Validation of a Virtual Still Face Procedure and Deep Learning Algorithms to Assess Infant Emotion Regulation and Infant-Caregiver Interactions in the Wild
验证虚拟静脸程序和深度学习算法,以评估野外婴儿情绪调节和婴儿与护理人员的互动
基本信息
- 批准号:10777825
- 负责人:
- 金额:$ 62.28万
- 依托单位:
- 依托单位国家:美国
- 项目类别:
- 财政年份:2023
- 资助国家:美国
- 起止时间:2023-09-30 至 2028-07-31
- 项目状态:未结题
- 来源:
- 关键词:
项目摘要
“This study is part of the NIH’s Helping to End Addiction Long-term (HEAL) initiative to speed scientific solutions to the national opioid public health crisis. The NIH HEAL Initiative bolsters research across NIH to improve treatment for opioid misuse and addiction.”
Moment-to-moment infant-parent interactions are a central context in which infants learn to regulate emotions. Investigating infant-parent interactions in which emotion regulation unfolds is particularly important for infants at risk for emotion dysregulation and/or relationship disturbance, including infants with prenatal substance exposure. Yet, current state-of-the art methods to assess infant emotion regulation and infant-parent interaction predominantly rely on brief laboratory tasks. These procedures pose burdens on participants, especially families
experiencing demographic and psychosocial risk, and place limits on generalizability and ecological validity of findings. Technological advances in (a) machine learning methods, including deep learning approaches that mine for complex patterns in raw unlabeled data, and (b) wearable sensors have the potential to transform our ability to capture infants’ moment-to-moment emotional experiences in their real-world environments, while also lowering burden on families participating in infant research. With these issues in mind, we will develop next-generation methods to assess infant emotion regulation and infant-parent interaction. In doing so, we will use
LittleBeats, an infant multimodal wearable device developed by our team, to collect time-synced data on infant and parent vocalizations (via microphone), infant motor activity (via motion sensor), and infant cardiac vagal tone (via electrocardiogram [ECG]) for extended periods of time (~8-10 hours per day) in the home. We propose three specific aims. First, we will validate a virtual visit protocol for the gold-standard Still Face Paradigm, which is typically conducted in a laboratory setting, for assessing emotion regulation among infants during the first year of life. Second, we will validate multimodal deep learning algorithms to detect infant emotional states in real time
using LittleBeats audio, ECG and motion data. Third, we will validate deep learning algorithms to detect and label vocalization types of infants (babble, fuss, cry, laugh) and parents (infant-direct speech, adult-directed speech, sing, laugh), which create the build blocks of infant-parent vocal interactions, such as turn taking. By bringing together innovative wearable technology with cutting-edge deep learning algorithms, we aim to advance understanding of the mechanisms through which prenatal substance exposures contribute to adverse outcomes. Further, prenatal substance exposure is a heterogeneous phenomena that transacts with environmental risk and
