Identification of Mild Cognitive Impairment using Machine Learning from Language and Behavior Markers
使用机器学习从语言和行为标记识别轻度认知障碍
基本信息
- 批准号:10212669
- 负责人:
- 金额:$ 228.64万
- 依托单位:
- 依托单位国家:美国
- 项目类别:
- 财政年份:2021
- 资助国家:美国
- 起止时间:2021-04-15 至 2025-03-31
- 项目状态:未结题
- 来源:
- 关键词:
项目摘要
Project Summary
Recent estimates indicate that Alzheimer’s disease (AD) may rank as the third leading cause of death
for older people in the United States, just behind heart disease and cancer. While scientists know that
AD involves a progressive brain cell failure, the reason why cells fail is still not clear. To understand the
progression of the disease, one of the keys is to investigate the cognitive changes in patients with mild
cognitive impairment (MCI). Even though biomarkers such as imaging and clinical functions are found
to be outstanding in differentiating AD patients from those with normal cognition (NC), studies suggest
that their discriminative power in early-stage MCI are rather limited. Detecting signals which distinguish
subjects with MCI from those with NC is challenging due to the low sensitivity and high variability of
current clinical measures such as annually assessed neuropsychological test results and self-reported
functional measurements. Moreover, even though in-vivo biomarkers such as beta-amyloid and tau can
be used as indicators of pathological progression towards AD, the screening of biomarkers are
prohibitively expensive to be widely used among pre-symptomatic individuals in the outpatient setting.
We hypothesize that progressive cognitive impact from MCI has elicited detectable changes in the way
people talk and behave, which can be sensed by inexpensive and accessible sensors and leveraged
by machine learning (ML) algorithms to build predictive models for quantifying the risk of MCI. Our
preliminary results on a small cohort indicated that there are significant differences between MCI and
NC subjects during a semi-structured conversation, and ML algorithms can use such differences for
differentiating MCI and NC with promising performance. Our preliminary results in behavior monitoring
also suggest highly predictive performance using temporal patterns of behavior signals. In this project,
we plan to build upon our initial success and conduct comprehensive studies on language and behavior
markers in larger-scale cohorts to build high-performance and interpretable ML models for screening
MCI. Our three Specific Aims are: (1) Discover language markers and develop predictive models
characterizing MCI. Using interview recordings from the I-CONECT project, we will use natural
language processing and ML algorithms to extract linguistic and acoustic markers and develop multi-
modal learning algorithms to fuse the two types of information. (2) Discover behavior markers and
develop predictive models characterizing MCI. Using the in-home monitoring data from ORCATECH,
we will extract short-term and long-term behavior patterns and integrate multi-granularity behavior
markers to differentiate MCI and NC. (3) Linking language and behavior markers with an information
framework. We will use demographics and common clinical information to profile the patients and match
the two cohorts via certain similarity metrics, creating complementary features for improved prediction.
项目摘要
最近的估计表明,阿尔茨海默氏病(AD)可能是死亡的第三大原因
对于美国的老年人,就在心脏病和癌症之后。虽然科学家知道
AD涉及进行性脑细胞衰竭,原因仍然无法清楚。理解
疾病的进展,其中之一是研究中部患者的认知变化
认知障碍(MCI)。即使发现了成像和临床功能之类的生物标志物
为了使AD患者与正常认知患者(NC)的出色表现出色,研究表明
他们在早期MCI中的判别力量相当有限。检测有区别的信号
由于敏感性低和高可变性,具有NC患者的MCI受试者是具有挑战性的
当前的临床指标,例如每年评估神经心理学测试结果并自我报告
功能测量。而且,即使体内生物标志物(例如β-淀粉样蛋白和tau)也可以
用作病理发展向AD的指标,生物标志物的筛查是
在门诊环境中广泛使用的症状较高。
我们假设MCI的渐进认知影响引起了可检测的方式
人们说话和行为,可以通过便宜且可访问的传感器来感知和杠杆
