多伯努利分布框架下的多细胞运动分析方法研究
项目介绍
AI项目解读
基本信息
- 批准号:61876024
- 项目类别:面上项目
- 资助金额:60.0万
- 负责人:
- 依托单位:
- 学科分类:F0608.智能系统与人工智能安全
- 结题年份:2022
- 批准年份:2018
- 项目状态:已结题
- 起止时间:2019-01-01 至2022-12-31
- 项目参与者:徐本连; 施健; 王飞; 朱继红; 张董; 李明月; 孙乙丹;
- 关键词:
项目摘要
In time and space field, aiming to tracking difficult problems, such as large amount of information, complex and changeable shape of cell structure, division, collision, adhesion and the uncertainty of cell movement, multi-cell state estimation and tracking methods with engineering application prospect are put forward in the Multi-Bernoulli distribution framework. Firstly, theory model for multi cell tracking of the Multi-Bernoulli label Random Finite Set (RFS) and the Multi-Bernoulli label random finite ant colony are proposed. On this basis, through adding collision and division parameters to Multi-Bernoulli filtering state parameters set, augmented labeled Multi-Bernoulli filtering and multiple model Multi-Bernoulli filtering multi-cell tracking method are proposed. Then, in the Multi-Bernoulli distribution framework, constructing the dual prediction of multi ant colony state and pheromone field and fast updating mechanism, label Random Finite ant colony multi-cell tracking methods are proposed in Multi-Bernoulli distribution framework. Finally, from the three dimensions of the algorithm itself, the biological measure and the practicality, an objective evaluation system for cell tracking algorithm is studied. It is believed that our results will enrich multi-cell tracking theory and contribute to the development of pathology and drug discovery, and provide new ideas and technical as well.
在时间与空间域里,针对细胞数据量大,细胞个体易变形、分裂,细胞间碰撞、粘连等运动不确定等跟踪难题,本项目拟在多伯努利分布框架下,提出具有工程应用前景的多细胞跟踪方法。首先,提出面向多细胞跟踪的多伯努利标签随机有限集和多伯努利标签随机有限蚁群理论模型;在此基础上,对多伯努利滤波目标状态参数集进行扩展(引入分裂、碰撞事件),提出扩展标签多伯努利、多模型多伯努利细胞状态估计与跟踪方法;在多伯努利分布假设框架下,构建多蚁群状态与信息素场双重预测与快速更新机制,提出多伯努利框架下的标签随机有限蚁群多细胞跟踪方法。最后,从算法本身、生物学度量和实用性等三个维度,研发细胞跟踪算法的客观评价系统。该项目研究成果将进一步完善和丰富细胞跟踪与评价方法,并为病理学研究和新药物的研制提供新的思路和技术途径。
结项摘要
细胞迁移运动是血管生成、损伤愈合、胚胎生长、免疫反应、感染和癌症转移等多种生理过程必不可少的环节。细胞运动分析方法作为一种医学辅助手段对于提高疾病诊断和预防的准确性、药效分析和药物研发的效率、可靠性具有重要的理论研究价值和工程实践意义。本项目针对细胞数据量大,细胞个体易变形、分裂,细胞间碰撞、粘连等运动不确定跟踪难题,在面向多细胞跟踪的多伯努利(标签)随机有限集和多伯努利(标签)随机有限蚁群理论模型研究的基础上,提出具有工程应用前景的多伯努利多细胞跟踪方法。本课题研究的重要成果主要体现在:(1)针对粘连多细胞跟踪精度低的问题,研究了基于蚂蚁自调整觅食的多细胞跟踪方法;(2)针对近距离黏连,碰撞细胞的状态估计问题,提出了基于信息素预测的蚁群多细胞运动分析方法;(3)针对细胞动力学差异性大、细胞黏连与分裂谱系树构建问题,提出基于标签蚁群的多细胞谱系树构建方法;(4)针对细胞数量时变、细胞图像信噪比低等难题,提出基于标签多贝努利滤波器的多细胞运动分析方法;(5)针对细胞数量及形态时变等难题,提出基于多伯努利随机有限蚁群多细胞状态参数与形态学参数估计方法;(6)针对低信噪比图像细胞分裂频率高等难题,提出双层多伯努利随机有限蚁群多细胞运动分析方法等,实现复杂情形下多细胞数量、位置、速度、轨迹等运动参数和形态学参数的自动估计。研究成果不仅有助于丰富多目标(细胞)跟踪的理论方法,也可为药理学和病理学的研究提供重要的参考价值和技术路径。
项目成果
期刊论文数量(12)
专著数量(0)
科研奖励数量(0)
会议论文数量(10)
专利数量(10)
A Novel Gaussian Ant Colony Algorithm for Clustering Cell Tracking
一种用于细胞聚类跟踪的新型高斯蚁群算法
- DOI:10.1155/2021/9205604
- 发表时间:2021-09
- 期刊:DISCRETE DYNAMICS IN NATURE AND SOCIETY
- 影响因子:1.4
- 作者:Lu Mingli;Wu Di;Jin Yuchen;Shi Jian;Xu Benlian;Cong Jinliang;Ma Yingying;Lu Jiadi
- 通讯作者:Lu Jiadi
Multi-cell multi-Bernoulli tracking method based on fractal measurement model
基于分形测量模型的多小区多伯努利跟踪方法
- DOI:10.1504/ijmic.2021.121838
- 发表时间:2021
- 期刊:International Journal of Modelling, Identification and Control
- 影响因子:--
- 作者:Zhu Jihong;Lu Mingli;Wang Fei
- 通讯作者:Wang Fei
A Joint Tracking Approach via Ant Colony Evolution for Quantitative Cell Cycle Analysis
通过蚁群进化进行细胞周期定量分析的联合跟踪方法
- DOI:10.1109/jbhi.2020.3032592
- 发表时间:2021
- 期刊:IEEE JOURNAL OF BIOMEDICAL AND HEALTH INFORMATICS
- 影响因子:7.7
- 作者:Xu Benlian;Lu Mingli;Shi Jian;Cong Jinliang;Nener Brett
- 通讯作者:Nener Brett
An accurate cell tracking approach with self-regulated foraging behavior of ant colonies in dynamic microscopy images
动态显微镜图像中蚁群自我调节觅食行为的精确细胞跟踪方法
- DOI:10.1007/s10489-021-02424-0
- 发表时间:2021-05
- 期刊:Applied Intelligence
- 影响因子:5.3
- 作者:Lu Mingli;Xu Benlian;Brett Nener;Cong Jinliang;Shi Jian
- 通讯作者:Shi Jian
Distributed fusion algorithm based on maximum internal ellipsoid mechanism
基于最大内椭球机制的分布式融合算法
- DOI:10.1504/ijcat.2021.121529
- 发表时间:2021
- 期刊:International Journal of Computer Applications in Technology
- 影响因子:1.1
- 作者:Cong Jinliang;Xu Benlian;Lu Mingli;Shi Jian;Fang Chi;Yu Qian;Sheng Andong
- 通讯作者:Sheng Andong
数据更新时间:{{ journalArticles.updateTime }}
{{
item.title }}
{{ item.translation_title }}
- DOI:{{ item.doi || "--"}}
- 发表时间:{{ item.publish_year || "--" }}
- 期刊:{{ item.journal_name }}
- 影响因子:{{ item.factor || "--"}}
- 作者:{{ item.authors }}
- 通讯作者:{{ item.author }}
数据更新时间:{{ journalArticles.updateTime }}
{{ item.title }}
- 作者:{{ item.authors }}
数据更新时间:{{ monograph.updateTime }}
{{ item.title }}
- 作者:{{ item.authors }}
数据更新时间:{{ sciAawards.updateTime }}
{{ item.title }}
- 作者:{{ item.authors }}
数据更新时间:{{ conferencePapers.updateTime }}
{{ item.title }}
- 作者:{{ item.authors }}
数据更新时间:{{ patent.updateTime }}
其他文献
A multiCell visual tracking algorithm using multi-task particle swarm optimization for low-contrast image sequences
针对低对比度图像序列使用多任务粒子群优化的多细胞视觉跟踪算法
- DOI:10.1007/s10489-016-0802-2
- 发表时间:2016-07
- 期刊:Applied Intelligence
- 影响因子:5.3
- 作者:徐本连;朱培逸;鲁明丽;蒋冬梅
- 通讯作者:蒋冬梅
其他文献
{{
item.title }}
{{ item.translation_title }}
- DOI:{{ item.doi || "--" }}
- 发表时间:{{ item.publish_year || "--"}}
- 期刊:{{ item.journal_name }}
- 影响因子:{{ item.factor || "--" }}
- 作者:{{ item.authors }}
- 通讯作者:{{ item.author }}
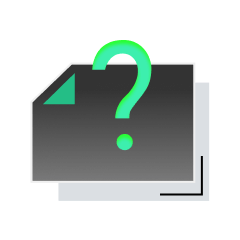
内容获取失败,请点击重试
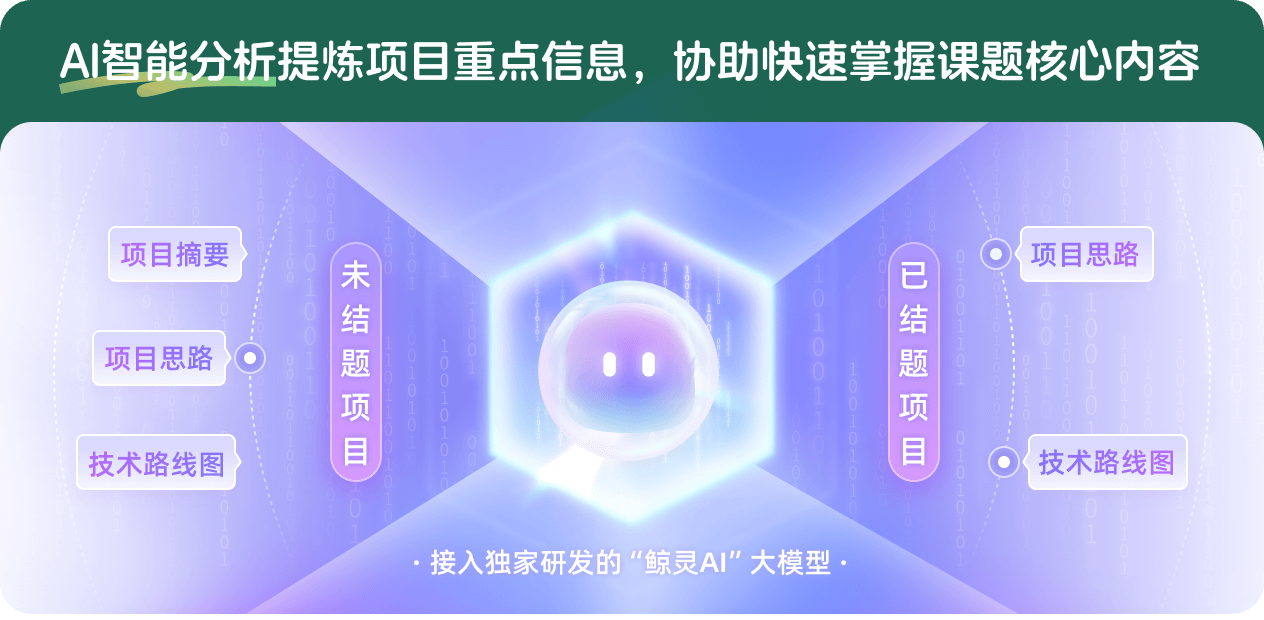
查看分析示例
此项目为已结题,我已根据课题信息分析并撰写以下内容,帮您拓宽课题思路:
AI项目摘要
AI项目思路
AI技术路线图
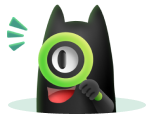
请为本次AI项目解读的内容对您的实用性打分
非常不实用
非常实用
1
2
3
4
5
6
7
8
9
10
您认为此功能如何分析更能满足您的需求,请填写您的反馈:
相似国自然基金
{{ item.name }}
- 批准号:{{ item.ratify_no }}
- 批准年份:{{ item.approval_year }}
- 资助金额:{{ item.support_num }}
- 项目类别:{{ item.project_type }}
相似海外基金
{{
item.name }}
{{ item.translate_name }}
- 批准号:{{ item.ratify_no }}
- 财政年份:{{ item.approval_year }}
- 资助金额:{{ item.support_num }}
- 项目类别:{{ item.project_type }}