海量数据小训练样本集环境下的高效图像理解
项目介绍
AI项目解读
基本信息
- 批准号:61572451
- 项目类别:面上项目
- 资助金额:66.0万
- 负责人:
- 依托单位:
- 学科分类:F0210.计算机图像视频处理与多媒体技术
- 结题年份:2019
- 批准年份:2015
- 项目状态:已结题
- 起止时间:2016-01-01 至2019-12-31
- 项目参与者:李礼; 卢庆博; 沈旭; 孙韶言; 李亚; 贾强槐; 董哲; 林彬; 郭聪;
- 关键词:
项目摘要
With the amount of digital images on the Internet increasing dramatically, how to annotate images with semantic labels for realizing semantic-based image retrieval and management becomes an increasingly important challenge. In image understanding, large amount of annotated training samples are essentially required for building robust semantic models. Although billions of images are there on the Internet, when training a semantic model for a specific concept, the valid samples (training images) which are truly related to the concept are few. Besides, how to collect those related training samples from the Internet is another challenging problem. Therefore, under the environment of massive web image resources, image understanding still faces the problem of small training set. In this proposal, we try to tackle this problem by making efforts from two directions: training set expansion and model optimization. For training set expansion, we propose to utilize image search quality assessment to guide training sample collection from Internet, and propose to jointly use training samples from closely related concepts. For model optimization, we try to improve the robustness and generalization ability of both shallow learning models and deep learning models, by proposing jointly optimized multi-task learning, scale- and rotation-invariant convolutional neural networks, and continuous dropout. Therefore, these models can achieve good performance for image understanding in the case of small training set. The aim of this project is to make a breakthrough in the area of image semantic understanding and we believe it will advance the development of both theoretical research and techniques in image semantic understanding.
随着互联网图像数量急剧增长,如何对图像进行语义标注以实现海量图像的检索和管理是目前面临的重要挑战。图像理解中需要大量已标注样本用于训练鲁棒的语义模型。虽然互联网上充斥着海量图像数据,然而在训练某一特定语义模型时,与该语义概念相关的有效数据非常少,而且如何从网络上获取这些相关训练图像也是需要解决的问题。因此,尽管在海量网络图像资源环境下,图像理解依然面临小训练样本集这一严峻问题。为解决该难题,本课题从训练数据集扩充和模型优化两个方向共同努力。在数据方面,提出以图像检索结果质量评价为指导从网络图像中扩充训练集,并联合使用相关语义概念的训练数据。在模型方面,提出联合优化的多任务学习、尺度和旋转不变的卷积网络及连续Dropout模型,以提升浅层学习和深度学习模型的鲁棒性,使之在小训练集下依然能取得不错的效果。本课题预期在图像理解的理论、方法和技术上有较大突破,推动图像语义理解的基础理论和技术研究。
结项摘要
尽管在海量网络图像资源环境下,图像理解依然面临小训练样本集这一严峻问题。为了解决小样本训练集带来的特征表达不稳定和模型过拟合问题,项目组主要从两个方面展开研究:1)多任务学习和特征表达学习;2)提升模型鲁棒性。项目组经过四年研究,在这两个方面取得了一系列创新研究成果。项目组提出的特征和模型参数联合优化的多任务学习方法,通过将多个任务的特征映射到一个共同的特征空间提升任务之间的相关性,泛化误差的理论分析证明我们的方法可以更好地衡量任务之间的相关性。项目组提出的分类和特征表达联合学习框架同时考虑了分类类别信息和训练样本间的局部空间分布信息,可以有效提升分类和特征表达的性能。项目组提出了一种非常简单但是有效的方法来提升卷积神经网络的旋转和平移不变性,通过对卷积层特征图进行块重排的操作将位置不变性编码到模型参数中。该块重排序模块可以很容易地嵌入到任意标准卷积神经网络框架中。受人脑中神经网络的激活模式启发,项目组将传统的二值Dropout扩展成连续Dropout。理论分析和实验证明连续Dropout可以更好地防止特征检测器在深层神经网络中的相互依赖。项目执行期间项目组发表学术论文28篇,其中IEEE和ACM汇刊论文8篇,CCF A类会议论文10篇;申请发明专利3件,其中授权发明专利1件。该项目培养博士毕业生4人,硕士毕业生4人。项目组圆满完成了项目计划书制定的研究计划,达到了预期的研究目标。
项目成果
期刊论文数量(7)
专著数量(0)
科研奖励数量(0)
会议论文数量(14)
专利数量(1)
Monet: A System for Reliving Your Memories by Theme-Based Photo Storytelling
莫奈:通过基于主题的照片讲故事来重温记忆的系统
- DOI:10.1109/tmm.2016.2614185
- 发表时间:2016
- 期刊:IEEE Transactions on Multimedia
- 影响因子:7.3
- 作者:Wu Yue;Shen Xu;Mei Tao;Tian Xinmei;Yu Nenghai;Rui Yong
- 通讯作者:Rui Yong
PageSense: Toward Stylewise Contextual Advertising via Visual Analysis of Web Pages
PageSense:通过网页视觉分析实现风格化的上下文广告
- DOI:10.1109/tcsvt.2016.2598702
- 发表时间:2018
- 期刊:IEEE Trans. Circuits Syst. Video Techn.
