基于GPU-CPU混合并行计算的微生物组大数据索引与搜索方法学研究
项目介绍
AI项目解读
基本信息
- 批准号:31771463
- 项目类别:面上项目
- 资助金额:55.0万
- 负责人:
- 依托单位:
- 学科分类:C0608.生物数据资源与分析方法
- 结题年份:2021
- 批准年份:2017
- 项目状态:已结题
- 起止时间:2018-01-01 至2021-12-31
- 项目参与者:黄适; 荆功超; 公衍海; 孙政; 何曰辉;
- 关键词:
项目摘要
Metagenomics is one of the most important and widely used approaches to study microbiome, which has enormous potential in solving crucial issues such as human health, environment protection and bio-energy. Although the volume of metagenome datasets has been increasing exponentially, the paucity of efficient methods for the “microbiome to microbiomes” type of search has greatly hindered the in-depth annotation of an unknown microbiota based on the vast amount of existing metagenomic data. The key technological bottlenecks here include the difficulty of reducing searching complexity among massive number of samples and the inability to comprehensively, rapidly yet precisely measure the structural or functional ‘matches’ between communities. To tackle these challenges, in this work we propose to establish a normalized indexing strategy based on microbial community features to improve the speed of search response, and then develop a multi-angle metagenome-to-metagenome alignment algorithm using the GPU-CPU hybrid parallel computing to enable the whole-microbiome oriented rapid search. Such a strategy should enable rapid and accurate identification of the microbiome in the database that is of the highest similarity in either community structure or function to the query microbiome, so that the associated metadata of the matching microbiome(s) can be used to annotate the query microbiome or to predict the environmental/host properties associated with the query microbiome. Based on this novel methodology, the first large-scale microbiome search engine that allows both ‘structure-based’ and ‘function-based’ search of metagenomes will be demonstrated using oral microbiome as a model, which will serve the research by enabling “gaining new knowledge by reviewing old” in the emerging area of Microbiome Data Science.
元基因组学是研究微生物组最重要和最普及的手段之一,在解决健康、环保、生物能源等重大问题上有巨大潜力。目前,元基因组学数据正在呈指数级增长,然而“群落对群落”式的微生物组搜索尚无高效解决方案,严重阻碍了基于海量已知数据对新样本的深入解读。此中的技术难点包括如何降低海量样本中的搜索复杂度以及如何全面、迅速、准确地衡量群落间的匹配性等。针对以上瓶颈,申请人提出建立微生物群落特征的归一化索引,以提高搜索响应,并开发基于GPU-CPU混合并行计算的多角度群落对比算法,能够在急速增长的已知数据中,迅速准确地找到与新样本在群落结构或功能上高度相似的匹配,从而根据后者的相关信息来注释新样本的特性并预测其所代表的环境特征。在此基础上,将以口腔微生物组为模式,示范首个“结构”和“功能”搜索兼备的大规模微生物组数据引擎与共性计算分析工具,服务于微生物组的“温故而知新”。
结项摘要
元基因组学是研究微生物组最重要和最普及的手段之一,在解决健康、环保、生物能源等重大问题上有巨大潜力。目前,元基因组学数据正在呈指数级增长,然而“群落对群落”式的微生物组搜索尚无高效解决方案,严重阻碍了基于海量已知数据对新样本的深入解读。此中的技术难点包括如何降低海量样本中的搜索复杂度以及如何全面、迅速、准确地衡量群落间的匹配性等。针对以上瓶颈,本研究开发了微生物组搜索引擎,通过建立微生物群落特征的归一化索引,以提高搜索响应,并开发基于并行计算的多角度群落对比算法,在急速增长的已知数据中,迅速准确地找到与新样本在群落结构或功能上高度相似的匹配,从而根据后者的相关信息来注释新样本的特性并预测其所代表的环境特征。在此基础上,将以人体微生物组为模式,示范首个“结构”和“功能”搜索兼备的大规模微生物组数据引擎与共性计算分析工具,服务于微生物组的“温故而知新”。
项目成果
期刊论文数量(16)
专著数量(0)
科研奖励数量(0)
会议论文数量(0)
专利数量(0)
Method development for cross-study microbiome data mining: Challenges and opportunities
交叉研究微生物组数据挖掘的方法开发:挑战和机遇
- DOI:10.1016/j.csbj.2020.07.020
- 发表时间:2020
- 期刊:Computational and Structural Biotechnology Journal
- 影响因子:6
- 作者:Xiaoquan Su;Gongchao Jing;Yufeng Zhang;Shunyao Wu
- 通讯作者:Shunyao Wu
Microbiome Search Engine 2: a Platform for Taxonomic and Functional Search of Global Microbiomes on the Whole-Microbiome Level
微生物组搜索引擎2:在全微生物组水平上对全球微生物组进行分类和功能搜索的平台
- DOI:10.1128/msystems.00943-20
- 发表时间:2021-01-19
- 期刊:mSystems
- 影响因子:6.4
- 作者:Jing G;Liu L;Wang Z;Zhang Y;Qian L;Gao C;Zhang M;Li M;Zhang Z;Liu X;Xu J;Su X
- 通讯作者:Su X
Reply to Sun et al., "Identifying composition novelty in microbiome studies: Improvement of prediction accuracy"
回复 Sun 等人,“识别微生物组研究中的新颖性成分:提高预测准确性”
- DOI:10.1128/mbio.01234-19
- 发表时间:2019
- 期刊:mBio
- 影响因子:6.4
- 作者:Xiaoquan Su;Gongchao Jing;Daniel McDonald;Honglei Wang;Zengbin Wang;Antonio Gonzalez;Zheng Sun;Shi Huang;Jose Navas;Rob Knight;Jian Xu
- 通讯作者:Jian Xu
Multiple-Disease Detection and Classification across Cohorts via Microbiome Search
通过微生物组搜索进行跨队列的多种疾病检测和分类
- DOI:10.1128/msystems.00150-20
- 发表时间:2020
- 期刊:mSystems
- 影响因子:6.4
- 作者:Xiaoquan Su;Gongchao Jing;Zheng Sun;Lu Liu;Zhenjiang Xu;Daniel McDonald;Zengbin Wang;Honglei Wang;Antonio Gonzalez;Yufeng Zhang;Shi Huang;Gavin Huttley;Rob Knight;Jian Xu
