新疆地区人群碘摄入量及组蛋白翻译后修饰在桥本甲状腺炎发病中的作用
项目介绍
AI项目解读
基本信息
- 批准号:81560136
- 项目类别:地区科学基金项目
- 资助金额:37.0万
- 负责人:
- 依托单位:
- 学科分类:H0701.内分泌系统免疫相关疾病
- 结题年份:2019
- 批准年份:2015
- 项目状态:已结题
- 起止时间:2016-01-01 至2019-12-31
- 项目参与者:王新玲; 罗蕴之; 王惠丽; 陈园; 韩莉; 辛亮; 李素丽; 徐子奇; 张竞;
- 关键词:
项目摘要
Hashimoto’s thyroiditis(HT) is a type of Autoimmune thyroid diseases(ATD) and is a main reason of primary hypothyroidism. Significant progress has been made in our understanding of the mechanisms leading to ATD, which epigenetics is a molecular link between genes and enviroment fantros.Our studies have found that there were interaction among DNA methylation modifications of susceptibility genes promoter by MeDIP-Seq technology and the proportion of Th1,Th2,Th17,Treg in vitro by Flow cytometry and median urinary iodine in ATD cases and controls. So our hypothesis is that epigenetic changes of ATD susceptibility genes in response to environmental stimulus of iodine intake would contribute to development of ATD in the individual with genetic susceptibility by autoimmune respones unbalance and genes expression abnormality. Based on the above study found, the project will further analyze histone post-translational modifications of ATD susceptibility genes of peripheral blood leukocytes in HT cases and controls by proteomic technologies, and find the genomic regions affected by the difference of histone post-translational modifications between case and control through ChIP-Seq technology targeting specific histone modifications. Molecular biological functional verification tests of important genes of important regions found and their affects on the proportion of Th1、Th2、Th17、Treg cell subsets, will be studied. This perspective will provide some insights into whether iodine intake influnce epigenetic modifications, including DNA methylaiton and histone post-translational modifications, resulting in increased disease susceptibility of individual for first time, which would find epigenetic marker of ATD development and help prevention early and scientific iodine intake of susceptibility individuals .
