面向大数据的预期形状导向压缩感知成像的重建方法研究
项目介绍
AI项目解读
基本信息
- 批准号:61472301
- 项目类别:面上项目
- 资助金额:80.0万
- 负责人:
- 依托单位:
- 学科分类:F0210.计算机图像视频处理与多媒体技术
- 结题年份:2018
- 批准年份:2014
- 项目状态:已结题
- 起止时间:2015-01-01 至2018-12-31
- 项目参与者:石光明; 吴金建; 李岚; 王立志; 刘作志; 李永波; 李超; 邓健; 韩亭玉;
- 关键词:
项目摘要
Compressed sensing theory plays a beneficial role in the ability of signal acquirement. However, the efficiency and quality of signal reconstruction cannot meet an urgent demand of the fields such as remote sensing imaging, medical diagnostic imaging and calculation imaging. Different from that the traditional compressed sensing reconstruction considers the whole and detail simultaneously, the calculation process of human visual perception is to reconstruct visible geometries partitioned by color, gray scale and texture, based on the prior knowledge and the expectation. How to reconstruct signals, to reconstruct the main information with a higher quality and to improve the reconstruction efficiency according to the human visual perception mechanism are the main issues studied in this project. Firstly, in this project, the sparse representation is extended from independent and identically distributed single signal to big data group signals which have high redundancy in feature structure. Therefore, it can characterize humans' prior knowledge and express the expected information more effectively. Secondly, an innovative idea that signals can be reconstructed according to expected shape is proposed, which not only keeps the high quality reconstruction of main information, but also reduces the redundancy. Lastly, an optimization strategy based on target constraints is proposed, which can improve the reconstruction efficiency. The contributions of this project include: 1) the sparse representation of group signals with associated structural features, 2) the optimized reconstruction strategy under constraints with expected shape guidance. The research achievements of this project can provide a good theoretical and methodological foundation for information mining and value discovery for big data in the current cloud computing environment.
压缩感知理论对于提高信号获取效率和能力非常有利,但其重构效率和质量还远不能满足遥感探测成像、医学诊断影像、计算成像等领域的需求。传统压缩感知重构是同时考虑整体与细节,而人类视觉感知的计算过程是用先验知识和预期重构出由颜色、灰阶、纹理等划分的可见面几何形状。如何按人类视觉感知信息机理重构信号,并高质量反演主要信息和提高重构效率,是本课题重点攻克的目标。本课题首先将稀疏表示从独立同分布的单信号内部扩展到特征结构高度冗余的大数据中的群信号,从而更有效地刻画人们的先验知识和表达预期信息;其次是提出了按预期形状重构信号的创新思想,既保留影像中重要信息高质量重构,又可减少冗余数据产生;最后提出基于预期形状导向约束的优化策略,可保证重构效率的提高。本项目的创新点:群体信号结构特征关联稀疏表示,预期形状导向约束优化重构策略。项目的研究成果可为当前云环境下大数据中的信息挖据、价值发现提供良好的理论和方法基础
结项摘要
压缩感知理论对于提高信号获取效率和能力非常有利,但其重构效率和质量还远不能满足遥感探测成像、医学诊断影像、计算成像等领域的需求。传统压缩感知重构是同时考虑整体与细节,而人类视觉感知的计算过程是用先验知识和预期重构出由颜色、灰阶、纹理等划分的可见面几何形状。如何按人类视觉感知信息机理重构信号,并高质量反演主要信息和提高重构效率,是本课题重点攻克的目标。已完成研究内容包括:(1)将稀疏表示从独立同分布的单信号内部扩展到特征结构高度冗余的大数据中的群信号,从而更有效地刻画人们的先验知识和表达预期信息;(2)提出了按预期形状重构信号的创新思想,既保留影像中重要信息高质量重构,又可减少冗余数据产生;(3)提出基于预期形状导向约束的优化策略,可保证重构效率的提高;(4)本项目完成了群体信号结构特征关联稀疏表示,预期形状导向约束优化重构策略。项目的研究成果可为当前云环境下大数据中的信息挖据、价值发现提供良好的理论和方法基础。总之,研究成果达到预期效果。
项目成果
期刊论文数量(13)
专著数量(0)
科研奖励数量(1)
会议论文数量(21)
专利数量(10)
Single-Shot Dense Depth Sensing with Color Sequence Coded Fringe Pattern.
具有颜色序列编码条纹图案的单次密集深度感测
- DOI:10.3390/s17112558
- 发表时间:2017-11-06
- 期刊:Sensors (Basel, Switzerland)
- 影响因子:--
- 作者:Li F;Zhang B;Shi G;Niu Y;Li R;Yang L;Xie X
- 通讯作者:Xie X
Compressive spectral imaging using variable number of measurements
使用可变数量的测量进行压缩光谱成像
- DOI:--
- 发表时间:--
- 期刊:applied optics
- 影响因子:1.9
- 作者:Yaohai Lin;Xuemei Xie;Guangming Shi;Danhua Liu;Dahua Gao
- 通讯作者:Dahua Gao
Maximum a posteriori-based depth sensing with a single-shot maze pattern
具有单次迷宫模式的最大后验深度感测
- DOI:10.1364/oe.25.025332
- 发表时间:2017
- 期刊:OPTICS EXPRESS
- 影响因子:3.8
- 作者:Li Ruodai;Li Fu;Niu Yi;Shi Guangming;Yang Lili;Xie Xuemei
- 通讯作者:Xie Xuemei
Single-shot dense depth sensing with frequency-division multiplexing fringe projection
采用频分复用条纹投影的单次密集深度传感
- DOI:10.1016/j.jvcir.2017.03.018
- 发表时间:2017
- 期刊:Journal of Visual Communication and Image Representation
- 影响因子:2.6
- 作者:Yang Lili;Li Fu;Xiong Zhiwei;Shi Guangming;Niu Yi;Li Ruodai
- 通讯作者:Li Ruodai
Object-dependent sparse representation for extracellular spike detection
用于细胞外尖峰检测的对象相关稀疏表示
- DOI:10.1016/j.neucom.2017.05.082
- 发表时间:2017-11
- 期刊:Neurocomputing
- 影响因子:6
- 作者:Shi Guangming;Liu Zuozhi;Wang Xiaotian;Li Chengyu T.;Gu Xiaowei
- 通讯作者:Gu Xiaowei
数据更新时间:{{ journalArticles.updateTime }}
{{
item.title }}
{{ item.translation_title }}
- DOI:{{ item.doi || "--"}}
- 发表时间:{{ item.publish_year || "--" }}
- 期刊:{{ item.journal_name }}
- 影响因子:{{ item.factor || "--"}}
- 作者:{{ item.authors }}
- 通讯作者:{{ item.author }}
数据更新时间:{{ journalArticles.updateTime }}
{{ item.title }}
- 作者:{{ item.authors }}
数据更新时间:{{ monograph.updateTime }}
{{ item.title }}
- 作者:{{ item.authors }}
数据更新时间:{{ sciAawards.updateTime }}