通过机器学习(ML)算法来构建预测模型,以量化MCI的风险。我们的
小队列的初步结果表明,MCI和
在半结构化对话中的NC受试者,ML算法可以使用此类差异
通过有希望的表现区分MCI和NC。我们在行为监控的初步结果
还建议使用行为信号的临时模式提出高度预测性的性能。在这个项目中,
我们计划以最初的成功为基础,并就语言和行为进行全面研究
大规模同伙的标记,以建立高性能和可解释的ML模型用于筛选
MCI。我们的三个具体目的是:(1)发现语言标记并开发预测模型
表征MCI。使用I-Conect项目的访谈记录,我们将使用自然
语言处理和ML算法提取语言和声学标记,并发展多种多样
模态学习算法融合了两种类型的信息。 (2)发现行为标记和
开发表征MCI的预测模型。使用来自Orcatech的家庭监视数据,
我们将提取短期和长期行为模式以及综合的多粒性行为
区分MCI和NC的标记。 (3)将语言和行为标记与信息联系起来
框架。我们将使用人口统计和常见的临床信息来介绍患者并匹配
通过某些相似性指标,这两个队列,创建了完整的功能以改进预测。
项目成果
期刊论文数量(0)
专著数量(0)
科研奖励数量(0)
会议论文数量(0)
专利数量(0)
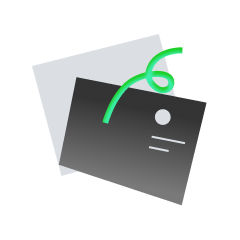
暂无数据
数据更新时间:2024-06-01
HIROKO Hayama DODG...的其他基金
Identification of Mild Cognitive Impairment using Machine Learning from Language and Behavior Markers
使用机器学习从语言和行为标记识别轻度认知障碍
- 批准号:1070909410709094
- 财政年份:2021
- 资助金额:$ 228.64万$ 228.64万
- 项目类别:
Data Management and Statistical Core
数据管理与统计核心
- 批准号:1036903610369036
- 财政年份:2020
- 资助金额:$ 228.64万$ 228.64万
- 项目类别:
Data Management and Statistical Core
数据管理与统计核心
- 批准号:1020377210203772
- 财政年份:2020
- 资助金额:$ 228.64万$ 228.64万
- 项目类别:
Data Management and Statistical Core
数据管理与统计核心
- 批准号:1064103110641031
- 财政年份:2020
- 资助金额:$ 228.64万$ 228.64万
- 项目类别:
Web-enabled social interaction to delay cognitive decline among seniors with MCI: Phase I
基于网络的社交互动可延缓 MCI 老年人认知能力下降:第一阶段
- 批准号:93115849311584
- 财政年份:2017
- 资助金额:$ 228.64万$ 228.64万
- 项目类别:
Web-enabled social interaction to delay cognitive decline among seniors with MCI: Phase I
基于网络的社交互动可延缓 MCI 老年人认知能力下降:第一阶段
- 批准号:98982099898209
- 财政年份:2017
- 资助金额:$ 228.64万$ 228.64万
- 项目类别:
Web-enabled social interaction to delay cognitive decline among seniors with MCI: Phase I Administrative Supplement
基于网络的社交互动可延缓 MCI 老年人认知能力下降:第一阶段行政补充
- 批准号:1036331010363310
- 财政年份:2017
- 资助金额:$ 228.64万$ 228.64万
- 项目类别:
Web-enabled social interaction to delay cognitive decline among seniors with MCI: Phase I
基于网络的社交互动可延缓 MCI 老年人认知能力下降:第一阶段
- 批准号:99303449930344
- 财政年份:2017
- 资助金额:$ 228.64万$ 228.64万
- 项目类别:
Conversational Engagement as a Means to Delay Onset AD: Phase II Administrative Supplement
对话参与作为延迟 AD 发作的一种手段:第二阶段行政补充
- 批准号:1005878410058784
- 财政年份:2016
- 资助金额:$ 228.64万$ 228.64万
- 项目类别:
Web-enabled social interaction to delay cognitive decline among seniors with MCI: Phase I
基于网络的社交互动可延缓 MCI 老年人认知能力下降:第一阶段
- 批准号:93487269348726
- 财政年份:2016
- 资助金额:$ 228.64万$ 228.64万
- 项目类别:
相似国自然基金
时空序列驱动的神经形态视觉目标识别算法研究
- 批准号:61906126
- 批准年份:2019
- 资助金额:24.0 万元
- 项目类别:青年科学基金项目
本体驱动的地址数据空间语义建模与地址匹配方法
- 批准号:41901325
- 批准年份:2019
- 资助金额:22.0 万元
- 项目类别:青年科学基金项目
大容量固态硬盘地址映射表优化设计与访存优化研究
- 批准号:61802133
- 批准年份:2018
- 资助金额:23.0 万元
- 项目类别:青年科学基金项目
IP地址驱动的多径路由及流量传输控制研究
- 批准号:61872252
- 批准年份:2018
- 资助金额:64.0 万元
- 项目类别:面上项目
针对内存攻击对象的内存安全防御技术研究
- 批准号:61802432
- 批准年份:2018
- 资助金额:25.0 万元
- 项目类别:青年科学基金项目
相似海外基金
Integrative Analysis of Adaptive Information Processing and Learning-Dependent Circuit Reorganization in the Auditory System
听觉系统中自适应信息处理和学习依赖电路重组的综合分析
- 批准号:1071592510715925
- 财政年份:2023
- 资助金额:$ 228.64万$ 228.64万
- 项目类别:
HEAR-HEARTFELT (Identifying the risk of Hospitalizations or Emergency depARtment visits for patients with HEART Failure in managed long-term care through vErbaL communicaTion)
倾听心声(通过口头交流确定长期管理护理中的心力衰竭患者住院或急诊就诊的风险)
- 批准号:1072329210723292
- 财政年份:2023
- 资助金额:$ 228.64万$ 228.64万
- 项目类别:
Hearing Loss, Prognosis, and Long-Term Impact of Otitis Media with Effusion in Children
儿童渗出性中耳炎的听力损失、预后和长期影响
- 批准号:1085214310852143
- 财政年份:2023
- 资助金额:$ 228.64万$ 228.64万
- 项目类别:
Concurrent volumetric imaging with multimodal optical systems
多模态光学系统的并行体积成像
- 批准号:1072749910727499
- 财政年份:2023
- 资助金额:$ 228.64万$ 228.64万
- 项目类别:
Dynamic neural coding of spectro-temporal sound features during free movement
自由运动时谱时声音特征的动态神经编码
- 批准号:1065611010656110
- 财政年份:2023
- 资助金额:$ 228.64万$ 228.64万
- 项目类别: