面向变压器寿命评价的绕组结构动态特征参数识别方法
项目介绍
AI项目解读
基本信息
- 批准号:51805504
- 项目类别:青年科学基金项目
- 资助金额:25.0万
- 负责人:
- 依托单位:
- 学科分类:E0503.机械动力学
- 结题年份:2021
- 批准年份:2018
- 项目状态:已结题
- 起止时间:2019-01-01 至2021-12-31
- 项目参与者:徐红伟; 富雅琼; 王圣彬; 章俊涛; 陈兵役;
- 关键词:
项目摘要
The operational safety of power transformers is very important for the electrical power networks, and effective condition detection methods of in-service transformers are the key to ensure the reliable operation of the system. Windings are the most important and vulnerable components in transformers, however, the lack of effective live detection method is an urgent problem to be solved. The previous studies show that the transformer vibration is related to the internal structure, and the vibration characteristics of transformers under different conditions have statistical regularity. Unlike the off-line winding structural model, the winding unit of an in-service transformer is represented by a dynamic system varying with the load in this study. The dynamic feature parameters of the winding structures are identified by extracting the response characteristics of different excitation sources from the mixed vibration. The main contents include: (1) Separation of mixed vibration signals by means of signal decomposition reconstruction and vector relation model; (2) A "load-structure" coupled winding model is established, and the relationship between the dynamic structural parameters and the load is analyzed; (3) The dynamic response characteristics of winding structure under different excitations are studied, and the related feature extraction methods are obtained; (4) A winding condition decision model based on multi-dimensional vibration features is established, and the transformer lifetime evaluation method is proposed through analyzing the vibration trend in the process of winding structure degradation. This research work provides technical support for the condition detection and intelligent diagnosis of in-service transformers.
变压器的安全运行对电力系统而言至关重要,对运行变压器实施有效的状态检测是保证系统可靠运行的关键。绕组是变压器内部既重要又容易发生故障的部件,然而缺乏有效的带电检测方法是亟待解决的问题。前期研究表明变压器振动与内部结构相关,且不同工况下变压器振动特征具有统计规律性。不同于离线状态的绕组结构模型,本研究将运行变压器的绕组等效为随负载变化的动态系统,并通过提取混合振动信号中不同激励源对应的响应特征实现绕组结构动态特征参数识别。主要研究内容包括:(1)利用信号分解重构和矢量关系模型分离混合振动信号;(2)建立“负载-结构”耦合的绕组数学模型,分析动态结构参数与负载的关系;(3)研究不同激励对应的绕组结构动态响应特征及相应特征提取方法;(4)建立基于多维振动特征的绕组状态决策模型,研究绕组结构衰退过程中振动变化趋势,提出变压器寿命评价方法。上述研究为在役变压器状态检测和智能诊断提供技术支撑。
结项摘要
变压器是电力系统中的关键部件。振动分析法作为一种非接触式测量方法,能对变压器机械结构进行在线监测。以压紧力为代表的运行变压器绕组机械结构寿命评价是目前工程研究的难点问题。本研究从分析绕组绝缘材料非线性力学特性入手,通过引入Rayleigh阻尼,建立阻尼比与频率的关系。并利用变分模态分解(VMD)和经验小波变换(EWT)从绕组结构下电或冲击过程的自由振动中提取不同频率对应的阻尼比。结果表明结构阻尼比包含绕组在不同压紧力下的非线性特征。研究负载电流对绕组振动响应的影响对运行变压器故障诊断意义重大。在负载-结构耦合的绕组模型研究中,利用Abaqus建立绕组结构非线性有限元模型,研究负载电流对结构模态及响应的影响。在仿真基础上,搭建测试平台并获取运行绕组模态参数。实验结果与有限元仿真结果一致。结果均表明压紧力正常绕组的结构参数几乎不受负载电流的影响。相比之下,松动绕组的固有频率会随电流的增大而增大,且电流所致的固有频率变化是绕组压紧力评价的重要指标。在上述模型基础上,提出一种基于振动图像和深度学习的绕组状态评价方法。该方法根据振动和电流趋势数据构造振动灰度图像,并结合卷积神经网络(CNN)对样本进行训练和识别。利用上述理论,对现场100多台不同状态下的变压器进行分析。结果表明绕组状态越好,灰度图像的像素值越小。相比传统单一维度的振动特征,本研究提出的方法能准确识别正常、老化和异常绕组结构,且可达到98.3%的分类准确率。在基于结构非线性特征的绕组寿命评价方法研究中,利用交叉递归图分析法提取电流与基频振动之间的非线性特征,并利用隐马尔科夫模型(HMM)构建不同负载下的非线性特征序列与压紧力衰退过程的关系。结果表明非线性特征能准确预测绕组结构的压紧力状态。将振动分析法作为常规绕组变形检测的辅助手段,对变压器内部结构状态进行跟踪,减少停役维护时间,具有很大的经济和社会效益。
项目成果
期刊论文数量(6)
专著数量(0)
科研奖励数量(0)
会议论文数量(0)
专利数量(2)
Transformer Winding Fault Diagnosis Using Vibration Image and Deep Learning
使用振动图像和深度学习进行变压器绕组故障诊断
- DOI:10.1109/tpwrd.2020.2988820
- 发表时间:2021-04-01
- 期刊:IEEE TRANSACTIONS ON POWER DELIVERY
- 影响因子:4.4
- 作者:Hong, Kaixing;Jin, Ming;Huang, Hai
- 通讯作者:Huang, Hai
Transformer Condition Monitoring Based on Load-Varied Vibration Response and GRU Neural Networks
基于变负载振动响应和 GRU 神经网络的变压器状态监测
- DOI:10.1109/access.2020.3027834
- 发表时间:2020-01-01
- 期刊:IEEE ACCESS
- 影响因子:3.9
- 作者:Hong, Kaixing;Pan, Jie;Jin, Ming
- 通讯作者:Jin, Ming
基于经验小波变换的变压器绕组结构衰退特征提取方法
- DOI:10.13465/j.cnki.jvs.2019.14.013
- 发表时间:2019
- 期刊:振动与冲击
- 影响因子:--
- 作者:洪凯星;章俊涛;许素安;黄海
- 通讯作者:黄海
A Variational Mode Decomposition Approach for Degradation Assessment of Power Transformer Windings
电力变压器绕组退化评估的变分模态分解方法
- DOI:10.1109/tim.2018.2865048
- 发表时间:2019-04-01
- 期刊:IEEE TRANSACTIONS ON INSTRUMENTATION AND MEASUREMENT
- 影响因子:5.6
- 作者:Hong, Kaixing;Wang, Ling;Xu, Suan
- 通讯作者:Xu, Suan
A Study of Nonlinear Behavior of Transformer Windings in Structural Degradation Detection
结构退化检测中变压器绕组非线性行为的研究
- DOI:10.1115/1.4045382
- 发表时间:2020-02
- 期刊:Journal of Vibration and Acoustics-Transactions of the ASME
- 影响因子:1.7
- 作者:Hong Kaixing;Huang Xueqian;Xu Suan
- 通讯作者:Xu Suan
数据更新时间:{{ journalArticles.updateTime }}
{{
item.title }}
{{ item.translation_title }}
- DOI:{{ item.doi || "--"}}
- 发表时间:{{ item.publish_year || "--" }}
- 期刊:{{ item.journal_name }}
- 影响因子:{{ item.factor || "--"}}
- 作者:{{ item.authors }}
- 通讯作者:{{ item.author }}
数据更新时间:{{ journalArticles.updateTime }}
{{ item.title }}
- 作者:{{ item.authors }}
数据更新时间:{{ monograph.updateTime }}
{{ item.title }}
- 作者:{{ item.authors }}
数据更新时间:{{ sciAawards.updateTime }}
{{ item.title }}
- 作者:{{ item.authors }}
数据更新时间:{{ conferencePapers.updateTime }}
{{ item.title }}
- 作者:{{ item.authors }}
数据更新时间:{{ patent.updateTime }}
其他文献
其他文献
{{
item.title }}
{{ item.translation_title }}
- DOI:{{ item.doi || "--" }}
- 发表时间:{{ item.publish_year || "--"}}
- 期刊:{{ item.journal_name }}
- 影响因子:{{ item.factor || "--" }}
- 作者:{{ item.authors }}
- 通讯作者:{{ item.author }}
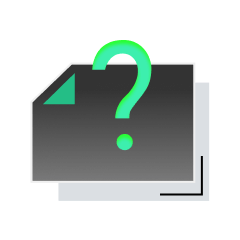
内容获取失败,请点击重试
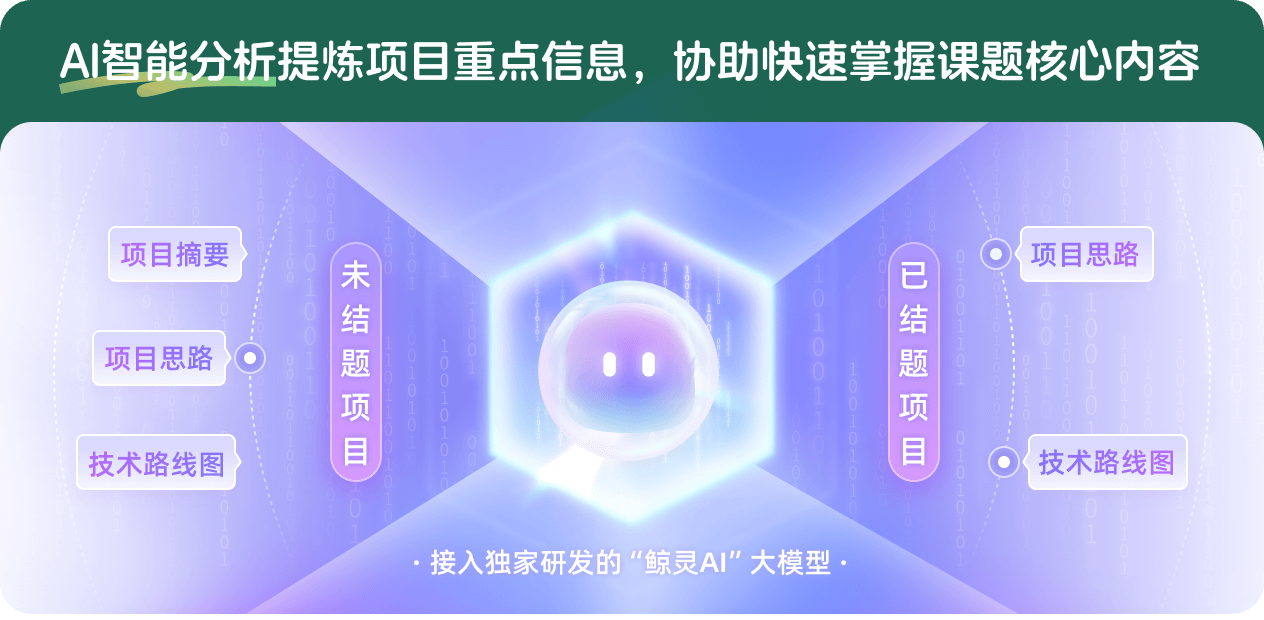
查看分析示例
此项目为已结题,我已根据课题信息分析并撰写以下内容,帮您拓宽课题思路:
AI项目摘要
AI项目思路
AI技术路线图
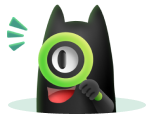
请为本次AI项目解读的内容对您的实用性打分
非常不实用
非常实用
1
2
3
4
5
6
7
8
9
10
您认为此功能如何分析更能满足您的需求,请填写您的反馈:
相似国自然基金
{{ item.name }}
- 批准号:{{ item.ratify_no }}
- 批准年份:{{ item.approval_year }}
- 资助金额:{{ item.support_num }}
- 项目类别:{{ item.project_type }}
相似海外基金
{{
item.name }}
{{ item.translate_name }}
- 批准号:{{ item.ratify_no }}
- 财政年份:{{ item.approval_year }}
- 资助金额:{{ item.support_num }}
- 项目类别:{{ item.project_type }}