{{ item.title }}
- 作者:{{ item.authors }}
数据更新时间:{{ conferencePapers.updateTime }}
{{ item.title }}
- 作者:{{ item.authors }}
数据更新时间:{{ patent.updateTime }}
其他文献
带有区间不确定性的约束平差算法及应用
- DOI:10.16251/j.cnki.1009-2307.2018.05.017
- 发表时间:2018
- 期刊:测绘科学
- 影响因子:--
- 作者:肖兆兵;宋迎春;谢雪梅
- 通讯作者:谢雪梅
不确定性平差算法在沉降灰色模型中的应用
- DOI:--
- 发表时间:2019
- 期刊:测绘地理信息
- 影响因子:--
- 作者:肖兆兵;宋迎春;谢雪梅
- 通讯作者:谢雪梅
肿瘤抑制因子PTEN亚细胞定位与缺血-缺氧性脑损伤
- DOI:--
- 发表时间:2016
- 期刊:基础医学与临床
- 影响因子:--
- 作者:赵婧;谢雪梅;陈玉蓉
- 通讯作者:陈玉蓉
参数带有区间约束的平差模型迭代算法
- DOI:--
- 发表时间:2018
- 期刊:测绘学报
- 影响因子:--
- 作者:谢雪梅;宋迎春;肖兆兵
- 通讯作者:肖兆兵
旅游目的地形象的符号隐喻关联研究——以陕西省为例
- DOI:--
- 发表时间:--
- 期刊:资源科学
- 影响因子:--
- 作者:谢雪梅;白凯;孙天宇
- 通讯作者:孙天宇
其他文献
{{
item.title }}
{{ item.translation_title }}
- DOI:{{ item.doi || "--" }}
- 发表时间:{{ item.publish_year || "--"}}
- 期刊:{{ item.journal_name }}
- 影响因子:{{ item.factor || "--" }}
- 作者:{{ item.authors }}
- 通讯作者:{{ item.author }}
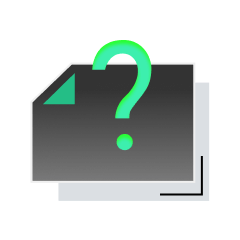
内容获取失败,请点击重试
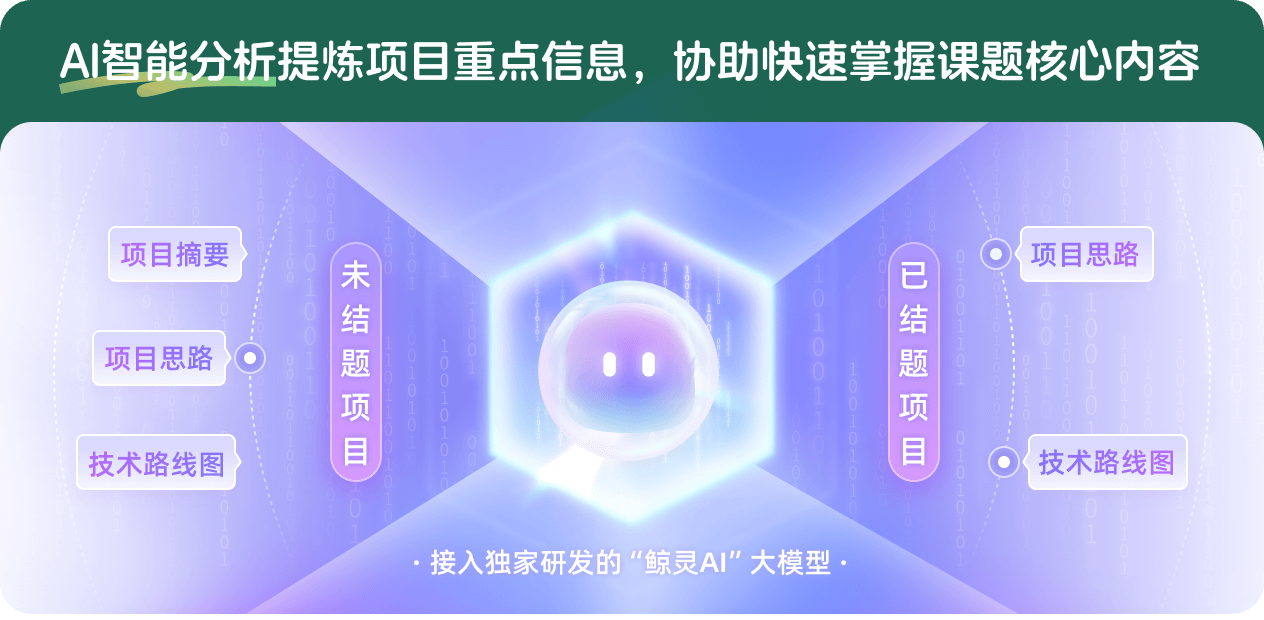
查看分析示例
此项目为已结题,我已根据课题信息分析并撰写以下内容,帮您拓宽课题思路:
AI项目摘要
AI项目思路
AI技术路线图
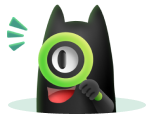
请为本次AI项目解读的内容对您的实用性打分
非常不实用
非常实用
1
2
3
4
5
6
7
8
9
10
您认为此功能如何分析更能满足您的需求,请填写您的反馈:
谢雪梅的其他基金
多带非均匀方向滤波器组和方向小波的理论、设计和应用
- 批准号:61072104
- 批准年份:2010
- 资助金额:35.0 万元
- 项目类别:面上项目
M带方向滤波器组的构造及其对图像纹理方向的检测研究
- 批准号:60672125
- 批准年份:2006
- 资助金额:24.0 万元
- 项目类别:面上项目
相似国自然基金
{{ item.name }}
- 批准号:{{ item.ratify_no }}
- 批准年份:{{ item.approval_year }}
- 资助金额:{{ item.support_num }}
- 项目类别:{{ item.project_type }}
相似海外基金
{{
item.name }}
{{ item.translate_name }}
- 批准号:{{ item.ratify_no }}
- 财政年份:{{ item.approval_year }}
- 资助金额:{{ item.support_num }}
- 项目类别:{{ item.project_type }}