流模式下人类行为数据时空特性可视化分析研究
项目介绍
AI项目解读
基本信息
- 批准号:61502083
- 项目类别:青年科学基金项目
- 资助金额:20.0万
- 负责人:
- 依托单位:
- 学科分类:F0214.新型计算及其应用基础
- 结题年份:2018
- 批准年份:2015
- 项目状态:已结题
- 起止时间:2016-01-01 至2018-12-31
- 项目参与者:刘金虎; 陈玲姣; 赵倩; 卢扬;
- 关键词:
项目摘要
Increasing amounts of large-scale data recording human behaviors is becoming available for researchers due to the advances in electronic technologies. Mining or analyzing these kinds of data can shed light into many important applications such as city management, road network planning, traffic control, and emergency response etc. However, exploring and analyzing this enormous amount of data become increasingly difficult for traditional data mining and machine learning methods. These complex data are usually noisy, sparse or incomplete, unstructured, high dimensional, and contain both spatial and temporal attributes and many other attributes varied by different application domains. Visualization can be of great value for human behavior data analysis as it can represent the data in intuitive ways with rich context over multiple dimensions, spatio-temporal attributes and also support explorative visual analysis that keeps humans in the loop. In this project, we propose to work on research for visual analysis on temporal, spatial, and multidimensional multivariate features for large-scale human behavior data under streaming data model. In order to explorative research on scalability of data visual analysis in different displays, in different applications, in different data sizes we plan to propose a new visual analytic model including visual encoding design and user interaction design. This model is designed to visual mining and reveal the hidden spatial-temporal patterns in these data can convey useful knowledge, which contributes to the decision-making and problem solving, and have high social and commercial values. This model aims to adopt a new visual encoding algorithm to better rendering the visual representations with more clear presentation under limited computational resources while reducing the complexity of visual cues with minimum information loss. And it will improve the interaction design with more flexible operations, allowing users to explore the data and improve mining processes and results with their domain knowledge.
