基于级数估计的非参数和半参数股票收益预测模型研究
项目介绍
AI项目解读
基本信息
- 批准号:71703045
- 项目类别:青年科学基金项目
- 资助金额:20.0万
- 负责人:
- 依托单位:
- 学科分类:G0301.计量经济与经济统计
- 结题年份:2020
- 批准年份:2017
- 项目状态:已结题
- 起止时间:2018-01-01 至2020-12-31
- 项目参与者:王少平; 颜诚; 陈力; 周炜伦; 艾娣; 黎娇龙; 邹建文;
- 关键词:
项目摘要
The predictability of stock returns is one of the most important problems in finance and is one of the current focus of the researches in econometrics and finance. The integrable nonlinear cointegrating regression model was shown to catch the characteristics of the data structure in stock return predictive regression where the predicting variables are unit roots or nearly unit roots while the predicted return is a nearly white noise process, thus is more suitable in modeling the predictive regression than the widely used linear model. This project examines univariate nonparametric cointegrating regression model and multivariate semiparametric cointegrating regression models based on series estimation which is a huge progress in the theoretical reseraches in nonlinear nonstationary time series. At the same time, we will examine whether the proposed models can out-perform the traditional linear model and historic mean (no predictability) model which will be of great interests for the nonlinear stock return predictability researches.
股票收益的可预测性是金融学中最重要的问题之一,也是当前计量经济学和金融学研究的热点问题之一。股票收益预测回归模型的一个重要数据特征是:预测变量为单位根或接近单位根过程然而被预测的股票收益是个接近白噪声的过程。这说明非线性的可积协整回归模型比文献中广泛使用的线性模型更适合用来对预测回归模型建模。本项目考察基于级数估计的单变量的非参数协整回归模型和多变量的半参数协整回归模型。这是对当前非线性非平稳时间序列计量理论的一个突破。同时我们将分析提出的模型是否在样本外预测表现优于传统的线性模型和历史均值(无预测性)模型。这将对非线性的股票收益预测研究具有重要的启发意义。
结项摘要
本项目聚焦于非线性非平稳预测回归模型研究,将基于可积类协整回归模型的估计与检验方法用于股市收益预测这一金融学经典问题。我们指出,考虑到预测回归模型的特征(低持续性的被解释变量和高度持续性的解释变量),可积协整模型在预测回归分析中有广泛的应用性。 .我们考察了可积协整模型中被解释变量的一些主要的时间序列特征;提出了基于Hermite级数的Wald类检验统计量,证明其渐近分布为卡方分布,自由度为级数个数;我们也提出了用Hermite级数估计可积类协整回归模型,证明该估计量的渐近正态性。.通过利用基于Hermite级数的Wald类检验统计量,我们发现美国股市中,股息率,账面市值比率和收益价格比等三个常用预测变量对股票收益具有样本内预测能力。此外,我们发现股息率具有样本外预测能力而其它两个变量未具有样本外预测能力。但是在中国股市中,这三个变量均不具备样本外预测能力。
项目成果
期刊论文数量(4)
专著数量(1)
科研奖励数量(0)
会议论文数量(0)
专利数量(0)
Modelling fundamental analysis in portfolio selection
投资组合选择中的基本面分析建模
- DOI:10.1080/14697688.2017.1418520
- 发表时间:2018-02
- 期刊:Quantitative Finance
- 影响因子:1.3
- 作者:Cheng Yan
- 通讯作者:Cheng Yan
Hot money in disaggregated capital flows
分类资本流动中的热钱
- DOI:10.1080/1351847x.2017.1411821
- 发表时间:2017-11
- 期刊:The European Journal of Finance
- 影响因子:--
- 作者:Cheng Yan
- 通讯作者:Cheng Yan
Bankruptcy prediction with financial systemic risk
金融系统性风险的破产预测
- DOI:10.1080/1351847x.2019.1656095
- 发表时间:2019-08
- 期刊:The European Journal of Finance
- 影响因子:--
- 作者:Cheng Yan
- 通讯作者:Cheng Yan
数据更新时间:{{ journalArticles.updateTime }}
{{
item.title }}
{{ item.translation_title }}
- DOI:{{ item.doi || "--"}}
- 发表时间:{{ item.publish_year || "--" }}
- 期刊:{{ item.journal_name }}
- 影响因子:{{ item.factor || "--"}}
- 作者:{{ item.authors }}
- 通讯作者:{{ item.author }}
数据更新时间:{{ journalArticles.updateTime }}
{{ item.title }}
- 作者:{{ item.authors }}
数据更新时间:{{ monograph.updateTime }}
{{ item.title }}
- 作者:{{ item.authors }}
数据更新时间:{{ sciAawards.updateTime }}
{{ item.title }}
- 作者:{{ item.authors }}
数据更新时间:{{ conferencePapers.updateTime }}
{{ item.title }}
- 作者:{{ item.authors }}
数据更新时间:{{ patent.updateTime }}
其他文献
其他文献
{{
item.title }}
{{ item.translation_title }}
- DOI:{{ item.doi || "--" }}
- 发表时间:{{ item.publish_year || "--"}}
- 期刊:{{ item.journal_name }}
- 影响因子:{{ item.factor || "--" }}
- 作者:{{ item.authors }}
- 通讯作者:{{ item.author }}
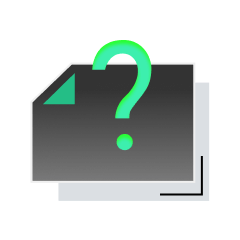
内容获取失败,请点击重试
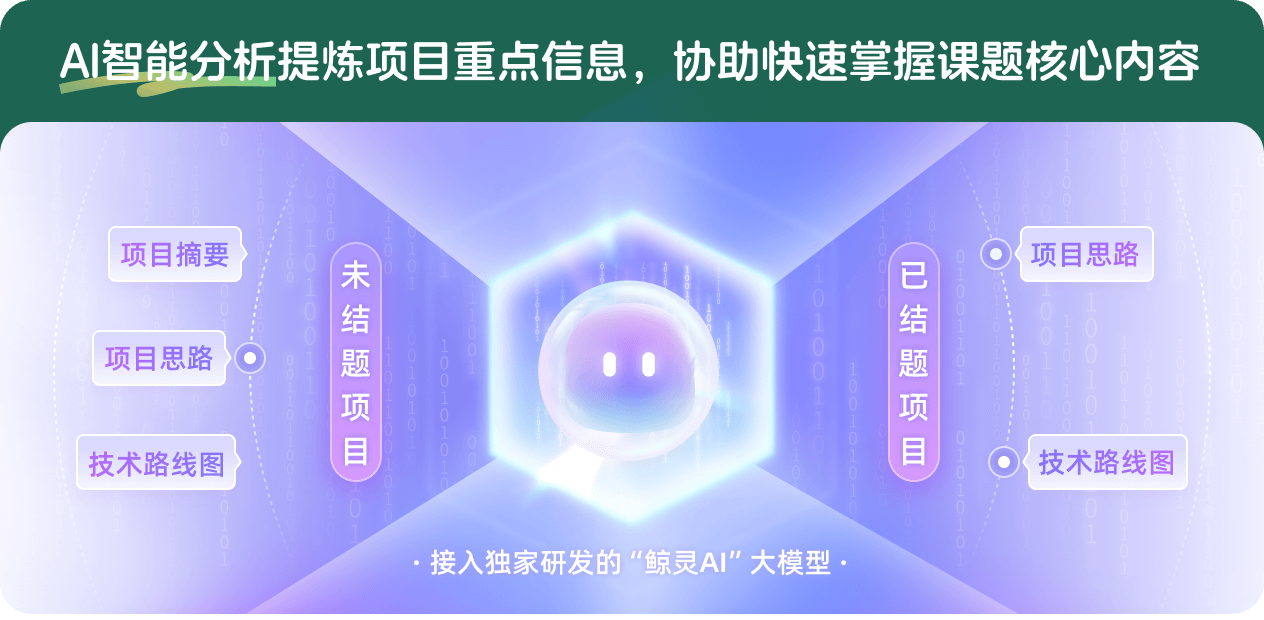
查看分析示例
此项目为已结题,我已根据课题信息分析并撰写以下内容,帮您拓宽课题思路:
AI项目摘要
AI项目思路
AI技术路线图
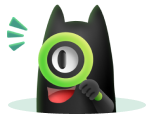
请为本次AI项目解读的内容对您的实用性打分
非常不实用
非常实用
1
2
3
4
5
6
7
8
9
10
您认为此功能如何分析更能满足您的需求,请填写您的反馈:
相似国自然基金
{{ item.name }}
- 批准号:{{ item.ratify_no }}
- 批准年份:{{ item.approval_year }}
- 资助金额:{{ item.support_num }}
- 项目类别:{{ item.project_type }}
相似海外基金
{{
item.name }}
{{ item.translate_name }}
- 批准号:{{ item.ratify_no }}
- 财政年份:{{ item.approval_year }}
- 资助金额:{{ item.support_num }}
- 项目类别:{{ item.project_type }}