RAPID: Computational Modeling of Contact Density and Outbreak Estimation for COVID-19 Using Large-scale Geolocation Data from Mobile Devices
RAPID:使用来自移动设备的大规模地理位置数据进行接触密度计算建模和 COVID-19 爆发估计
基本信息
- 批准号:2028687
- 负责人:
- 金额:$ 20万
- 依托单位:
- 依托单位国家:美国
- 项目类别:Standard Grant
- 财政年份:2020
- 资助国家:美国
- 起止时间:2020-05-01 至 2021-10-31
- 项目状态:已结题
- 来源:
- 关键词:
项目摘要
The outbreak of COVID-19 has highlighted both the growing global risk of emerging pandemics and the urgent need for enhanced data-driven tools to identify, contain, and mitigate their effects, particularly in dense urban areas. There has been increasing attention given to locational data from smartphones as a way to enhance epidemiological modeling and predict outbreak progression, transmission, and exposure risk. When combined with artificial intelligence or machine learning algorithms, these high resolution data have the potential to vastly improve the granularity and precision of infection and hospitalization estimates. However, the use of locational data raises serious social, ethical, and technical challenges. Trade-offs between the potential public health benefits and the impacts for privacy and civil liberties have started to be debated in earnest within the context of the current pandemic, especially in light of increasing use of these data by private companies to promote targeted advertisements, evaluate retail consumer behavior, and model travel demand, among other applications. Furthermore, the use of these data in the public interest is undermined by an incomplete understanding of the representativeness and bias embedded in these data, particularly in relation to under-represented and vulnerable communities. What is not yet known is the extent of this bias in locational data and how the public health benefits of using these data diminish with spatial and temporal aggregation, which could help to minimize privacy concerns in the collection and use of these data. To address these questions, this project will develop computational models derived from large-scale locational data to (1) estimate the exposure density across a range of temporal (hourly, daily, etc.) and spatial (census block, neighborhood, etc.) scales, which will enable officials and researchers to evaluate and predict transmission rates in a particular area; (2) measure and evaluate the extent and effectiveness of social (physical) distancing efforts over time and comparatively within and across counties and cities, as well as understand the disparate impacts on vulnerable communities and populations; and (3) measure the extent of disease spread based on movement and travel patterns between neighborhoods and communities, which will support predictions of the spatial-temporal patterns of disease outbreak and identify “at-risk” locations based on the aggregated mobility trajectories for areas were infections have been identified or suspected. The project team is particularly concerned with how shelter-in-place orders and exposure risk disproportionately impact low-income and minority communities, and the implications of potential bias in locational data in assessing socioeconomic variations. The project will assess how the usefulness of these models for epidemiologists and public health officials varies with spatial aggregation (e.g. is neighborhood level data superior to county level data) and temporal aggregation (e.g. is a near-real-time model superior to daily or weekly timescales) and provide quantitative performance assessments that can be used for collective decision-making on the trade-offs between health benefits and privacy risk. Project outputs will be made open-source and publicly available as appropriate.This award reflects NSF's statutory mission and has been deemed worthy of support through evaluation using the Foundation's intellectual merit and broader impacts review criteria.
Covid-19的爆发强调了全球出现的大流行病的日益增长的风险,以及迫切需要增强数据驱动的工具以识别,遏制和减轻其影响,尤其是在密集的城市地区。越来越多的关注智能手机的位置数据是一种增强流行病学建模并预测爆发进展,传播和暴露风险的方法。当与人工智能或机器学习算法结合使用时,这些高分辨率数据有可能大大提高感染和住院估计的粒度和精度。但是,使用位置数据会带来严重的社会,道德和技术挑战。潜在的公共卫生福利与隐私的影响与公民自由之间的权衡已经开始认真辩论,尤其是鉴于私营公司越来越多地使用这些数据来促进目标广告,评估零售消费者的行为,并建模旅行需求以及其他应用程序。此外,由于对这些数据中嵌入的代表性和偏见的不完全理解,特别是与代表性不足和代表性不足和脆弱的社区有关的代表性和偏见,因此破坏了这些数据的使用。尚不清楚的是该位置数据中这种偏见的程度,以及使用这些数据的公共卫生益处如何减少空间和临时聚合,这可以帮助最大程度地减少收集和使用这些数据的隐私问题。为了解决这些问题,该项目将开发从大规模位置数据得出的计算模型,以估算一系列临时(小时,每日等)和空间(人口普查,邻里等)量表的暴露密度,这将使官员和研究人员能够评估特定领域的传播率和预测特定领域的传播率; (2)衡量和评估社会(物理)疏远的范围和有效性,随着时间的流逝,县内和整个城市内和整个城市,以及了解对弱势社区和人群的不同影响; (3)根据社区和社区之间的运动和旅行模式来衡量疾病的扩散程度,这将支持对疾病暴发的空间周期性模式的预测,并根据感染的区域的综合移动性轨迹确定“处于危险的”位置。项目团队特别关注现场订单和暴露风险如何不成比例地影响低收入和少数民族社区,以及潜在偏见在评估社会经济变化中的潜在偏见的影响。该项目将评估这些模型对流行病学家和公共卫生官员的有用性如何随空间聚集而变化(例如,邻里级别的数据优于县级数据)和临时聚集(例如,近乎现实的模型优于每日或每周的时间标准),并可以将其定量绩效评估用于整体决策,从而可以将其用于损害健康和损害健康的损害和损害健康的效果和损害。该奖项将由NSF的法定任务进行开放源代码,并适当公开提供。该奖项被认为是值得通过基金会的知识分子优点和更广泛影响的评论标准来评估的。
项目成果
期刊论文数量(1)
专著数量(0)
科研奖励数量(0)
会议论文数量(0)
专利数量(0)
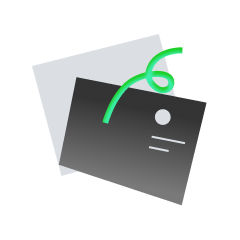
暂无数据
数据更新时间:2024-06-01
Constantine Kontok...的其他基金
AI-DCL: EAGER: Bias and Discrimination in City Predictive Analytics
AI-DCL:EAGER:城市预测分析中的偏见和歧视
- 批准号:19264701926470
- 财政年份:2019
- 资助金额:$ 20万$ 20万
- 项目类别:Standard GrantStandard Grant
CAREER: Urban Informatics for Smart, Sustainable Cities: Toward a Data-Driven Understanding of Metropolitan Energy Dynamics
职业:智慧、可持续城市的城市信息学:以数据驱动的方式理解大都市能源动态
- 批准号:16537721653772
- 财政年份:2017
- 资助金额:$ 20万$ 20万
- 项目类别:Standard GrantStandard Grant
相似国自然基金
基于知识重用的车身自动几何建模方法研究
- 批准号:51905389
- 批准年份:2019
- 资助金额:19.0 万元
- 项目类别:青年科学基金项目
有理插值算子、保凸插值的深入研究
- 批准号:61772025
- 批准年份:2017
- 资助金额:50.0 万元
- 项目类别:面上项目
适用于复杂外形产品的等几何造型理论及新型分析方法研究
- 批准号:61472111
- 批准年份:2014
- 资助金额:80.0 万元
- 项目类别:面上项目
半序微结构多孔模型双尺度设计优化
- 批准号:61472356
- 批准年份:2014
- 资助金额:84.0 万元
- 项目类别:面上项目
基于拓扑结构分析的实体特征造型理论与方法
- 批准号:61402249
- 批准年份:2014
- 资助金额:26.0 万元
- 项目类别:青年科学基金项目
相似海外基金
Collaborative Research: RAPID: Rapid computational modeling of wildfires and management with emphasis on human activity
合作研究:RAPID:野火和管理的快速计算建模,重点关注人类活动
- 批准号:23452562345256
- 财政年份:2023
- 资助金额:$ 20万$ 20万
- 项目类别:Standard GrantStandard Grant
CRCNS: Acetylcholine and state-dependent neural network reorganization
CRCNS:乙酰胆碱和状态依赖的神经网络重组
- 批准号:1083005010830050
- 财政年份:2023
- 资助金额:$ 20万$ 20万
- 项目类别:
Elucidating Non-Routine Events Arising from Interhospital Transfers
阐明院间转移引起的非常规事件
- 批准号:1074944810749448
- 财政年份:2023
- 资助金额:$ 20万$ 20万
- 项目类别:
Novel bioinformatics methods to detect DNA and RNA modifications using Nanopore long-read sequencing
使用 Nanopore 长读长测序检测 DNA 和 RNA 修饰的新型生物信息学方法
- 批准号:1079241610792416
- 财政年份:2023
- 资助金额:$ 20万$ 20万
- 项目类别:
Developing a computational platform for induced-fit and chemogenetic drug design
开发诱导拟合和化学遗传学药物设计的计算平台
- 批准号:1068074510680745
- 财政年份:2023
- 资助金额:$ 20万$ 20万
- 项目类别: