信息混淆。显示的信息过少会导致信息丢失或上下文信息不足,显示的信息过多将导致视觉遮挡。本项目拟探索时空大数据可视分析中信息混淆问题,基于语义技术、信息关联度与信息势能理论,从时空大数据可视分析信息混淆模型、信息混淆可视化表征算法,与信息混淆模型性能评估三个方面,对海量数据规模下在有限的显示空间中如何进行数据筛选与计算信息密度展开研究。本项目探索了在时空大数据可视分析中如何量化信息密度,有助于判断分析中需要包含的数据,并选择显示中所需要的信息量,尽可能在计算力有限的情况下提高视觉呈现的信息粒度并降低视觉复杂度,满足用户对辨识能力、准确性和实时性的要求。
结项摘要
本研究旨在解决海量时空数据可视分析中信息混淆的问题,通过在有限的显示空间中对数据筛选与信息密度问题展开研究,针对数据规模大、维度高、多源异构等条件。我们主要基于用户认知的语义模型与交互反馈评估技术,对相关理论与方法展开了研究,研究内容包括以下三个方面:.首先,我们基于先验知识与语义技术进行复杂分析任务分解,对任务信息进行关联度建模,通过信息势能理论探索分析显示空间中的任务信息密度,总结并完成了信息混淆模型建模分析与研究。这一方面的研究成果,能够有效提高视觉呈现的信息粒度,并降低视觉复杂度。.其次,针对时空大数据空间、时间属性和高维多元特性,我们在上述基础上,设计了一种灵活的具备可扩展性的可视化表征算法,包含了完整地视觉编码算法、布局算法,和交互算法设计。这一方面的研究成果,通过提供流畅的交互,逐步精进分析结果,能够揭示数据中的隐藏特征,产生实际应用价值,并向分析决策者提供对普适性问题的验证。.最后,我们结合人机交互,构建了一种针对信息混淆模型性能评估的科学评价反馈机制。在这种机制下,评估结果将利用视觉通道与可视化手段将评估反馈结果全面转化为视觉图谱与知识库,同时结合模型参数设置与先验知识来提供相应的反馈。这一方面的研究成果,不仅能够提高分析结果的精确性和可靠性,还为应用和改进提供了重要的参考。.综上所述,本项目的研究成果不仅在理论和应用方面都具有重要的意义,而且能够应对大数据时代的分析需求,向决策者提供可信的数据分析结果,为实际应用提供了有力支撑。
项目成果
期刊论文数量(11)
专著数量(0)
科研奖励数量(0)
会议论文数量(6)
专利数量(7)
Dual Projective Zero-Shot Learning using Text Descriptions
使用文本描述的双投影零样本学习
- DOI:10.1145/3514247
- 发表时间:2022-07
- 期刊:ACM Transactions on Multimedia Computing, Communications, and Applications
- 影响因子:--
- 作者:Yunbo Rao;Ziqiang Yang;Xi Peng;Qifei Wang;Jiansu Pu
- 通讯作者:Jiansu Pu
A Symmetric Fully Convolutional Residual Network With DCRF for Accurate Tooth Segmentation
具有 DCRF 的对称全卷积残差网络,用于精确的牙齿分割
- DOI:10.1109/access.2020.2994592
- 发表时间:2020-01-01
- 期刊:IEEE ACCESS
- 影响因子:3.9
- 作者:Rao, Yunbo;Wang, Yilin;Wang, Qifei
- 通讯作者:Wang, Qifei
散点图信息混淆的可视分析与探究
- DOI:--
- 发表时间:--
- 期刊:计算机辅助设计与图形学学报
- 影响因子:--
- 作者:张景文;蒲剑苏;邵慧;程卓越;黄锦岳;丛迅超
- 通讯作者:丛迅超
A Projective Dual Approach for Generalized Zero-Shot Learning from Noisy Text Descriptions
从噪声文本描述中进行广义零样本学习的投影对偶方法
- DOI:--
- 发表时间:--
- 期刊:IEEE Transactions on Multimedia
- 影响因子:7.3
- 作者:Rao Yunbo;Yang Ziqiang;Wang Qifei;Pu Jiansu
- 通讯作者:Pu Jiansu
Extreme Feature Regions Detection and Accurate Quality Assessment for Point-Cloud 3D Reconstruction
点云 3D 重建的极端特征区域检测和精确质量评估
- DOI:10.1109/access.2019.2898731
- 发表时间:2019-01-01
- 期刊:IEEE ACCESS
- 影响因子:3.9
- 作者:Rao, Yunbo;Fan, Baijiang;Jin, Rize
- 通讯作者:Jin, Rize
数据更新时间:{{ journalArticles.updateTime }}
{{
item.title }}
{{ item.translation_title }}
- DOI:{{ item.doi || "--"}}
- 发表时间:{{ item.publish_year || "--" }}
- 期刊:{{ item.journal_name }}
- 影响因子:{{ item.factor || "--"}}
- 作者:{{ item.authors }}
- 通讯作者:{{ item.author }}
数据更新时间:{{ journalArticles.updateTime }}
{{ item.title }}
- 作者:{{ item.authors }}
数据更新时间:{{ monograph.updateTime }}
{{ item.title }}
- 作者:{{ item.authors }}
数据更新时间:{{ sciAawards.updateTime }}
{{ item.title }}
- 作者:{{ item.authors }}
数据更新时间:{{ conferencePapers.updateTime }}
{{ item.title }}
- 作者:{{ item.authors }}
数据更新时间:{{ patent.updateTime }}
其他文献
其他文献
{{
item.title }}
{{ item.translation_title }}
- DOI:{{ item.doi || "--" }}
- 发表时间:{{ item.publish_year || "--"}}
- 期刊:{{ item.journal_name }}
- 影响因子:{{ item.factor || "--" }}
- 作者:{{ item.authors }}
- 通讯作者:{{ item.author }}
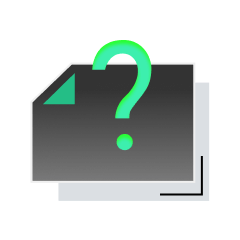
内容获取失败,请点击重试
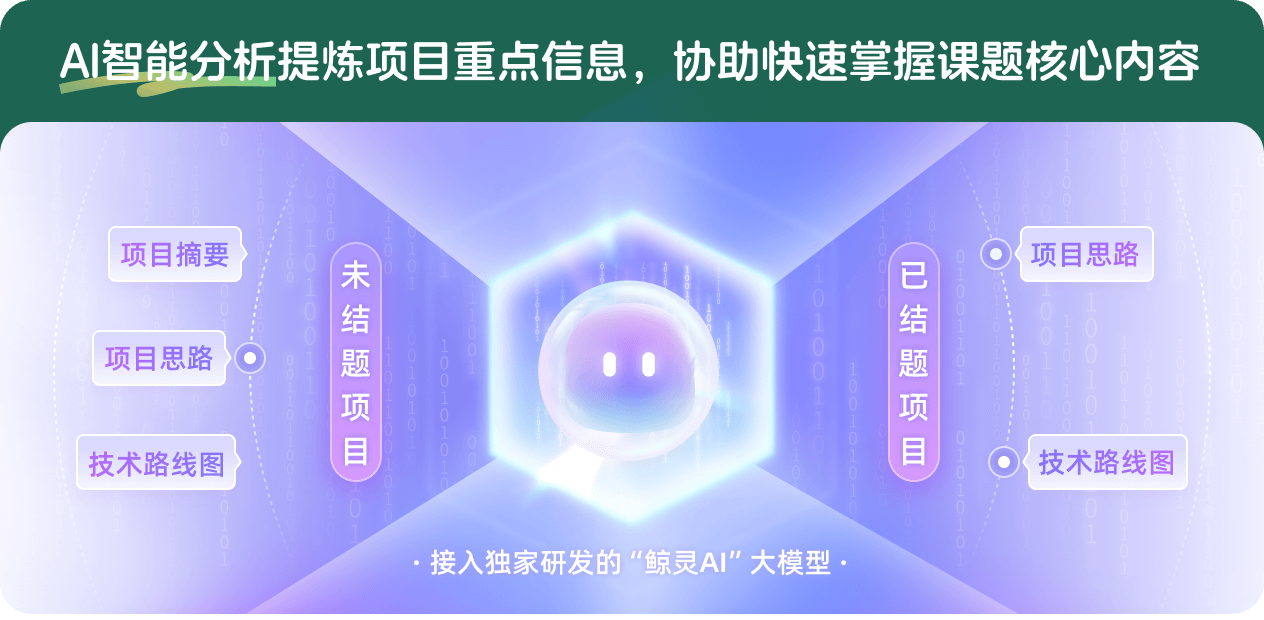
查看分析示例
此项目为已结题,我已根据课题信息分析并撰写以下内容,帮您拓宽课题思路:
AI项目摘要
AI项目思路
AI技术路线图
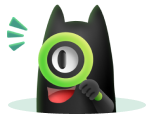
请为本次AI项目解读的内容对您的实用性打分
非常不实用
非常实用
1
2
3
4
5
6
7
8
9
10
您认为此功能如何分析更能满足您的需求,请填写您的反馈:
蒲剑苏的其他基金
基于可视分析的可解释小样本深度学习在固态电解质数据中的研究
- 批准号:
- 批准年份:2022
- 资助金额:55 万元
- 项目类别:面上项目
基于可视分析的可解释小样本深度学习在固态电解质数据中的研究
- 批准号:62272088
- 批准年份:2022
- 资助金额:55.00 万元
- 项目类别:面上项目
流模式下人类行为数据时空特性可视化分析研究
- 批准号:61502083
- 批准年份:2015
- 资助金额:20.0 万元
- 项目类别:青年科学基金项目
相似国自然基金
{{ item.name }}
- 批准号:{{ item.ratify_no }}
- 批准年份:{{ item.approval_year }}
- 资助金额:{{ item.support_num }}
- 项目类别:{{ item.project_type }}
相似海外基金
{{
item.name }}
{{ item.translate_name }}
- 批准号:{{ item.ratify_no }}
- 财政年份:{{ item.approval_year }}
- 资助金额:{{ item.support_num }}
- 项目类别:{{ item.project_type }}