基于烷基酰胺化衍生反应和链接组合数据库搜索的唾液酸链接特异N-糖蛋白质组学的研发与应用研究
项目介绍
AI项目解读
基本信息
- 批准号:
- 项目类别:面上项目
- 资助金额:65万
- 负责人:
- 依托单位:
- 学科分类:
- 结题年份:
- 批准年份:2020
- 项目状态:未结题
- 起止时间:2020至
- 项目参与者:田志新;
- 关键词:
项目摘要
结项摘要
项目成果
期刊论文数量(0)
专著数量(0)
科研奖励数量(0)
会议论文数量(0)
专利数量(0)
数据更新时间:{{ journalArticles.updateTime }}
{{
item.title }}
{{ item.translation_title }}
- DOI:{{ item.doi || "--"}}
- 发表时间:{{ item.publish_year || "--" }}
- 期刊:{{ item.journal_name }}
- 影响因子:{{ item.factor || "--"}}
- 作者:{{ item.authors }}
- 通讯作者:{{ item.author }}
数据更新时间:{{ journalArticles.updateTime }}
{{ item.title }}
- 作者:{{ item.authors }}
数据更新时间:{{ monograph.updateTime }}
{{ item.title }}
- 作者:{{ item.authors }}
数据更新时间:{{ sciAawards.updateTime }}
{{ item.title }}
- 作者:{{ item.authors }}
数据更新时间:{{ conferencePapers.updateTime }}
{{ item.title }}
- 作者:{{ item.authors }}
数据更新时间:{{ patent.updateTime }}
其他文献
Analysis device and method for identifying biomacromolecules
生物大分子鉴定的分析装置及方法
- DOI:--
- 发表时间:2012
- 期刊:
- 影响因子:--
- 作者:田志新
- 通讯作者:田志新
Structure for manyfold increasing analysis flux of high performance liquid chromatograph
高效液相色谱仪分析通量倍增结构
- DOI:--
- 发表时间:2012
- 期刊:
- 影响因子:--
- 作者:田志新
- 通讯作者:田志新
整体蛋白质电喷雾质谱原位解析
- DOI:10.13488/j.smhx.20170103
- 发表时间:2017
- 期刊:生命的化学
- 影响因子:--
- 作者:肖开捷;田志新
- 通讯作者:田志新
混沌空间光通信研究进展
- DOI:10.3788/irla20220296
- 发表时间:2023
- 期刊:红外与激光工程
- 影响因子:--
- 作者:王超;董怡泽;王卉婷;高冀;田志新;高建威;江宁
- 通讯作者:江宁
其他文献
{{
item.title }}
{{ item.translation_title }}
- DOI:{{ item.doi || "--" }}
- 发表时间:{{ item.publish_year || "--"}}
- 期刊:{{ item.journal_name }}
- 影响因子:{{ item.factor || "--" }}
- 作者:{{ item.authors }}
- 通讯作者:{{ item.author }}
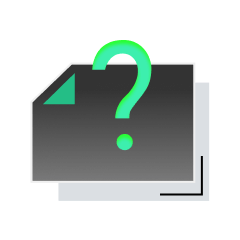
内容获取失败,请点击重试
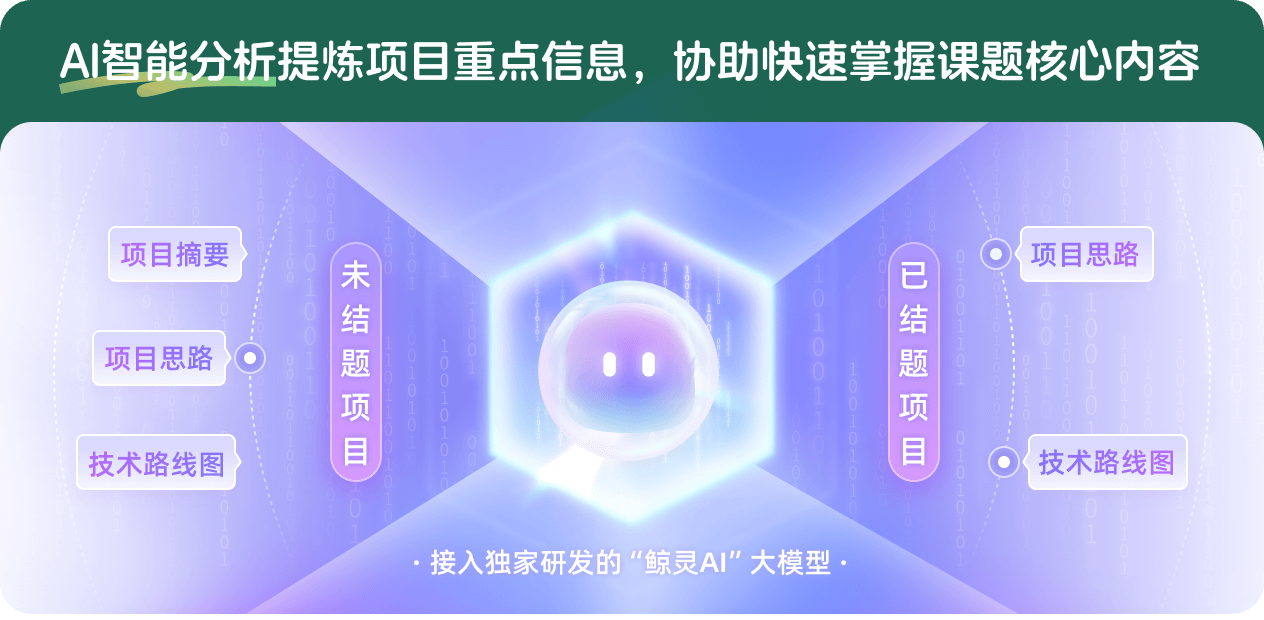
查看分析示例
此项目为未结题,我已根据课题信息分析并撰写以下内容,帮您拓宽课题思路:
AI项目摘要
AI项目思路
AI技术路线图
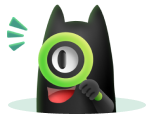
请为本次AI项目解读的内容对您的实用性打分
非常不实用
非常实用
1
2
3
4
5
6
7
8
9
10
您认为此功能如何分析更能满足您的需求,请填写您的反馈:
田志新的其他基金
基于近同重二甲基标记和同位素轮廓指纹比对的完整N-糖肽定性定量分析研究
- 批准号:21775110
- 批准年份:2017
- 资助金额:65.0 万元
- 项目类别:面上项目
基于同位素轮廓指纹比对和同重二甲基标记的整体蛋白质组定量研究
- 批准号:21575104
- 批准年份:2015
- 资助金额:65.0 万元
- 项目类别:面上项目
相似国自然基金
{{ item.name }}
- 批准号:{{ item.ratify_no }}
- 批准年份:{{ item.approval_year }}
- 资助金额:{{ item.support_num }}
- 项目类别:{{ item.project_type }}
相似海外基金
{{
item.name }}
{{ item.translate_name }}
- 批准号:{{ item.ratify_no }}
- 财政年份:{{ item.approval_year }}
- 资助金额:{{ item.support_num }}
- 项目类别:{{ item.project_type }}