基于Tensor Train分解的两类张量优化问题的研究及其应用
项目介绍
AI项目解读
基本信息
- 批准号:11701132
- 项目类别:青年科学基金项目
- 资助金额:25.0万
- 负责人:
- 依托单位:
- 学科分类:A0405.连续优化
- 结题年份:2020
- 批准年份:2017
- 项目状态:已结题
- 起止时间:2018-01-01 至2020-12-31
- 项目参与者:韩斌; 李婵; 闫伟杰; 张振伟;
- 关键词:
项目摘要
Tensor Train decomposition is one of the most efficient form to express high-order data. Due to the separable structure, this kind of decomposition brings great convenience for solving large-scale tensor optimization problems. In this project, based on Tensor Train decomposition, we consider two kinds of tensor optimization problems: tensor eigenvalue complementarity problem and tensor completion problem. By integrating tensor analysis (e.g. decomposition, spectral theory) and optimization techniques (e.g. duality, relaxation), we carry out the theoretical analysis and algorithm design from the perspective of alternating optimization and Riemannian optimization, respectively. The main research content includes: 1) For tensor eigenvalue complementarity problem, we analyze the properties of the solutions and propose efficient algorithms with convergence analysis by combining the alternating direction method and projection technique. 2) For tensor completion problem, we establish corresponding tensor optimization model, analyze the properties (e.g. semismooth, strongly semismooth) of objective function on the Riemannian manifold of tensors with fixed TT-ranks, and propose efficient Riemannian gradient method. 3) We apply our proposed methods to practical problems, such as MIMO system simulation and machine learning, in order to check the feasibility and efficiency of our methods.
Tensor Train分解是表示高阶数据有效的分解形式之一,其可分离的结构特点对大规模张量优化问题的求解带来极大便利。在本项目中,我们将基于Tensor Train分解,围绕张量优化中特征值互补和张量完备化这两类重要的问题展开研究,采用张量分析(如分解、谱理论等)与优化方法(如对偶、松弛等)相结合的技术,分别从交替优化和Riemannian优化的角度对所研究的问题进行理论分析和算法设计。内容包括:1)建立张量特征值互补问题解的性质,采用交替方向法和投影技术相结合思路,设计有效算法并进行收敛性分析;2)针对张量完备化问题,建立相应的张量优化模型,分析目标函数在固定张量TT秩所构成的Riemannian流形上的性质(包括半光滑、强半光滑等),并设计有效的Riemannian投影梯度型算法;3)将所提出的算法应用到MIMO系统仿真、机器学习等实际问题,进一步检验算法的可行性和有效性。
结项摘要
张量优化是最优化研究领域一个新兴研究分支,近年来,基于Tensor Train分解的优化问题的研究越来越引起人们的关注,而目前国内对相关张量优化问题的研究还处于起步阶段。在此背景下,本项目在Tensor Train分解的框架下系统研究张量特征值互补、多项式分类器等问题,同时结合实际问题的特点,设计出有效的求解方法并提供严格可靠的理论依据。在本项目的资助下,取得的主要成果如下:1)张量特征值互补问题是一类重要的非线性优化问题,在固体力学、动力系统等领域具有广泛的应用。本项目研究一类广义张量特征值互补问题,得到其解的性质,包括存在性、唯一性等。在对称情形下,研究其相应优化问题等价形式,得到互补问题解的最优性条件及可解的充分条件,并提出自适应的梯度投影算法,给出收敛性分析。2)模式识别是机器学习中一类非常重要的监督学习问题,其中多项式分类器是一类广泛使用且有效的模型之一。针对传统的多项式分类器在处理高维问题时出现的维数灾难,提出基于Tensor Train分解的学习框架,进一步结合Tensor Train分解的结构特点,提出两种快速可并行的学习算法,并在USPS和MNIST数据集上验证了算法的有效性。3)张量函数表示论是固体力学中基本理论之一,具有重要的理论和实际意义。本项目研究了三阶对称张量和四阶对称无迹张量的各向同性不可约函数基。基于Olive-Auffray整基,首次对三阶三维对称张量的各向同性不变量进行了完整刻画。针对四阶三维对称无迹张量,证明Smith-Bao整基构成不可约函数基,并给出一组新的不可约函数基,并证明其不被任何最小整基包含。