- 影响因子:--
- 作者:Tao Mei;Lusong Li;Xinmei Tian;Dacheng Tao;Chong-Wah Ngo
- 通讯作者:Chong-Wah Ngo
Eigenvector-Based Distance Metric Learning for Image Classification and Retrieval
用于图像分类和检索的基于特征向量的距离度量学习
- DOI:10.1145/3340262
- 发表时间:2019-08
- 期刊:ACM Trans. Multim. Comput. Commun. Appl.
- 影响因子:--
- 作者:Zhangcheng Wang;Ya Li;Richang Hong;Xinmei Tian
- 通讯作者:Xinmei Tian
Eigenfunction-Based Multitask Learning in a Reproducing Kernel Hilbert Space
再生核希尔伯特空间中基于特征函数的多任务学习
- DOI:10.1109/tnnls.2018.2873649
- 发表时间:2019-06-01
- 期刊:IEEE TRANSACTIONS ON NEURAL NETWORKS AND LEARNING SYSTEMS
- 影响因子:10.4
- 作者:Tian, Xinmei;Li, Ya;Tao, Dacheng
- 通讯作者:Tao, Dacheng
Accelerating Convolutional Neural Networks by Removing Interspatial and Interkernel Redundancies
通过消除空间间和内核间冗余来加速卷积神经网络
- DOI:10.1109/tcyb.2018.2873762
- 发表时间:2020-02
- 期刊:IEEE Transactions on Cybernetics
- 影响因子:11.8
- 作者:Linghua Zeng;Xinmei Tian
- 通讯作者:Xinmei Tian
数据更新时间:{{ journalArticles.updateTime }}
{{
item.title }}
{{ item.translation_title }}
- DOI:{{ item.doi || "--"}}
- 发表时间:{{ item.publish_year || "--" }}
- 期刊:{{ item.journal_name }}
- 影响因子:{{ item.factor || "--"}}
- 作者:{{ item.authors }}
- 通讯作者:{{ item.author }}
数据更新时间:{{ journalArticles.updateTime }}
{{ item.title }}
- 作者:{{ item.authors }}
数据更新时间:{{ monograph.updateTime }}
{{ item.title }}
- 作者:{{ item.authors }}
数据更新时间:{{ sciAawards.updateTime }}
{{ item.title }}
- 作者:{{ item.authors }}
数据更新时间:{{ conferencePapers.updateTime }}
{{ item.title }}
- 作者:{{ item.authors }}
数据更新时间:{{ patent.updateTime }}
其他文献
其他文献
{{
item.title }}
{{ item.translation_title }}
- DOI:{{ item.doi || "--" }}
- 发表时间:{{ item.publish_year || "--"}}
- 期刊:{{ item.journal_name }}
- 影响因子:{{ item.factor || "--" }}
- 作者:{{ item.authors }}
- 通讯作者:{{ item.author }}
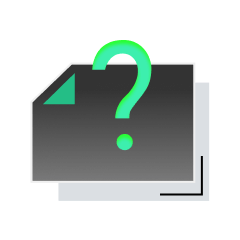
内容获取失败,请点击重试
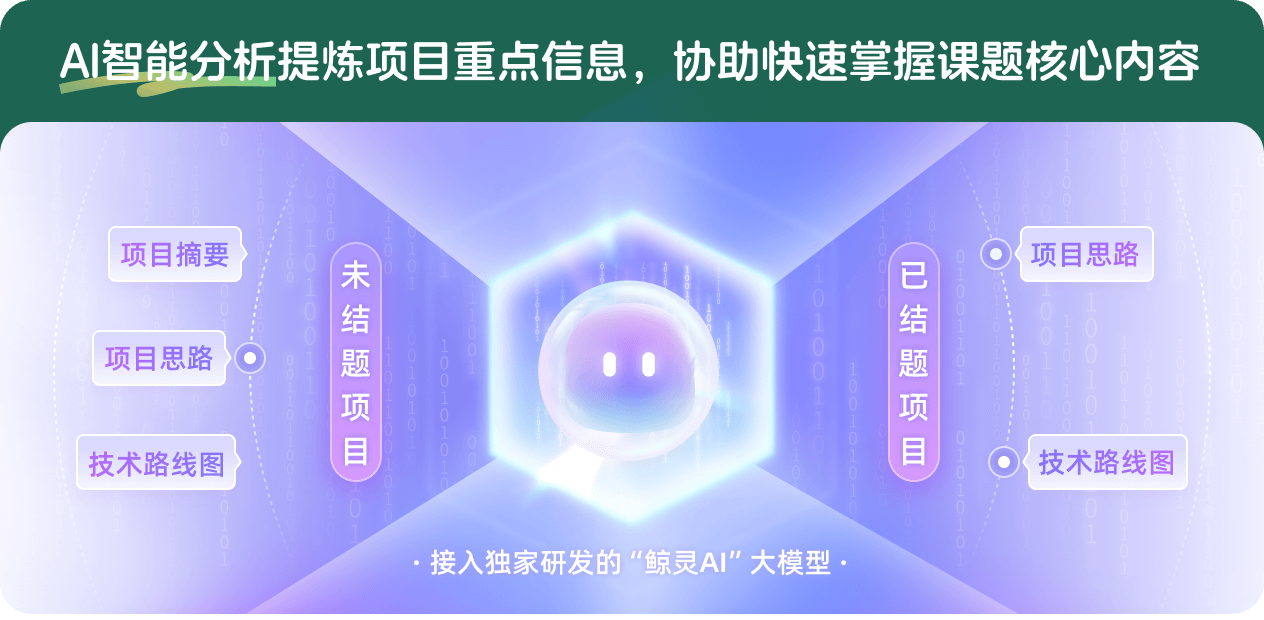
查看分析示例
此项目为已结题,我已根据课题信息分析并撰写以下内容,帮您拓宽课题思路:
AI项目摘要
AI项目思路
AI技术路线图
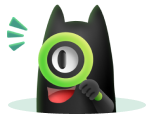
请为本次AI项目解读的内容对您的实用性打分
非常不实用
非常实用
1
2
3
4
5
6
7
8
9
10
您认为此功能如何分析更能满足您的需求,请填写您的反馈:
田新梅的其他基金
高效鲁棒的行人重识别研究
- 批准号:61872329
- 批准年份:2018
- 资助金额:64.0 万元
- 项目类别:面上项目
遥感图象解译竞赛设计与实施
- 批准号:61350002
- 批准年份:2013
- 资助金额:30.0 万元
- 项目类别:专项基金项目
基于网络知识和人工知识的图像语义建模方法研究
- 批准号:61201413
- 批准年份:2012
- 资助金额:27.0 万元
- 项目类别:青年科学基金项目
相似国自然基金
{{ item.name }}
- 批准号:{{ item.ratify_no }}
- 批准年份:{{ item.approval_year }}
- 资助金额:{{ item.support_num }}
- 项目类别:{{ item.project_type }}
相似海外基金
{{
item.name }}
{{ item.translate_name }}
- 批准号:{{ item.ratify_no }}
- 财政年份:{{ item.approval_year }}
- 资助金额:{{ item.support_num }}
- 项目类别:{{ item.project_type }}