面向低质量图像数据的低秩判别迁移子空间特征抽取研究
项目介绍
AI项目解读
基本信息
- 批准号:61401214
- 项目类别:青年科学基金项目
- 资助金额:25.0万
- 负责人:
- 依托单位:
- 学科分类:F0116.图像信息处理
- 结题年份:2017
- 批准年份:2014
- 项目状态:已结题
- 起止时间:2015-01-01 至2017-12-31
- 项目参与者:业宁; 王婷; 韦素云; 喻垚慎; 刘俊焱; 尹婷婷;
- 关键词:
项目摘要
With the development of information technology and the ongoing emergence of new domains, the image data acquired by people is fiercely increasing in terms of dimensionality and cannot also be guaranteed to be independent and identically distributed. How to extract the features from these image data is a very challenging problem. There are few efforts on transfer subspace learning that have made some preliminary attempts to solve this problem. However, still there exist many unsolved problems. Based on the theory of low-rank transfer representation, this project will design some novel low-rank discriminant transfer subspace feature extraction algirhtms with high discrimination for images.To be specific, the reseach topics of this project maily focus on four aspects, namely, 1)to propose a low-rank discriminant transfer subspace feature extraction algorithm via the robust distribution estimation, which considers both advantages and disadvantages of the low-rank transfer representation and the data distribution estimation stimutaneously;2) to design a low-rank preserving discriminant transfer subspace feature extraction algorithm, an extension of the above work, which aims to mine and utilize the underlying discriminant knowledge inside the low-rank transfer representation; 3) to develop a maximum slack margin low-rank discriminant transfer subspace feature extraction algorithm, which is to solve the problem that the above works cannot absolutely guarantee the extraction of the optimal discriminant features for testing image data.Finally, these algorithms are applied on low-quality image recognition problems to verify their effectiveness. The project development will give the research on transfer subspace learning a shot in the arm and simultaneously enrich the theoretical system of feature extraction.
随着信息技术的发展和新领域的不断涌现,人们获取的图像数据不仅维数上迅猛增长,分布上也难以保证满足独立同分布原则。如何从这样的数据中提取有效特征是一个极具挑战的问题。有关迁移子空间特征抽取研究在此方面做了初步的尝试,但仍有很多问题尚未解决。本项目以低秩迁移描述理论为基础,拟设计更具判别力的低秩判别迁移子空间特征提取算法。研究内容包括:1)平衡低秩迁移描述和分布估计的优劣,拟提出基于鲁棒分布估计的低秩判别迁移子空间特征提取算法;2)充分利用低秩迁移描述中潜在判别知识,对上述研究进行拓展,拟提出低秩保留判别迁移子空间特征抽取算法;3)针对上述研究不能保证测试集上判别特征最优提取问题,拟设计最大松弛间隔低秩判别迁移子空间特征抽取算法。最后,在低质量图像识别问题上,对所提算法有效性进行验证和定量分析。本项目的开展必将为迁移子空间分析研究注入新鲜的血液,丰富和发展模式识别、图像特征提取技术的理论体系。
结项摘要
随着信息技术的发展和新领域的不断涌现,人们获取的图像数据不仅维数上迅猛增长,分布上也难以保证满足独立同分布原则。如何从这样的数据中提取有效特征是一个极具挑战的问题。有关迁移子空间特征抽取研究在此方面做了初步的尝试,但仍有很多问题尚未解决。本项目以低秩迁移描述理论为基础,设计了更具判别力的低秩判别迁移子空间特征提取算法。具体的,从理论层面给出了基于图嵌入回归子空间特征抽取中经典的随机游走SRW方法的正则化框架,研究了其与已有的标记繁衍方法关系,提出了迭代正交化的基于图嵌入回归迁移子空间特征抽取模型,解决了判别投影不相关和投影向量数量受限问题;从理论上得出了已有的局部判别子空间学习技术本质上是通过加权实现图像数据局部拓扑结构的挖掘,但无法保证图像的鲁棒迁移能力,为此,研究了基于L1/Lp范数最大最小距离的鲁棒判别子空间特征抽取和聚类模型;研究了快速正交鲁棒判别迁移子空间特征抽取模型,其创新点在于应用了QR和回归联合模型加速了模型的计算,模型求解最终简单归结为一个回归问题;揭示了已有的鲁棒判别子空间特征抽取方法的内在联系,提出了非峰鲁棒判别模型。最后,在受光照变化、腐蚀、遮挡大的人脸、地形、手写体等图像集上,通过计算、比较识别精度和计算代价,验证了所提算法的有效性。本项目的开展能为迁移子空间分析研究注入新鲜的血液,丰富和发展模式识别、图像特征提取技术的理论体系。
项目成果
期刊论文数量(12)
专著数量(0)
科研奖励数量(0)
会议论文数量(0)
专利数量(0)
A Feature Selection Method for Projection Twin Support Vector Machine
投影双支持向量机的特征选择方法
- DOI:10.1007/s11063-017-9624-4
- 发表时间:2017-06
- 期刊:Neural Processing Letters
- 影响因子:3.1
- 作者:Rui Yan;Qiaolin Ye;Liyan Zhang;Ning Ye;Xiangbo Shu
- 通讯作者:Xiangbo Shu
L1-Norm Distance Linear Discriminant Analysis Based on an Effective Iterative Algorithm
基于有效迭代算法的L1-范数距离线性判别分析
- DOI:10.1109/tcsvt.2016.2596158
- 发表时间:2018
- 期刊:IEEE Transactions on Circuits and Systems for Video Technology
- 影响因子:8.4
- 作者:赵春霞;刘凡;Ye Ning;Yin Tongming
- 通讯作者:Yin Tongming
Comments on “Joint Global and Local Structure Discriminant Analysis”
对“联合全局和局部结构判别分析”的评论
- DOI:10.1109/tifs.2015.2490624
- 发表时间:2016-02
- 期刊:IEEE TRANSACTIONS ON INFORMATION FORENSICS AND SECURITY
- 影响因子:6.8
- 作者:业巧林
- 通讯作者:业巧林
L1-Norm Distance Minimization-Based Fast Robust Twin Support Vector k-Plane Clustering
基于L1范数距离最小化的快速鲁棒双支持向量k平面聚类
- DOI:10.1109/tnnls.2017.2749428
- 发表时间:2018-09-01
- 期刊:IEEE TRANSACTIONS ON NEURAL NETWORKS AND LEARNING SYSTEMS
- 影响因子:10.4
- 作者:Ye, Qiaolin;Zhao, Henghao;Ye, Ning
- 通讯作者:Ye, Ning
Can the Virtual Labels Obtained by Traditional LP Approaches Be Well Encoded in WLR?
传统LP方式获得的虚拟标签能否很好地编码在WLR中?
- DOI:10.1109/tnnls.2015.2499311
- 发表时间:2016-07
- 期刊:IEEE Transactions on Neural Networks and Learning Systems
- 影响因子:10.4
- 作者:Ye Qiaolin;Yang Jian;Yin Tongming;Zhang Zhao
- 通讯作者:Zhang Zhao
数据更新时间:{{ journalArticles.updateTime }}