- 通讯作者:Jian Xu
Dynamic Meta-Storms enables comprehensive taxonomic and phylogenetic comparison of shotgun metagenomes at the species level
动态元风暴能够在物种水平上对鸟枪法宏基因组进行全面的分类学和系统发育比较
- DOI:10.1093/bioinformatics/btz910
- 发表时间:2020
- 期刊:Bioinformatics
- 影响因子:5.8
- 作者:Gongchao Jing;Yufeng Zhang;Ming Yang;Lu Liu;Jian Xu;Xiaoquan Su
- 通讯作者:Xiaoquan Su
数据更新时间:{{ journalArticles.updateTime }}
{{
item.title }}
{{ item.translation_title }}
- DOI:{{ item.doi || "--"}}
- 发表时间:{{ item.publish_year || "--" }}
- 期刊:{{ item.journal_name }}
- 影响因子:{{ item.factor || "--"}}
- 作者:{{ item.authors }}
- 通讯作者:{{ item.author }}
数据更新时间:{{ journalArticles.updateTime }}
{{ item.title }}
- 作者:{{ item.authors }}
数据更新时间:{{ monograph.updateTime }}
{{ item.title }}
- 作者:{{ item.authors }}
数据更新时间:{{ sciAawards.updateTime }}
{{ item.title }}
- 作者:{{ item.authors }}
数据更新时间:{{ conferencePapers.updateTime }}
{{ item.title }}
- 作者:{{ item.authors }}
数据更新时间:{{ patent.updateTime }}
其他文献
元基因组学及其在转化医学中的应用
- DOI:--
- 发表时间:2015
- 期刊:遗传
- 影响因子:--
- 作者:孙政;王晓君;苏晓泉;宁康
- 通讯作者:宁康
元基因组学及其在转化医学中的应用
- DOI:--
- 发表时间:2015
- 期刊:遗传
- 影响因子:--
- 作者:孙政;王晓君;苏晓泉;宁康
- 通讯作者:宁康
其他文献
{{
item.title }}
{{ item.translation_title }}
- DOI:{{ item.doi || "--" }}
- 发表时间:{{ item.publish_year || "--"}}
- 期刊:{{ item.journal_name }}
- 影响因子:{{ item.factor || "--" }}
- 作者:{{ item.authors }}
- 通讯作者:{{ item.author }}
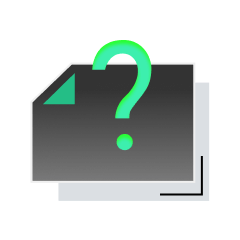
内容获取失败,请点击重试
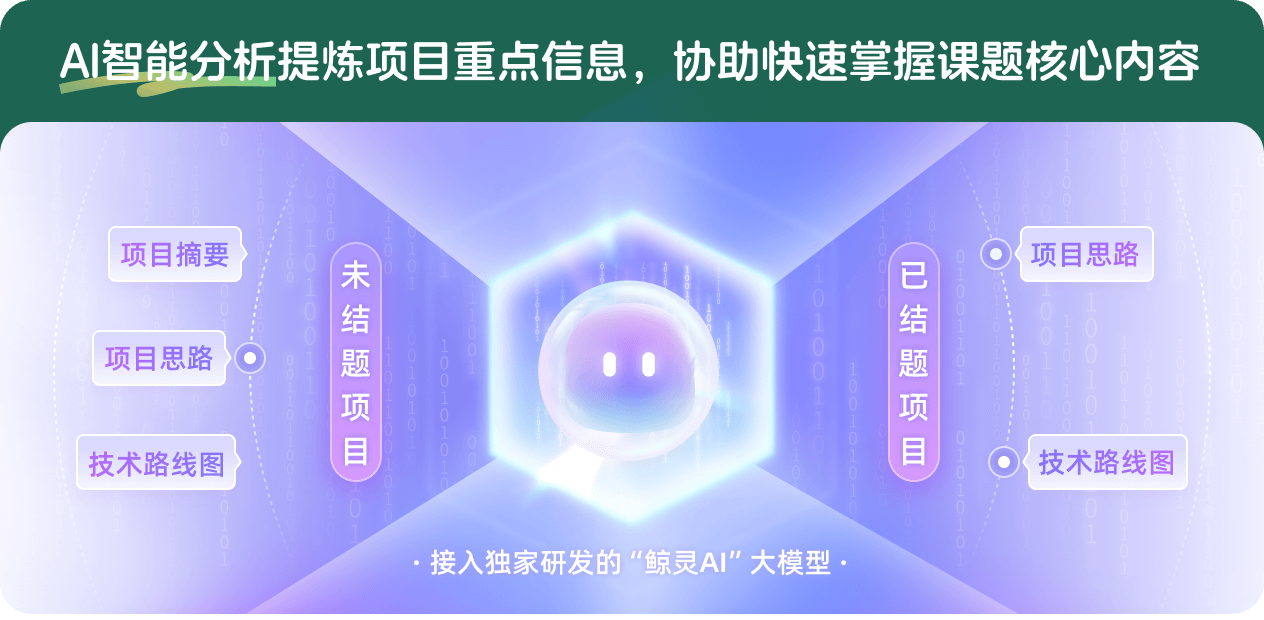
查看分析示例
此项目为已结题,我已根据课题信息分析并撰写以下内容,帮您拓宽课题思路:
AI项目摘要
AI项目思路
AI技术路线图
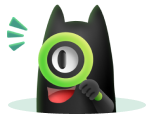
请为本次AI项目解读的内容对您的实用性打分
非常不实用
非常实用
1
2
3
4
5
6
7
8
9
10
您认为此功能如何分析更能满足您的需求,请填写您的反馈:
苏晓泉的其他基金
基于元基因组相似度计算的海量微生物群落数据挖掘
- 批准号:61303161
- 批准年份:2013
- 资助金额:23.0 万元
- 项目类别:青年科学基金项目
相似国自然基金
{{ item.name }}
- 批准号:{{ item.ratify_no }}
- 批准年份:{{ item.approval_year }}
- 资助金额:{{ item.support_num }}
- 项目类别:{{ item.project_type }}
相似海外基金
{{
item.name }}
{{ item.translate_name }}
- 批准号:{{ item.ratify_no }}
- 财政年份:{{ item.approval_year }}
- 资助金额:{{ item.support_num }}
- 项目类别:{{ item.project_type }}