面向多目标群智感知的任务分配算法研究
项目介绍
AI项目解读
基本信息
- 批准号:61872149
- 项目类别:面上项目
- 资助金额:64.0万
- 负责人:
- 依托单位:
- 学科分类:F0208.物联网及其他新型网络
- 结题年份:2022
- 批准年份:2018
- 项目状态:已结题
- 起止时间:2019-01-01 至2022-12-31
- 项目参与者:邵硕; 徐培明; 杨淑美; 江乐; 吴旭东; 白文杰;
- 关键词:
项目摘要
Crowdsensing makes use of a great number of participants equipped with mobile sensing devices to implement various large-scale sensing applications that penetrate into people’s life. As the core algorithms of crowdsensing, sensing task assignment algorithms have become one of the research hotspots in the research community. Most of the existing research works on crowdsensing focus on optimizing sensing tasks from the same category. These works construct single objective models, based on which task assignment and data collection methods are designed. They have not incorporated the spatiotemporal relation features of different kinds of tasks, and thus cannot conduct collaborative optimization of tasks belonging to multiple categories. To overcome this problem, this proposal aims to investigate multi-objective task optimization models for crowdsensing with different kinds of tasks. By analyzing the factors, such as uncertainty of participant trajectories and dynamic features of participants, that influence the performance of algorithms for multi-objective models of sensing tasks, we will propose assignment approaches based on trajectory estimation, coverage estimation, and dynamic interaction models. The proposed assignment algorithms can make full use of the multi-dimensional sensing capabilities of participants, and thus improve the efficiency of task assignment algorithms for crowdsensing. Finally, we will verify the performance of the designed task assignment algorithms on the sensing applications in crowdsensing-based intelligent transportation systems. The results of this project will improve the overall performance of task assignment for crowdsensing and promote the real-world applications of crowdsensing systems.