项目成果
期刊论文数量(4)
专著数量(0)
科研奖励数量(0)
会议论文数量(0)
专利数量(0)
An irreducible function basis of isotropic invariants of a third order three-dimensional symmetric tensor
三阶三维对称张量各向同性不变量的不可约函数基
- DOI:10.1063/1.5028307
- 发表时间:2018-03
- 期刊:Journal of Mathematical Physics
- 影响因子:1.3
- 作者:Zhongming Chen;Jinjie Liu;Liqun Qi;Quanshui Zheng;Wennan Zou
- 通讯作者:Wennan Zou
Two irreducible functional bases of isotropic invariants of a fourth-order three-dimensional symmetric and traceless tensor
四阶三维对称无迹张量各向同性不变量的两个不可约泛函基
- DOI:10.1177/1081286519835246
- 发表时间:2019
- 期刊:Mathematics and Mechanics of Solids
- 影响因子:2.6
- 作者:Zhongming Chen;Yannan Chen;Liqun Qi;Wennan Zou
- 通讯作者:Wennan Zou
Generalized Eigenvalue Complementarity Problem for Tensors
张量的广义特征值互补问题
- DOI:--
- 发表时间:2015-05
- 期刊:Pacific Journal of Optimization
- 影响因子:0.2
- 作者:Zhongming Chen;Qingzhi Yang;Lu Ye
- 通讯作者:Lu Ye
Parallelized Tensor Train Learning of Polynomial Classifiers
多项式分类器的并行张量训练学习
- DOI:10.1109/tnnls.2017.2771264
- 发表时间:2016-12
- 期刊:IEEE Transactions on Neural Networks and Learning Systems
- 影响因子:10.4
- 作者:Zhongming Chen;Kim Batselier;Johan A K Suykens;Ngai Wong
- 通讯作者:Ngai Wong
数据更新时间:{{ journalArticles.updateTime }}
{{
item.title }}
{{ item.translation_title }}
- DOI:{{ item.doi || "--"}}
- 发表时间:{{ item.publish_year || "--" }}
- 期刊:{{ item.journal_name }}
- 影响因子:{{ item.factor || "--"}}
- 作者:{{ item.authors }}
- 通讯作者:{{ item.author }}
数据更新时间:{{ journalArticles.updateTime }}
{{ item.title }}
- 作者:{{ item.authors }}
数据更新时间:{{ monograph.updateTime }}
{{ item.title }}
- 作者:{{ item.authors }}
数据更新时间:{{ sciAawards.updateTime }}
{{ item.title }}
- 作者:{{ item.authors }}
数据更新时间:{{ conferencePapers.updateTime }}
{{ item.title }}
- 作者:{{ item.authors }}
数据更新时间:{{ patent.updateTime }}
其他文献
基于模糊决策图与灰色关联分析的枪械设计方案评价
- DOI:--
- 发表时间:2016
- 期刊:兵工学报
- 影响因子:--
- 作者:阎艳;郝佳;陈中明;王国新;沙金龙
- 通讯作者:沙金龙
其他文献
{{
item.title }}
{{ item.translation_title }}
- DOI:{{ item.doi || "--" }}
- 发表时间:{{ item.publish_year || "--"}}
- 期刊:{{ item.journal_name }}
- 影响因子:{{ item.factor || "--" }}
- 作者:{{ item.authors }}
- 通讯作者:{{ item.author }}
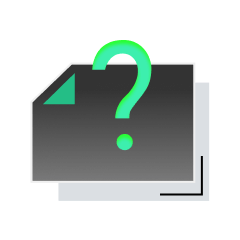
内容获取失败,请点击重试
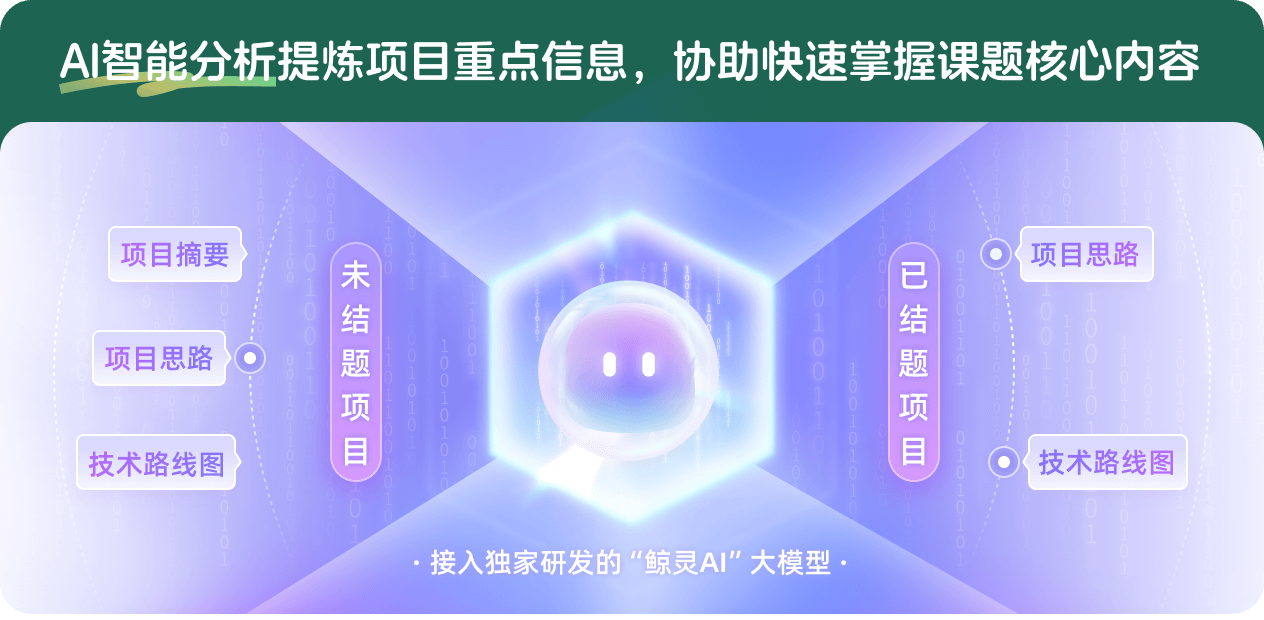
查看分析示例
此项目为已结题,我已根据课题信息分析并撰写以下内容,帮您拓宽课题思路:
AI项目摘要
AI项目思路
AI技术路线图
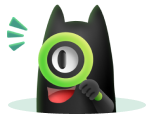
请为本次AI项目解读的内容对您的实用性打分
非常不实用
非常实用
1
2
3
4
5
6
7
8
9
10
您认为此功能如何分析更能满足您的需求,请填写您的反馈:
相似国自然基金
{{ item.name }}
- 批准号:{{ item.ratify_no }}
- 批准年份:{{ item.approval_year }}
- 资助金额:{{ item.support_num }}
- 项目类别:{{ item.project_type }}
相似海外基金
{{
item.name }}
{{ item.translate_name }}
- 批准号:{{ item.ratify_no }}
- 财政年份:{{ item.approval_year }}
- 资助金额:{{ item.support_num }}
- 项目类别:{{ item.project_type }}