Collaborative Research: NCS-FR: Beyond the ventral stream: Reverse engineering the neurocomputational basis of physical scene understanding in the primate brain
合作研究:NCS-FR:超越腹侧流:逆向工程灵长类大脑中物理场景理解的神经计算基础
基本信息
- 批准号:2124136
- 负责人:
- 金额:$ 225万
- 依托单位:
- 依托单位国家:美国
- 项目类别:Standard Grant
- 财政年份:2021
- 资助国家:美国
- 起止时间:2021-10-01 至 2024-09-30
- 项目状态:已结题
- 来源:
- 关键词:
项目摘要
The last ten years have witnessed an astonishing revolution in AI, with deep neural networks suddenly approaching human-level performance on problems like recognizing objects in an image and words in an audio recording. But impressive as these feats are, they fall far short of human-like intelligence. The critical gap between current AI and human intelligence is that, beyond just classifying patterns of input, humans build mental models of the world. This project begins with the problem of physical scene understanding: how one extracts not just the identities and locations of objects in the visual world, but also the physical properties of those objects, their positions and velocities, their relationships to each other, the forces acting upon them, and the effects of forces that could be exerted on them. It is hypothesized that humans represent this information in a structured mental model of the physical world, and use that model to predict what will happen next, much as the physics engine in a video game generates physically plausible future states of virtual worlds. To test this idea, computational models of physical scene understanding will be built and tested for their ability to predict future states of the physical world in a variety of scenarios. Performance of these models will then be compared to humans and to more traditional deep network models, both in terms of their accuracy on each task, and their patterns of errors. Computational models that incorporate structured representations of the physical world will then be tested against standard convolutional neural networks in their ability to explain neural responses of the human brain (using fMRI) and the monkey brain (using direct neural recording). These computational models will provide the first explicit theories of how physical scene understanding might work in the human brain, at the same time advancing the ability of AI systems to solve the same problems. Because the ability to understand and predict the physical world is essential for planning any action, this work is expected to help advance many technologies that require such planning, from robotics to self-driving cars to brain-machine interfaces. Each of the participating labs will also expand their established track records of recruiting, training, and mentoring women and under-represented minorities at the undergraduate, graduate, and postdoctoral levels. Finally, the collaborating laboratories will continue and increase their involvement in the dissemination of science to the general public, via public talks, web sites, and outreach activities.Deep neural networks have revolutionized object recognition in computers as well as understanding of object recognition in the primate brain, but object recognition is just one aspect of vision, and the ventral stream is just one of many brain systems. Studying physical scene understanding is a step toward scaling this reverse-engineering approach up to the rest of the mind and brain. Predicting what will happen next and planning effective action requires understanding the physical basis and physical relationships in the visual world. Yet it is unknown how humans do this or how machines could. Both challenges are addressed in this project by the building of image computable, neurally mappable computational models of physical scene understanding and prediction (Thread I), and using these models as explicit hypotheses for how the brain might accomplish these tasks, which will then be tested with behavioral and neural data from humans (Thread II) and non-human primates (Thread III). This project aims to make a transformative leap in understanding: from small-scale, special-case models and isolated experimental tests to an integrated large-scale, general-purpose model of a major swathe of the primate brain, that functionally explains much of the immediate content of our perceptual experience in every scene that confronts us. The work will advance theory by developing the first image-computable models capable of human-level physical scene understanding and prediction. Beyond understanding of the mind and brain, this research is directly relevant to AI and robotics (which require physical scene understanding), and brain-machine interfaces (which require understanding of the relevant neural codes). For the broader research community, the project will a) develop public datasets, benchmark tasks, and challenges, b) host adversarial collaborations to address these challenges, and c) host interdisciplinary workshops linking research communities from psychology to AI to neuroscience to address the fundamental questions that span these fields.This award reflects NSF's statutory mission and has been deemed worthy of support through evaluation using the Foundation's intellectual merit and broader impacts review criteria.
在过去的十年中,AI的一场令人惊讶的革命目睹了深层神经网络突然在诸如识别图像中的对象和录音中的单词之类的问题上接近人级的表现。但是,这些壮举令人印象深刻,它们远远远远远远没有类似人类的智慧。当前的AI和人类智能之间的关键差距是,除了对投入的模式进行分类之外,人类还建立了世界的心理模型。该项目始于物理场景理解的问题:人们如何不仅提取视觉世界中对象的身份和位置,还提取这些对象的物理特性,它们的位置和速度,它们之间的关系,彼此之间的关系,作用于它们的力以及可以施加在它们上的力的力的效果。假设人类在物理世界的结构化心理模型中表示这些信息,并使用该模型来预测接下来会发生的事情,就像视频游戏中的物理引擎一样,可以产生虚拟世界的物理上合理的未来状态。为了测试这一想法,将建立和测试物理场景理解的计算模型,以预测各种情况下预测物理世界未来状态的能力。然后,这些模型的性能将与人类和更传统的深层网络模型进行比较,包括它们在每个任务的准确性及其错误模式。然后,将结合物理世界结构化表示的计算模型将在解释人脑(使用fMRI)和猴子大脑(使用直接神经记录)的能力中对标准卷积神经网络进行测试。这些计算模型将提供第一个明确的理论,即物理场景理解如何在人脑中起作用,同时促进了AI系统解决相同问题的能力。因为理解和预测物理世界的能力对于计划采取任何行动至关重要,因此这项工作有望帮助推进许多需要此类计划的技术,从机器人技术到自动驾驶汽车再到脑机界面。每个参与的实验室还将扩大其既定的招聘,培训和指导妇女以及本科生,研究生和博士后级别的少数民族的记录。最后,通过公众谈判,网站和外展活动,合作实验室将继续并增加他们参与对公众的传播。深处神经网络彻底改变了计算机中的对象识别以及对灵长类动物大脑中对象识别的理解,但是对象识别只是视觉的一个方面,而周围的流是许多脑脑系统的一个方面。研究物理场景的理解是扩展这种反向工程的一步,直接接近思想和大脑的其余部分。预测接下来会发生的事情并计划有效的行动需要了解视觉世界中的身体基础和身体关系。然而,尚不清楚人类是如何做到这一点或机器如何做到的。在本项目中,通过构建图像可计算,神经映射的物理场景理解和预测(线程I)的计算模型来解决这两个挑战,并将这些模型用作大脑如何完成这些任务的明确假设,然后将通过人类的行为和神经数据(线程II)和非人类灵长类动物(线程III)进行测试。该项目旨在实现理解力的变革性飞跃:从小规模的特殊案例模型和孤立的实验测试到灵长类动物大脑的主要散布的集成大规模的通用模型,从而在功能上解释了我们在我们面临的每个场景中我们感知体验的许多直接内容。这项工作将通过开发能够以人类水平的物理场景理解和预测的第一个可计算模型来推进理论。除了了解思想和大脑外,这项研究与AI和机器人技术(需要物理场景理解)和脑机界面(需要了解相关神经代码)直接相关。对于更广泛的研究社区,该项目将a)开发公共数据集,基准任务和挑战,b)主持对抗性协作以应对这些挑战,c)c)c)c)将从心理学到AI到AI到AI到神经科学的神经科学的研究社区的跨学科研讨会,以解决这些领域的基本问题,以反映了这些奖项,以反映NSF的基本问题。和更广泛的影响审查标准。
项目成果
期刊论文数量(1)
专著数量(0)
科研奖励数量(0)
会议论文数量(0)
专利数量(0)
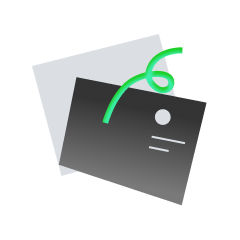
暂无数据
数据更新时间:2024-06-01
Nancy Kanwisher其他文献
Repetition blindness and illusory conjunctions: errors in binding visual types with visual tokens.
- DOI:10.1037//0096-1523.17.2.40410.1037//0096-1523.17.2.404
- 发表时间:1991-051991-05
- 期刊:
- 影响因子:0
- 作者:Nancy KanwisherNancy Kanwisher
- 通讯作者:Nancy KanwisherNancy Kanwisher
Fine-grained neural coding of bodies and body parts in human visual cortex
人类视觉皮层身体和身体部位的细粒度神经编码
- DOI:
- 发表时间:20242024
- 期刊:
- 影响因子:0
- 作者:Jesus Garcia Ramirez;Michaël Vanhoyland;Ratan N. Apurva Murty;T. Decramer;W. Van Paesschen;Stefania Bracci;H. Op De Beeck;Nancy Kanwisher;Peter Janssen;T. TheysJesus Garcia Ramirez;Michaël Vanhoyland;Ratan N. Apurva Murty;T. Decramer;W. Van Paesschen;Stefania Bracci;H. Op De Beeck;Nancy Kanwisher;Peter Janssen;T. Theys
- 通讯作者:T. TheysT. Theys
A module for the visual representation of faces
- DOI:10.1016/s1053-8119(96)80363-610.1016/s1053-8119(96)80363-6
- 发表时间:1996-06-011996-06-01
- 期刊:
- 影响因子:
- 作者:Nancy Kanwisher;Josh McDermott;Marvin M. ChunNancy Kanwisher;Josh McDermott;Marvin M. Chun
- 通讯作者:Marvin M. ChunMarvin M. Chun
MIT Open Access Articles An integrative computational architecture for object-driven cortex
麻省理工学院开放获取文章对象驱动皮层的综合计算架构
- DOI:
- 发表时间:
- 期刊:
- 影响因子:0
- 作者:Ilker Yildirim;Jiajun Wu;Nancy Kanwisher;Joshua B. TenenbaumIlker Yildirim;Jiajun Wu;Nancy Kanwisher;Joshua B. Tenenbaum
- 通讯作者:Joshua B. TenenbaumJoshua B. Tenenbaum
Privileged representational axes in biological and artificial neural networks
生物和人工神经网络中的特权表示轴
- DOI:
- 发表时间:20242024
- 期刊:
- 影响因子:0
- 作者:Meenakshi Khosla;Alex Williams;Josh McDermott;Nancy KanwisherMeenakshi Khosla;Alex Williams;Josh McDermott;Nancy Kanwisher
- 通讯作者:Nancy KanwisherNancy Kanwisher
共 5 条
- 1
Nancy Kanwisher的其他基金
Doctoral Dissertation Research in DRMS: Boundedly optimal sampling for decisions under uncertainty
DRMS 博士论文研究:不确定性下决策的有限最优抽样
- 批准号:08504140850414
- 财政年份:2009
- 资助金额:$ 225万$ 225万
- 项目类别:Standard GrantStandard Grant
Attention and Performance Meeting to be held July 1-7, 2002 in Erice, Sicily
注意力和绩效会议将于 2002 年 7 月 1 日至 7 日在西西里岛埃里切举行
- 批准号:02018980201898
- 财政年份:2002
- 资助金额:$ 225万$ 225万
- 项目类别:Standard GrantStandard Grant
相似国自然基金
多功能Bio-Ag NCs负载miR-200c靶向治疗耐药乳腺癌的研究
- 批准号:81871474
- 批准年份:2018
- 资助金额:25.0 万元
- 项目类别:面上项目
养老设施无障碍环境的色彩设计理论与方法研究
- 批准号:51808382
- 批准年份:2018
- 资助金额:25.0 万元
- 项目类别:青年科学基金项目
具有超高分辨率的M-NCs/M-NPs A/SAM/MEA结构光寻址生化传感器及其生化量检测研究
- 批准号:61471207
- 批准年份:2014
- 资助金额:81.0 万元
- 项目类别:面上项目
大肠杆菌胞嘧碱通透酶CodB结构和功能的研究
- 批准号:31300618
- 批准年份:2013
- 资助金额:22.0 万元
- 项目类别:青年科学基金项目
铝通过Ca2+敏感的通路抑制Ⅰ组mGluR依赖LTD机制的研究
- 批准号:30972512
- 批准年份:2009
- 资助金额:33.0 万元
- 项目类别:面上项目
相似海外基金
Collaborative Research: NCS-FR: Individual variability in auditory learning characterized using multi-scale and multi-modal physiology and neuromodulation
合作研究:NCS-FR:利用多尺度、多模式生理学和神经调节表征听觉学习的个体差异
- 批准号:24096522409652
- 财政年份:2024
- 资助金额:$ 225万$ 225万
- 项目类别:Standard GrantStandard Grant
Collaborative Research: NCS-FR: DEJA-VU: Design of Joint 3D Solid-State Learning Machines for Various Cognitive Use-Cases
合作研究:NCS-FR:DEJA-VU:针对各种认知用例的联合 3D 固态学习机设计
- 批准号:23196192319619
- 财政年份:2023
- 资助金额:$ 225万$ 225万
- 项目类别:Continuing GrantContinuing Grant
Collaborative Research: NCS-FO: Modified two-photon microscope with high-speed electrowetting array for imaging voltage transients in cerebellar molecular layer interneurons
合作研究:NCS-FO:带有高速电润湿阵列的改良双光子显微镜,用于对小脑分子层中间神经元的电压瞬变进行成像
- 批准号:23194062319406
- 财政年份:2023
- 资助金额:$ 225万$ 225万
- 项目类别:Continuing GrantContinuing Grant
Collaborative Research: NCS-FO: Dynamic Brain Graph Mining
合作研究:NCS-FO:动态脑图挖掘
- 批准号:23194502319450
- 财政年份:2023
- 资助金额:$ 225万$ 225万
- 项目类别:Continuing GrantContinuing Grant
Collaborative Research: NCS-FO: Dynamic Brain Graph Mining
合作研究:NCS-FO:动态脑图挖掘
- 批准号:23194512319451
- 财政年份:2023
- 资助金额:$ 225万$ 225万
- 项目类别:Standard GrantStandard Grant