protective factors, thereby making a one-size-fits-all approach ineffective. By monitoring moment-to-moment changes in infants’ emotion regulation, combined with deep learning algorithms that detect and classify infant-parent interactions during moments when infant show signs of distress, the proposed methods have the potential to transform our understanding of the dynamic processes through which prenatal substance exposure leads to poor outcomes and pinpoint protective factors that promote optimal development.
“这项研究是NIH的一部分,有助于结束长期(治愈)倡议,以加快美国阿片类药物公共卫生危机的科学解决方案。NIHHeal Heal Initiative Bolsters跨NIH的研究,以改善对阿片类药物滥用和成瘾的治疗。”
瞬间的婴儿与父母的互动是婴儿学会调节情绪的中心背景。调查婴儿的互动,其中情绪调节的展开尤为重要,这对于患有情绪失调和/或关系灾难的婴儿(包括具有产前药物暴露的婴儿)尤其重要。然而,当前评估婴儿情绪调节和婴儿互动的最新方法主要依赖于简短的实验室任务。这些程序为参与者,尤其是家庭带来了伯伦斯
经验人群和社会心理风险,并对发现的普遍性和生态有效性限制。 (a)机器学习方法的技术进步,包括针对原始数据的复杂模式的深度学习方法,以及(b)可穿戴的传感器有可能改变我们捕捉婴儿在现实世界环境中捕捉婴儿瞬间的情感体验的能力,同时还可以降低对婴儿研究的家庭的燃烧。考虑到这些问题,我们将开发下一代方法来评估婴儿的情绪调节和婴儿父母的互动。这样,我们将使用
LittleBeats是由我们的团队开发的婴儿多模式可穿戴设备,用于收集有关婴儿和家长发声的时间同步数据(通过麦克风),婴儿运动活动(通过运动传感器)和婴儿心脏迷走神经张力(通过心电图[ECG])在房屋中的时间(每天约8-10个小时)。我们提出了三个具体目标。首先,我们将验证金标准仍然面对范式的虚拟访问协议,该范式通常在实验室环境中进行,以评估婴儿第一年的情绪调节。其次,我们将验证多模式深度学习算法,以实时检测婴儿的情绪状态
使用LittleBeats音频,ECG和运动数据。第三,我们将验证深度学习算法来检测和标记婴儿的发声类型(Babble,大惊小怪,哭泣,笑声)和父母(婴儿指导的演讲,成人指导的演讲,唱歌,笑),从而创造了婴儿 - 父母的声音互动的建筑块,例如转弯。通过将创新的可穿戴技术与最先进的深度学习算法汇总在一起,我们旨在促进对产前物质暴露有助于不良后果的机制的理解。此外,产前物质暴露是一种异质现象,具有环境风险和
保护因素,从而使所有尺寸适合的方法无效。通过监视婴儿情绪调节的时刻变化,再加上深度学习算法,这些算法在婴儿表现出困扰的迹象时检测和分类了婴儿与父母的相互作用,提出的方法有可能改变我们对动态过程的理解,从而通过这种动态过程导致了较差的衰落并促进了较差的保护因素,从而促进了保护因素。
项目成果
期刊论文数量(0)
专著数量(0)
科研奖励数量(0)
会议论文数量(0)
专利数量(0)
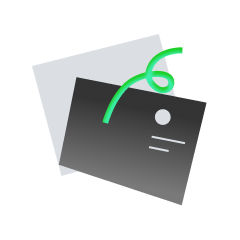
暂无数据
数据更新时间:2024-06-01
MARK ALLAN HASEGAW...的其他基金
Audiovisual Description and Recognition of Dysarthric Speech
构音障碍语音的视听描述和识别
- 批准号:72300767230076
- 财政年份:2006
- 资助金额:$ 62.28万$ 62.28万
- 项目类别:
Audiovisual Description and Recognition of Dysarthric Speech
构音障碍语音的视听描述和识别
- 批准号:70750537075053
- 财政年份:2006
- 资助金额:$ 62.28万$ 62.28万
- 项目类别:
FACTOR ANALYSIS OF MRI DERIVED ARTICULATOR SHAPES
MRI 得出的咬合架形状的因素分析
- 批准号:28721242872124
- 财政年份:1999
- 资助金额:$ 62.28万$ 62.28万
- 项目类别:
FACTOR ANALYSIS OF MRI DERIVED ARTICULATOR SHAPES
MRI 得出的咬合架形状的因素分析
- 批准号:25222592522259
- 财政年份:1998
- 资助金额:$ 62.28万$ 62.28万
- 项目类别:
相似国自然基金
成人型弥漫性胶质瘤患者语言功能可塑性研究
- 批准号:82303926
- 批准年份:2023
- 资助金额:30 万元
- 项目类别:青年科学基金项目
MRI融合多组学特征量化高级别成人型弥漫性脑胶质瘤免疫微环境并预测术后复发风险的研究
- 批准号:82302160
- 批准年份:2023
- 资助金额:30 万元
- 项目类别:青年科学基金项目
成人免疫性血小板减少症(ITP)中血小板因子4(PF4)通过调节CD4+T淋巴细胞糖酵解水平影响Th17/Treg平衡的病理机制研究
- 批准号:82370133
- 批准年份:2023
- 资助金额:49 万元
- 项目类别:面上项目
SMC4/FoxO3a介导的CD38+HLA-DR+CD8+T细胞增殖在成人斯蒂尔病MAS发病中的作用研究
- 批准号:82302025
- 批准年份:2023
- 资助金额:30 万元
- 项目类别:青年科学基金项目
融合多源异构数据应用深度学习预测成人肺部感染病原体研究
- 批准号:82302311
- 批准年份:2023
- 资助金额:30 万元
- 项目类别:青年科学基金项目
相似海外基金
Grounding models of category learning in the visual experiences of young children
幼儿视觉体验中类别学习的基础模型
- 批准号:1070406210704062
- 财政年份:2022
- 资助金额:$ 62.28万$ 62.28万
- 项目类别:
Molecular Pathobiology of Alport Syndrome
阿尔波特综合征的分子病理学
- 批准号:1070514710705147
- 财政年份:2022
- 资助金额:$ 62.28万$ 62.28万
- 项目类别:
Grounding models of category learning in the visual experiences of young children
幼儿视觉体验中类别学习的基础模型
- 批准号:1042818210428182
- 财政年份:2022
- 资助金额:$ 62.28万$ 62.28万
- 项目类别:
Augmem: A Novel Digital Cognitive Assessment for the Early Detection of Alzheimer's Disease
Augmem:一种用于早期检测阿尔茨海默病的新型数字认知评估
- 批准号:1068822710688227
- 财政年份:2022
- 资助金额:$ 62.28万$ 62.28万
- 项目类别:
Identification of Trauma-related Features in EHR Data for Patients with Psychosis and Mood Disorders
精神病和情绪障碍患者 EHR 数据中创伤相关特征的识别
- 批准号:1042743310427433
- 财政年份:2021
- 资助金额:$ 62.28万$ 62.28万
- 项目类别: