面向活动类型挖掘和主题提取的人类行为模式研究
项目介绍
AI项目解读
基本信息
- 批准号:41801378
- 项目类别:青年科学基金项目
- 资助金额:25.2万
- 负责人:
- 依托单位:
- 学科分类:D0114.地理信息学
- 结题年份:2021
- 批准年份:2018
- 项目状态:已结题
- 起止时间:2019-01-01 至2021-12-31
- 项目参与者:李圣文; 翟雅倩; 刘宏宇; 高中贤; 张苗; 袁梦飞; 崔笛;
- 关键词:
项目摘要
The human behavior patterns mined from trajectory data depict the relationship between human behavior, social and geographical environment, and help us to reveal the law of human behavior. The previous researches failed to effectively express the fundamental relationship in human activity patterns, because they neglected individual activity types, and overly simplified the relationship among individuals, spatiotemporal activities and behavior patterns. To address these problems, this project intends to adopt social media trajectory as data sources, develop modeling methods of human behavior patterns by identifying potential activity types and extracting human activity topics. Based on the knowledge graph theory and convolutional neural network, the human activity type discrimination method combining text content and trajectory position is designed to realize the accurate identification of human activity types in the trajectory. Using spatiotemporal activity flows instead of spatiotemporal activity hotspots and sparse simplification theory, a human activity topic model combining activity types and spatiotemporal locations is established to achieve accurate expressing of human behavioral patterns. In addition, the project will conduct research on the evaluation of human activity topic model considering of spatiotemporal heterogeneity. Those research results of this project are expected to theoretically reveal the essential relationship between individual spatiotemporal activities and human behavior patterns, provide methods for identifying human activities and for extracting activity patterns, and contribute to improving of understanding, prediction and intervening of human behavior.
从轨迹数据中挖掘隐含的人类行为模式,可以刻画人类行为与社会、地理环境之间的关系,揭示人类行为规律。当前研究忽视了个体活动类型信息,过度简化了个体、时空活动、行为模式间的关系,导致难以有效表达人类活动模式中的本质关系。本课题拟以社交媒体轨迹数据为数据源,通过识别潜在的活动类型、抽取人类活动主题,对人类行为模式的精细化建模展开研究。基于知识图谱和卷积神经网络,设计兼顾文本内容与轨迹位置的人类活动类型判别方法,实现轨迹中人类活动类型的精确判别;采用用户轨迹的时空活动流代替时空活动区域,基于稀疏化简的思路,建立结合活动类型和时空位置的人类活动主题提取模型,实现人类行为规律的准确表达;并在顾及时空异质性的基础上,对人类活动主题的评价进行研究。通过本课题研究,可望在理论上揭示个体时空活动与人类行为模式间的本质关系,建立人类活动内容识别、行为模式抽象的新方法,在应用上为理解、预测和干预人类行为提供支撑。
结项摘要
从轨迹数据中挖掘隐含的人类行为模式,可以刻画人类行为与社会、地理环境之间的关系,揭示人类行为规律。本课题以社交媒体轨迹数据为数据源,通过识别潜在的活动类型、抽取人类活动主题,对人类行为模式的精细化建模展开研究。基于知识图谱和卷积神经网络,设计兼顾文本内容与轨迹位置的人类活动类型判别方法,实现轨迹中人类活动类型的精确判别;采用用户轨迹的时空活动流代替时空活动区域,基于稀疏化简的思路,建立结合活动类型和时空位置的人类活动主题提取模型,实现人类行为规律的准确表达;并在顾及时空异质性的基础上,对人类活动主题的评价进行了研究。本课题主要成果在于提出了三种人类活动识别模型,一种人类活动模式提取模型,发布了一个可用于训练和评估人类活动类型识别模型的数据集。本课题的研究成果在理论上揭示了个体时空活动与人类行为模式间的本质关系,建立了人类活动内容识别、行为模式抽象的新方法,在应用上为理解、预测和干预人类行为提供支撑。
项目成果
期刊论文数量(10)
专著数量(1)
科研奖励数量(0)
会议论文数量(0)
专利数量(2)
Toward Measuring the Level of Spatiotemporal Clustering of Multi-Categorical Geographic Events
衡量多类别地理事件的时空聚类水平
- DOI:10.3390/ijgi9070440
- 发表时间:2020-07
- 期刊:ISPRS International Journal of Geo-Information
- 影响因子:3.4
- 作者:Gong Junfang;Lee Jay;Zhou Shunping;Li Shengwen
- 通讯作者:Li Shengwen
Recognizing Human Daily Activity Using Social Media Sensors and Deep Learning
使用社交媒体传感器和深度学习识别人类日常活动
- DOI:10.3390/ijerph16203955
- 发表时间:2019-10-01
- 期刊:INTERNATIONAL JOURNAL OF ENVIRONMENTAL RESEARCH AND PUBLIC HEALTH
- 影响因子:--
- 作者:Gong, Junfang;Li, Runjia;Li, Shengwen
- 通讯作者:Li, Shengwen
Synthesizing location semantics from street view images to improve urban land-use classification
从街景图像中合成位置语义以改进城市土地利用分类
- DOI:10.1080/13658816.2020.1831515
- 发表时间:2020-10
- 期刊:International Journal of Geographical Information Science
- 影响因子:5.7
- 作者:Fang Fang;Yu Yafang;Li Shengwen;Zuo Zejun;Liu Yuanyuan;Wan Bo;Luo Zhongwen
- 通讯作者:Luo Zhongwen
Identifying human daily activity types with time-aware interactions
通过时间感知交互识别人类日常活动类型
- DOI:10.3390/app10248922
- 发表时间:2020
- 期刊:Applied Sciences
- 影响因子:--
- 作者:Renyao Chen;Hong Yao;Runjia Li;Xiaojun Kang;Lijun Dong;Shengwen Li;Junfang Gong
- 通讯作者:Junfang Gong
DAWE: A Double Attention-Based Word Embedding Model with Sememe Structure Information
DAWE:具有义原结构信息的基于双重注意力的词嵌入模型
- DOI:10.3390/app10175804
- 发表时间:2020
- 期刊:Appl. Sci.