桥本甲状腺炎是自身免疫性甲状腺病(ATD)的一个类型,是原发性甲减最主要的原因。研究发现环境与遗传因素互作的表观遗传学改变可能是ATD重要病因。本课题组发现ATD病例对照组间碘营养状态、Th1/Th2/Th17/Treg细胞比例、CD40、IL2RA等易感基因的启动子区DNA甲基化频率有差异。故假设碘摄入量通过表观遗传途径影响ATD易感基因的表达,影响免疫系统的功能及相关基因表达异常,启动自身免疫反应,促使有遗传背景的个体发病。拟采用蛋白组学技术分析病例对照组间的组蛋白修饰差异,应用ChIP-Seq技术分析病例对照组间组蛋白差异所影响的基因组区域,及其重要基因对外周血Th1、Th2、Th17、Treg细胞亚群比例的影响。研究结果将首次阐述碘摄入量是否通过影响表观遗传修饰改变了疾病的易感性,为寻找ATD发病的表观遗传标志、高危人群的早期防治及科学合理补碘做出努力。
结项摘要
自身免疫性甲状腺疾病(AITD)主要包括Graves 病(GD)和桥本甲状腺炎(HT),分别是甲亢和甲减的常见原因。课题组的流行病学数据发现,碘缺乏组临床甲亢和甲减的患病率要明显高于碘足量组,但经历缺碘-补碘过程后,TPOAb阳性率增多,提示碘营养在疾病发生发展中有重要作用。转录组及组蛋白修饰研究发现:HT的甲状腺组织中,表达差异基因主要富集在细胞因子受体互相、原发性免疫缺陷、金黄色葡萄球菌感染等通路中,包括Th1、Th2、Th17细胞分化相关基因CD3G、CD3D、CD247、LCK、JAK3、ZAP70和IL2RG等的差异表达。H3K4me3和H3K9me3的组蛋白修饰在病例组有去甲基化性,差异在甲状腺激素信号通路、胰岛素信号通路、细菌感染。结合流式细胞分析数据发现,HT组的外周血样中Th1功能升高、细胞因子IFN表达升高,TRAb阳性的甲亢组则Th2为主、细胞因子IL10表达水平升高,Th1、Th2、Th17比例在不同临床表型间有显著差异。提示调节辅助T细胞Th1、Th2、Th17分化的基因参与疾病的发生过程,并可能在AITD的两种不同临床表型甲亢和甲减的动态平衡中发挥了积极作用。Th1/Th2的不平衡和Th17比例是疾病发生或加重的根源,转录数据显示,病例组CD3G、CD3D、CD247、LCK、JAK3、ZAP70和IL2RG等RNA的高表达参与调节了Th1/Th2/Th17的分化。后续,课题组拟探讨病例与正常对照者外周血中上述基因的转录水平与调T细胞的关系;同时拟通过Th1/Th2/Th17细胞试验,观察上述基因分别对调T细胞增值、分化及调控的影响。
项目成果
期刊论文数量(4)
专著数量(0)
科研奖励数量(0)
会议论文数量(0)
专利数量(0)
乌鲁木齐社区育龄期女性血清促甲状腺激素参考范围的建立
- DOI:--
- 发表时间:2019
- 期刊:中国全科医学
- 影响因子:--
- 作者:阿地拉·阿里木;郭艳英;王新玲;罗蕴之;张洁;吉米兰木·麦麦提明;李素丽
- 通讯作者:李素丽
新疆维吾尔自治区乌鲁木齐市维吾尔族和汉族育龄期妇女碘营养状况调查分析
- DOI:--
- 发表时间:2017
- 期刊:中华妇幼临床医学杂志(电子版)
- 影响因子:--
- 作者:郭艳英;阿地拉·阿里木;辛亮;李素丽;赵红丽;热孜万古丽·乌斯曼;王新玲
- 通讯作者:王新玲
社区人群促甲状腺素水平与血脂的相关性分析
- DOI:--
- 发表时间:2019
- 期刊:标记免疫分析与临床
- 影响因子:--
- 作者:邢淑清;赵建雯;王新玲;哈尼克孜·阿布都艾尼;努荣古丽·买买提;郭艳英
- 通讯作者:郭艳英
乌鲁木齐市某医院体检人群甲状腺功能检测结果
- DOI:--
- 发表时间:2017
- 期刊:热带医学杂志
- 影响因子:--
- 作者:邢淑清;郭艳英;田永芳;王新玲
- 通讯作者:王新玲
数据更新时间:{{ journalArticles.updateTime }}
{{
item.title }}
{{ item.translation_title }}
- DOI:{{ item.doi || "--"}}
- 发表时间:{{ item.publish_year || "--" }}
- 期刊:{{ item.journal_name }}
- 影响因子:{{ item.factor || "--"}}
- 作者:{{ item.authors }}
- 通讯作者:{{ item.author }}
数据更新时间:{{ journalArticles.updateTime }}
{{ item.title }}