随着电子技术的爆炸状发展,让大规模人类行为数据收集变得越来越容易。经过挖掘分析后,从数据中获取的知识在城市管理、道路规划、交通控制、应急处置等多个领域中具有十分广阔的应用前景。人类行为数据不仅包含空间和时间属性,而且由不同应用收集来的数据也具备多种其它属性,因此该数据量大维度高,纯粹使用数据挖掘和机器学习手段分析起来难度很大。可视化技术可以直观地呈现人类行为数据,并提供丰富的互动,以揭示数据中包含的规律。本项目主要对流模式下大规模人类行为数据的时间、空间与高维多元特性展开可视分析研究。项目组拟研究一种可视分析算法模型,包含视觉编码算法与交互算法设计,来探索解决数据可视分析在不同显示、不同任务与不同数据尺度下的面对的可拓展性问题,尽可能在计算力有限的情况下提高视觉呈现的信息并降低视觉复杂度,提供流畅的交互来迭代式地逐步精进分析挖掘,揭示数据中隐藏的特征,以数据为本提供对普适性问题的验证。
结项摘要
本项目针对流模式下大规模人类行为数据的时间、空间与高维多元特性,研究了一种可视分析算法模型,来探索解决数据可视分析在不同显示、不同任务与不同数据尺度下的面对的可拓展性问题,尽可能在计算力有限的情况下提高视觉呈现的信息并降低视觉复杂度,提供流畅的交互来迭代式地逐步精进分析挖掘,揭示数据中隐藏的特征。主要的研究内容是:(1)人类行为数据空间、时间与高维多元特性的特征提取与认知抽象研究;(2)人类行为数据空间、时间与高维多元特性的可视化呈现研究;(3)用户交互设计和评估反馈研究。主要研究成果是:(1)特征提取与认知抽象研究:本项目研究分析了人类行为数据的时间、空间与高维多元属性,并对三种特性进行问题刻画和任务概括分析,以准确对特性中存在的特征进行提取和抽象。同时利用了自动分析算法的计算力提取出特征并抽象化,减少用户的分析工作量,特别是可视化方面的视觉混淆和减轻人注意力方面的消耗,并能比较高效地将流模式下到来的数据实时从数值知识转换为易读的视觉线索。(2)可视化呈现研究:本项目在对人类行为数据的时间、空间与高维多元特性进行了特征提取与认知抽象后,研究了新的数据可视化技术来呈现此类新型信息。其中研究并提出了新的可视化编码算法来将抽象后的数据描述转换为信息可视化描述的数据形式,以完成分析任务。我们提出的可视分析模型在可视呈现方面具备比较好的可扩展性,特别是在数据规模变化的情况下。同时设计的模型结构灵活,能够根据不同数据规模,甚至不同应用选择数据抽象的自动分析方法和可视呈现方式中使用的视觉编码和布局算法,使得在计算能力有限的情况下对同种分析任务。(3)用户交互设计和评估反馈研究:基于内容(1)中对行为数据进行特征提取和抽象后获得的数据三个特性的可视化数据类型描述,同时以(2)中对可视分析任务的概括和操作类型抽象研究作为基础,本项目充分利用人的感知和认知优势研究并设计了相应的用户交互算法,用来评估反馈修正特征提取抽象,和可视化编码算法设计。通过设计好的交互操作,利用机器的计算力和专家的经验及领域知识,来减少数据中的不确定性。
项目成果
期刊论文数量(2)
专著数量(0)
科研奖励数量(1)
会议论文数量(11)
专利数量(0)
Sci-Fin: Visual Mining Spatial and Temporal Behavior Features from Social Media.
科幻小说:从社交媒体视觉挖掘时空行为特征
- DOI:10.3390/s16122194
- 发表时间:2016-12-20
- 期刊:Sensors (Basel, Switzerland)
- 影响因子:--
- 作者:Pu J;Teng Z;Gong R;Wen C;Xu Y
- 通讯作者:Xu Y
Extreme Feature Regions Detection and Accurate Quality Assessment for Point-Cloud 3D Reconstruction
点云 3D 重建的极端特征区域检测和精确质量评估
- DOI:10.1109/access.2019.2898731
- 发表时间:2019-01-01
- 期刊:IEEE ACCESS
- 影响因子:3.9
- 作者:Rao, Yunbo;Fan, Baijiang;Jin, Rize
- 通讯作者:Jin, Rize
数据更新时间:{{ journalArticles.updateTime }}
{{
item.title }}
{{ item.translation_title }}
- DOI:{{ item.doi || "--"}}
- 发表时间:{{ item.publish_year || "--" }}
- 期刊:{{ item.journal_name }}
- 影响因子:{{ item.factor || "--"}}
- 作者:{{ item.authors }}
- 通讯作者:{{ item.author }}
数据更新时间:{{ journalArticles.updateTime }}
{{ item.title }}
- 作者:{{ item.authors }}
数据更新时间:{{ monograph.updateTime }}
{{ item.title }}
- 作者:{{ item.authors }}
数据更新时间:{{ sciAawards.updateTime }}
{{ item.title }}
- 作者:{{ item.authors }}
数据更新时间:{{ conferencePapers.updateTime }}
{{ item.title }}
- 作者:{{ item.authors }}
数据更新时间:{{ patent.updateTime }}
其他文献
散点图信息混淆的可视分析与探究
- DOI:--
- 发表时间:--
- 期刊:计算机辅助设计与图形学学报
- 影响因子:--
- 作者:张景文;蒲剑苏;邵慧;程卓越;黄锦岳;丛迅超
- 通讯作者:丛迅超
基于可视化的固态电解质材料机器学习筛选与预测
- DOI:10.11871/jfdc.issn.2096-742x.2021.04.002
- 发表时间:2021
- 期刊:数据与计算发展前沿
- 影响因子:--
- 作者:蒲剑苏;朱正国;邵慧;高博洋;朱焱麟;闫宗楷;向勇
- 通讯作者:向勇
其他文献
{{
item.title }}
{{ item.translation_title }}
- DOI:{{ item.doi || "--" }}
- 发表时间:{{ item.publish_year || "--"}}
- 期刊:{{ item.journal_name }}
- 影响因子:{{ item.factor || "--" }}
- 作者:{{ item.authors }}
- 通讯作者:{{ item.author }}
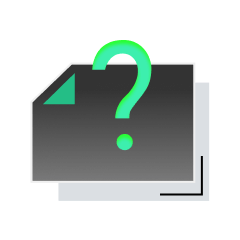
内容获取失败,请点击重试
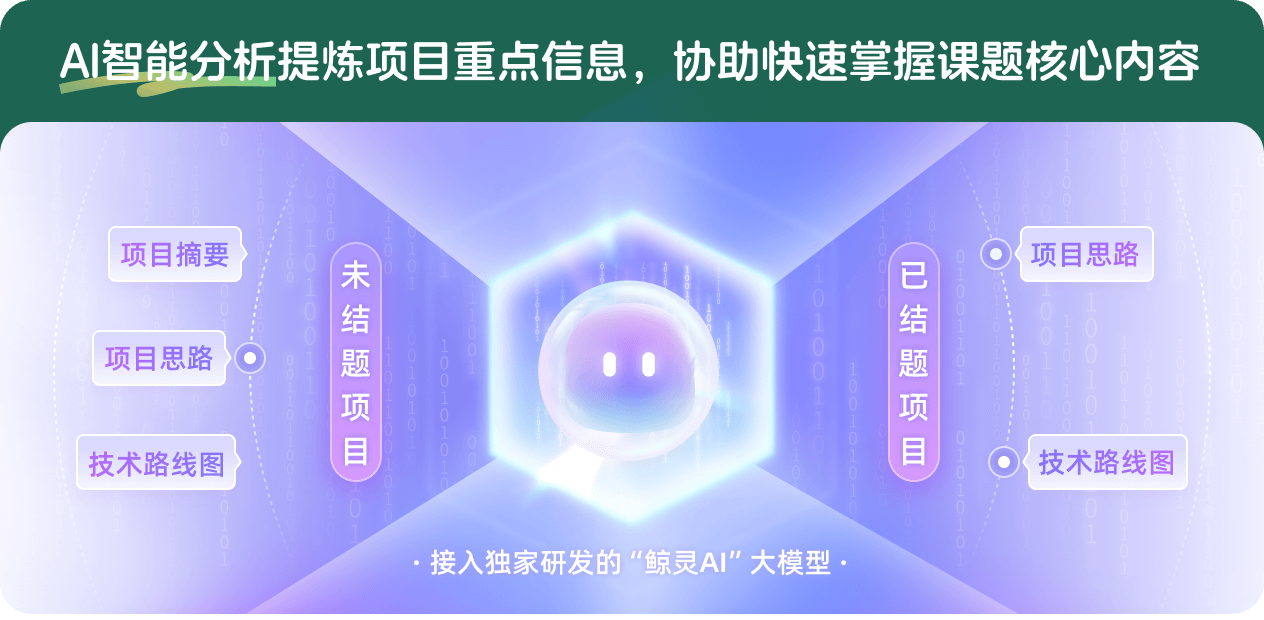
查看分析示例
此项目为已结题,我已根据课题信息分析并撰写以下内容,帮您拓宽课题思路:
AI项目摘要
AI项目思路
AI技术路线图
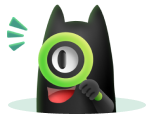
请为本次AI项目解读的内容对您的实用性打分
非常不实用
非常实用
1
2
3
4
5
6
7
8
9
10
您认为此功能如何分析更能满足您的需求,请填写您的反馈:
蒲剑苏的其他基金
基于可视分析的可解释小样本深度学习在固态电解质数据中的研究
- 批准号:
- 批准年份:2022
- 资助金额:55 万元
- 项目类别:面上项目
基于可视分析的可解释小样本深度学习在固态电解质数据中的研究
- 批准号:62272088
- 批准年份:2022
- 资助金额:55.00 万元
- 项目类别:面上项目
时空大数据可视分析中信息混淆问题研究
- 批准号:61872066
- 批准年份:2018
- 资助金额:65.0 万元
- 项目类别:面上项目
相似国自然基金
{{ item.name }}
- 批准号:{{ item.ratify_no }}
- 批准年份:{{ item.approval_year }}
- 资助金额:{{ item.support_num }}
- 项目类别:{{ item.project_type }}
相似海外基金
{{
item.name }}
{{ item.translate_name }}
- 批准号:{{ item.ratify_no }}
- 财政年份:{{ item.approval_year }}
- 资助金额:{{ item.support_num }}
- 项目类别:{{ item.project_type }}