- 影响因子:--
- 作者:Shengwen Li;Renyao Chen;Bo Wan;Junfang Gong;Lin Yang;Hong Yao
- 通讯作者:Hong Yao
数据更新时间:{{ journalArticles.updateTime }}
{{
item.title }}
{{ item.translation_title }}
- DOI:{{ item.doi || "--"}}
- 发表时间:{{ item.publish_year || "--" }}
- 期刊:{{ item.journal_name }}
- 影响因子:{{ item.factor || "--"}}
- 作者:{{ item.authors }}
- 通讯作者:{{ item.author }}
数据更新时间:{{ journalArticles.updateTime }}
{{ item.title }}
- 作者:{{ item.authors }}
数据更新时间:{{ monograph.updateTime }}
{{ item.title }}
- 作者:{{ item.authors }}
数据更新时间:{{ sciAawards.updateTime }}
{{ item.title }}
- 作者:{{ item.authors }}
数据更新时间:{{ conferencePapers.updateTime }}
{{ item.title }}
- 作者:{{ item.authors }}
数据更新时间:{{ patent.updateTime }}
其他文献
其他文献
{{
item.title }}
{{ item.translation_title }}
- DOI:{{ item.doi || "--" }}
- 发表时间:{{ item.publish_year || "--"}}
- 期刊:{{ item.journal_name }}
- 影响因子:{{ item.factor || "--" }}
- 作者:{{ item.authors }}
- 通讯作者:{{ item.author }}
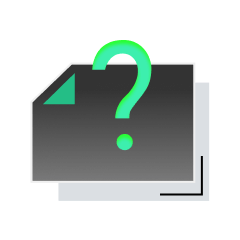
内容获取失败,请点击重试
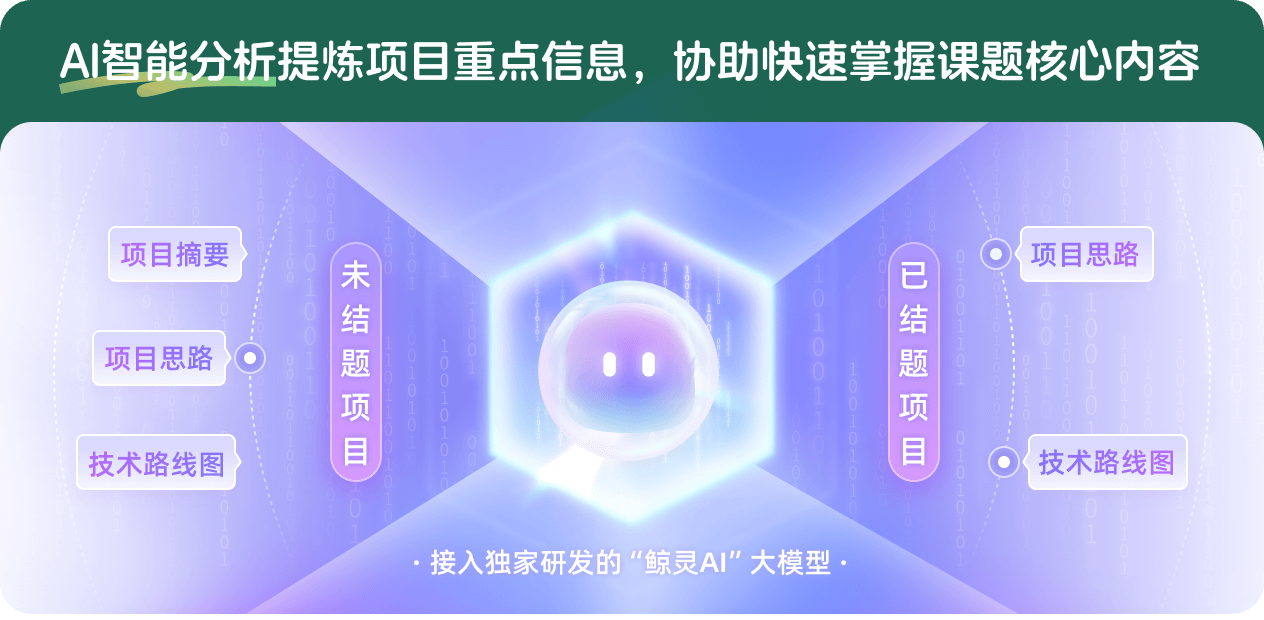
查看分析示例
此项目为已结题,我已根据课题信息分析并撰写以下内容,帮您拓宽课题思路:
AI项目摘要
AI项目思路
AI技术路线图
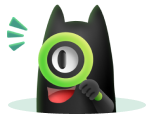
请为本次AI项目解读的内容对您的实用性打分
非常不实用
非常实用
1
2
3
4
5
6
7
8
9
10
您认为此功能如何分析更能满足您的需求,请填写您的反馈:
相似国自然基金
{{ item.name }}
- 批准号:{{ item.ratify_no }}
- 批准年份:{{ item.approval_year }}
- 资助金额:{{ item.support_num }}
- 项目类别:{{ item.project_type }}
相似海外基金
{{
item.name }}
{{ item.translate_name }}
- 批准号:{{ item.ratify_no }}
- 财政年份:{{ item.approval_year }}
- 资助金额:{{ item.support_num }}
- 项目类别:{{ item.project_type }}