- 作者:{{ item.authors }}
数据更新时间:{{ monograph.updateTime }}
{{ item.title }}
- 作者:{{ item.authors }}
数据更新时间:{{ sciAawards.updateTime }}
{{ item.title }}
- 作者:{{ item.authors }}
数据更新时间:{{ conferencePapers.updateTime }}
{{ item.title }}
- 作者:{{ item.authors }}
数据更新时间:{{ patent.updateTime }}
其他文献
乌鲁木齐地区正常人群血清TSH参考区间的建立
- DOI:--
- 发表时间:2015
- 期刊:标记免疫分析与临床
- 影响因子:--
- 作者:郭艳英;艾合买提江·吐呼提;热孜万古丽·乌斯曼;赵红丽
- 通讯作者:赵红丽
围绝经期和绝经后女性甲状腺疾病现况及特征研究
- DOI:--
- 发表时间:2021
- 期刊:中国全科医学
- 影响因子:--
- 作者:加孜热亚·再依拿提;邢淑清;热那姑丽·艾克拜尔;张凯迪;张玉媛;邢蓓;王新玲;郭艳英
- 通讯作者:郭艳英
Tg 基因 rs180195 位点多态性与新疆自身免疫性甲状腺疾病的相关性研究
- DOI:--
- 发表时间:2020
- 期刊:新疆医学
- 影响因子:--
- 作者:热那姑丽·艾克拜尔;王新玲;迪丽达尔·依马木;古再丽努尔·居来提;杜丹阳;董玲玲;郭艳英
- 通讯作者:郭艳英
CTLA4基因rs231775位点多态性与新疆维吾尔族人群自身免疫性甲状腺疾病的相关性
- DOI:--
- 发表时间:2020
- 期刊:中华地方病学杂志
- 影响因子:--
- 作者:热那姑丽·艾克拜尔;王新玲;李素丽;吉米兰木·买买提明;张洁;张凯迪;阿迪拉·阿里木;郭艳英
- 通讯作者:郭艳英
TPO、TG基因多个单核苷酸多态位点与新疆桥本甲状腺炎的病例对照研究
- DOI:--
- 发表时间:2020
- 期刊:中华内分泌代谢杂志
- 影响因子:--
- 作者:热那姑丽·艾克拜尔;王新玲;李素丽;古再丽努尔·居来提;邢蓓;迪丽达尔·依马木;穆叶沙尔·吾拉木;郭艳英
- 通讯作者:郭艳英
其他文献
{{
item.title }}
{{ item.translation_title }}
- DOI:{{ item.doi || "--" }}
- 发表时间:{{ item.publish_year || "--"}}
- 期刊:{{ item.journal_name }}
- 影响因子:{{ item.factor || "--" }}
- 作者:{{ item.authors }}
- 通讯作者:{{ item.author }}
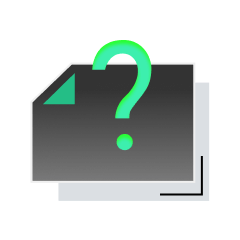
内容获取失败,请点击重试
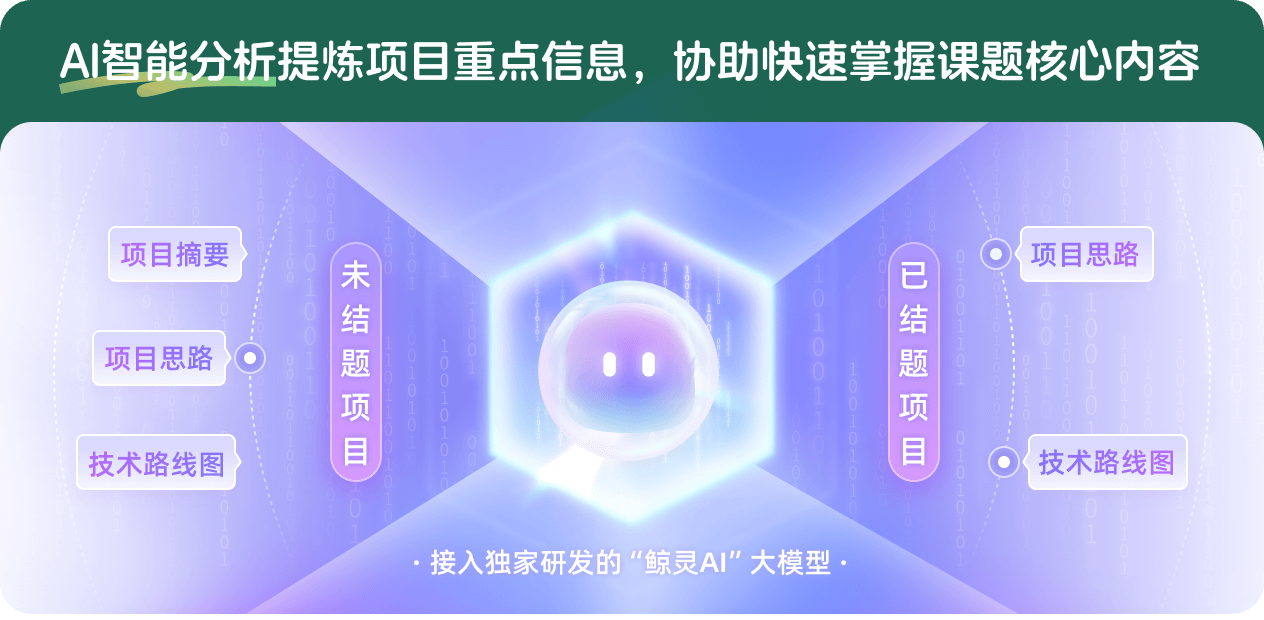
查看分析示例
此项目为已结题,我已根据课题信息分析并撰写以下内容,帮您拓宽课题思路:
AI项目摘要
AI项目思路
AI技术路线图
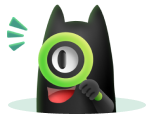
请为本次AI项目解读的内容对您的实用性打分
非常不实用
非常实用
1
2
3
4
5
6
7
8
9
10
您认为此功能如何分析更能满足您的需求,请填写您的反馈:
郭艳英的其他基金
自身免疫性甲状腺疾病遗传变异在介导AKT/mTOR信号通路及其与碘营养互作对甲状腺细胞生物学功能的研究
- 批准号:81860148
- 批准年份:2018
- 资助金额:35.0 万元
- 项目类别:地区科学基金项目
自身免疫性甲状腺疾病易感基因DNA甲基化与疾病发生机制的关系
- 批准号:81260127
- 批准年份:2012
- 资助金额:49.0 万元
- 项目类别:地区科学基金项目
相似国自然基金
{{ item.name }}
- 批准号:{{ item.ratify_no }}
- 批准年份:{{ item.approval_year }}
- 资助金额:{{ item.support_num }}
- 项目类别:{{ item.project_type }}
相似海外基金
{{
item.name }}
{{ item.translate_name }}
- 批准号:{{ item.ratify_no }}
- 财政年份:{{ item.approval_year }}
- 资助金额:{{ item.support_num }}
- 项目类别:{{ item.project_type }}