群智感知利用携带移动感知设备的大量参与者来实现大规模的、渗透到人们生活方方面面的各类感知应用。作为群智感知的核心算法,感知任务分配算法已成为该研究领域的研究热点。目前大部分群智感知研究专注于单一类型的感知任务,构建单目标优化模型并进行任务分配和数据收集利用,未能融合不同类型任务之间的时空关联特性并进行多类型任务的协同优化。针对该问题,本项目研究面向多类型任务的多目标群智感知优化模型,针对多目标感知任务模型中参与者轨迹不确定性和动态特性等影响算法性能的因素,分别提出基于轨迹估计、覆盖估计、以及动态交互的分配策略,充分利用感知参与者的多维度感知能力,提高群智感知任务分配算法的效率。最后在基于群智感知的智能交通感知应用中对以上新型任务分配算法进行应用检验。本项目研究成果将提高群智感知任务分配的整体性能,促进其在实际系统中的应用普及。
结项摘要
感知任务分配是群智感知的一个核心问题,目前大部分分配算法未能融合不同类型任务之间的时空关联特性并进行多类型任务的协同优化。针对该问题,本项目研究多目标群智感知的任务优化模型,基于用户轨迹、时空覆盖、动态交互等任务分配中的关键元素,系统地研究了群智感知任务分配算法理论、机制、方法、以及系统。具体的,针对主动式感知和机会式感知场景的特征,研究了一系列基于用户轨迹规划、用户轨迹模糊估计、用户感知时长估计、位置熟悉度估计等模型的高效分配算法。针对感知任务不同粒度的覆盖需求,研究了基于跨区域动态预算、低预算任务聚类、双边隐私保护等技术的用户激励和任务匹配算法。针对感知系统用户动态交互的挑战,研究了基于空间多样性、任务完成质量、平台效用优化、感知用户异构等元素的群智感知激励与分配算法。项目相关成果已发表高水平国际期刊和会议论文30篇,包括IEEE/ACM Transactions系列论文13篇,授权发明专利6项。这些成果提高了群智感知任务分配和执行的整体效率,促进了群智感知系统的应用普及。
项目成果
期刊论文数量(24)
专著数量(0)
科研奖励数量(0)
会议论文数量(6)
专利数量(10)
Promoting Users' Participation in Mobile Crowdsourcing: A Distributed Truthful Incentive Mechanism (DTIM) Approach
促进用户参与移动众包:分布式真实激励机制(DTIM)方法
- DOI:10.1109/tvt.2020.2982243
- 发表时间:2020
- 期刊:IEEE Transactions on Vehicular Technology
- 影响因子:6.8
- 作者:Xiumin Wang;Wayes Tushar;Chau Yuen;Xinglin Zhang
- 通讯作者:Xinglin Zhang
PriDPM: Privacy-preserving dynamic pricing mechanism for robust crowdsensing
PriDPM:用于强大群智感知的隐私保护动态定价机制
- DOI:10.1016/j.comnet.2020.107582
- 发表时间:2020
- 期刊:Computer Networks
- 影响因子:5.6
- 作者:Liu Yuxian;Liu Fagui;Wu Hao-Tian;Zhang Xinglin;Zhao Bowen;Yan Xingfu
- 通讯作者:Yan Xingfu
Adaptive Label Propagation for Facial Appearance Transfer
用于面部外观迁移的自适应标签传播
- DOI:10.1109/tmm.2019.2918717
- 发表时间:2019-05
- 期刊:IEEE Transactions on Multimedia
- 影响因子:7.3
- 作者:Lingyu Liang;Xinglin Zhang
- 通讯作者:Xinglin Zhang
Computation Offloading for Partitionable Applications in Dense Networks: An Evolutionary Game Approach
密集网络中可分区应用程序的计算卸载:一种进化博弈方法
- DOI:10.1109/jiot.2022.3175729
- 发表时间:2022-11
- 期刊:IEEE Internet of Things Journal
- 影响因子:10.6
- 作者:Wenjian Lu;Xinglin Zhang
- 通讯作者:Xinglin Zhang
Duration-Sensitive Task Allocation for Mobile Crowd Sensing
用于移动人群感知的持续时间敏感的任务分配
- DOI:10.1109/jsyst.2020.2967847
- 发表时间:2020-02
- 期刊:IEEE Systems Journal
- 影响因子:4.4
- 作者:Lai Chang;Zhang Xinglin
- 通讯作者:Zhang Xinglin
数据更新时间:{{ journalArticles.updateTime }}
{{
item.title }}
{{ item.translation_title }}
- DOI:{{ item.doi || "--"}}
- 发表时间:{{ item.publish_year || "--" }}
- 期刊:{{ item.journal_name }}
- 影响因子:{{ item.factor || "--"}}
- 作者:{{ item.authors }}
- 通讯作者:{{ item.author }}
数据更新时间:{{ journalArticles.updateTime }}
{{ item.title }}
- 作者:{{ item.authors }}
数据更新时间:{{ monograph.updateTime }}
{{ item.title }}
- 作者:{{ item.authors }}
数据更新时间:{{ sciAawards.updateTime }}
{{ item.title }}
- 作者:{{ item.authors }}
数据更新时间:{{ conferencePapers.updateTime }}
{{ item.title }}
- 作者:{{ item.authors }}
数据更新时间:{{ patent.updateTime }}
其他文献
其他文献
{{
item.title }}
{{ item.translation_title }}
- DOI:{{ item.doi || "--" }}
- 发表时间:{{ item.publish_year || "--"}}
- 期刊:{{ item.journal_name }}
- 影响因子:{{ item.factor || "--" }}
- 作者:{{ item.authors }}
- 通讯作者:{{ item.author }}
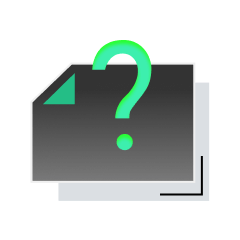
内容获取失败,请点击重试
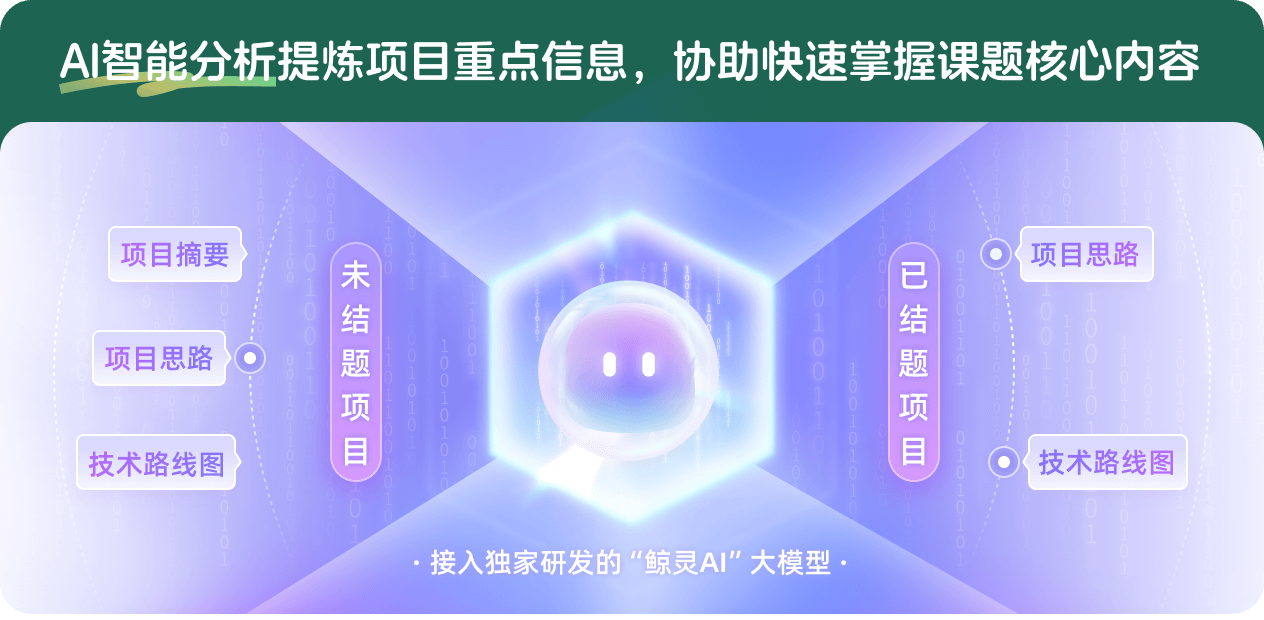
查看分析示例
此项目为已结题,我已根据课题信息分析并撰写以下内容,帮您拓宽课题思路:
AI项目摘要
AI项目思路
AI技术路线图
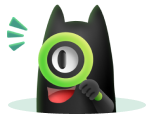
请为本次AI项目解读的内容对您的实用性打分
非常不实用
非常实用
1
2
3
4
5
6
7
8
9
10
您认为此功能如何分析更能满足您的需求,请填写您的反馈:
张幸林的其他基金
面向联邦学习的智能边缘网络服务关键技术研究
- 批准号:62372185
- 批准年份:2023
- 资助金额:50 万元
- 项目类别:面上项目
群体感知的隐私保护方法
- 批准号:61632013
- 批准年份:2016
- 资助金额:265.0 万元
- 项目类别:重点项目
群智感知中动态用户的在线激励机制研究
- 批准号:61502178
- 批准年份:2015
- 资助金额:21.0 万元
- 项目类别:青年科学基金项目
相似国自然基金
{{ item.name }}
- 批准号:{{ item.ratify_no }}
- 批准年份:{{ item.approval_year }}
- 资助金额:{{ item.support_num }}
- 项目类别:{{ item.project_type }}
相似海外基金
{{
item.name }}
{{ item.translate_name }}
- 批准号:{{ item.ratify_no }}
- 财政年份:{{ item.approval_year }}
- 资助金额:{{ item.support_num }}
- 项目类别:{{ item.project_type }}