{{
item.title }}
{{ item.translation_title }}
- DOI:{{ item.doi || "--"}}
- 发表时间:{{ item.publish_year || "--" }}
- 期刊:{{ item.journal_name }}
- 影响因子:{{ item.factor || "--"}}
- 作者:{{ item.authors }}
- 通讯作者:{{ item.author }}
数据更新时间:{{ journalArticles.updateTime }}
{{ item.title }}
- 作者:{{ item.authors }}
数据更新时间:{{ monograph.updateTime }}
{{ item.title }}
- 作者:{{ item.authors }}
数据更新时间:{{ sciAawards.updateTime }}
{{ item.title }}
- 作者:{{ item.authors }}
数据更新时间:{{ conferencePapers.updateTime }}
{{ item.title }}
- 作者:{{ item.authors }}
数据更新时间:{{ patent.updateTime }}
其他文献
一种新的支持向量分类算法ACNN-SVM
- DOI:--
- 发表时间:--
- 期刊:郑州大学学报(理学版)
- 影响因子:--
- 作者:武波;业宁;宋爱美;张训华;业巧林
- 通讯作者:业巧林
基于正则化技术的对支持向量机特征选择算法
- DOI:--
- 发表时间:--
- 期刊:计算机研究与发展
- 影响因子:--
- 作者:业巧林;赵春霞;陈小波
- 通讯作者:陈小波
一种非监督的道路场景分割方法
- DOI:--
- 发表时间:2012
- 期刊:南京理工大学学报
- 影响因子:--
- 作者:张浩峰;业巧林
- 通讯作者:业巧林
面向移动平台的深度学习复杂场景目标识别应用
- DOI:10.15983/j.cnki.jsnu.2019.05.152
- 发表时间:2019
- 期刊:陕西师范大学学报(自然科学版)
- 影响因子:--
- 作者:许博鸣;刘晓峰;业巧林;张福全;周京正
- 通讯作者:周京正
Recursive Dimension Reduction for semisupervised learning
半监督学习的递归降维
- DOI:10.1016/j.neucom.2015.06.062
- 发表时间:2016
- 期刊:Neurocomputing
- 影响因子:6
- 作者:业巧林;尹同明;高尚兵;景佳佳;张宇
- 通讯作者:张宇
其他文献
{{
item.title }}
{{ item.translation_title }}
- DOI:{{ item.doi || "--" }}
- 发表时间:{{ item.publish_year || "--"}}
- 期刊:{{ item.journal_name }}
- 影响因子:{{ item.factor || "--" }}
- 作者:{{ item.authors }}
- 通讯作者:{{ item.author }}
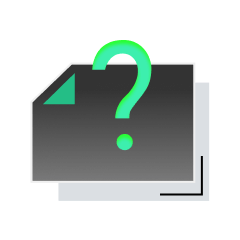
内容获取失败,请点击重试
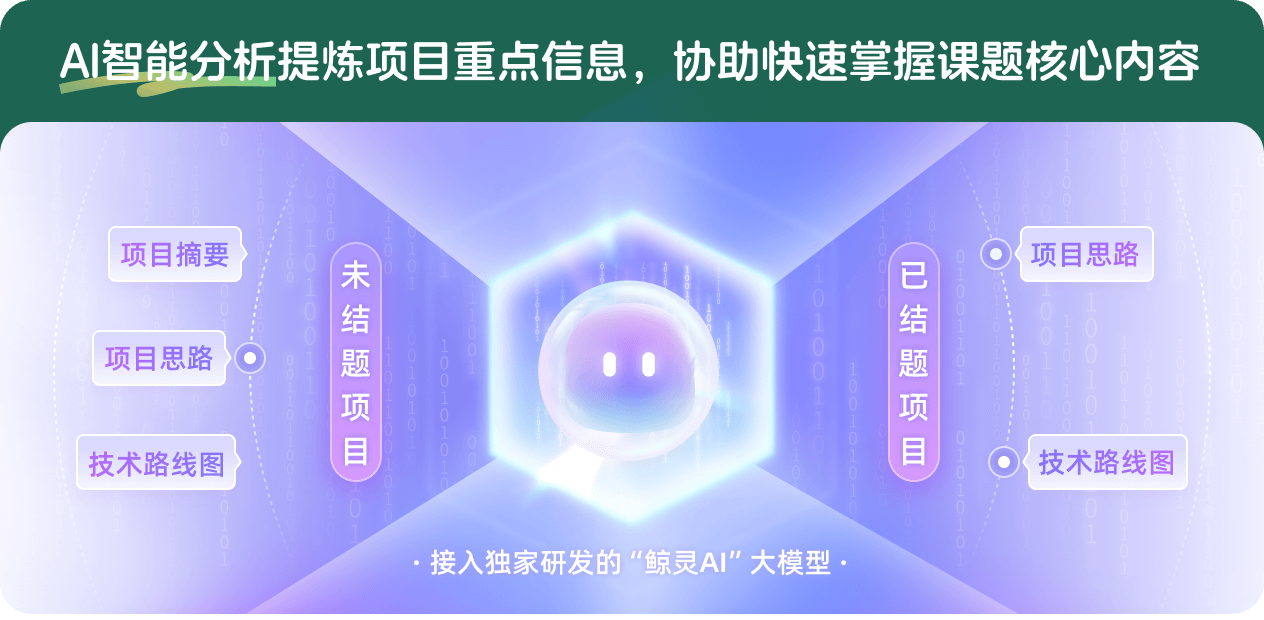
查看分析示例
此项目为已结题,我已根据课题信息分析并撰写以下内容,帮您拓宽课题思路:
AI项目摘要
AI项目思路
AI技术路线图
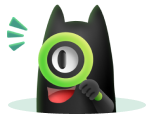
请为本次AI项目解读的内容对您的实用性打分
非常不实用
非常实用
1
2
3
4
5
6
7
8
9
10
您认为此功能如何分析更能满足您的需求,请填写您的反馈:
业巧林的其他基金
基于AIoT半球摄影的山地森林叶面积指数精准测算研究
- 批准号:32371877
- 批准年份:2023
- 资助金额:50.00 万元
- 项目类别:面上项目
面向复杂多视图图像的鲁棒判别距离度量协同学习研究
- 批准号:62072246
- 批准年份:2020
- 资助金额:56 万元
- 项目类别:面上项目
鲁棒判别的多视角自适应子空间学习及其在异质图像识别上的应用研究
- 批准号:61871444
- 批准年份:2018
- 资助金额:16.0 万元
- 项目类别:面上项目
相似国自然基金
{{ item.name }}
- 批准号:{{ item.ratify_no }}
- 批准年份:{{ item.approval_year }}
- 资助金额:{{ item.support_num }}
- 项目类别:{{ item.project_type }}
相似海外基金
{{
item.name }}
{{ item.translate_name }}
- 批准号:{{ item.ratify_no }}
- 财政年份:{{ item.approval_year }}
- 资助金额:{{ item.support_num }}
- 项目类别:{{ item.project_type }}