基于偏相关系数截断法的超高维模型的变量选择
项目介绍
AI项目解读
基本信息
- 批准号:11401497
- 项目类别:青年科学基金项目
- 资助金额:22.0万
- 负责人:
- 依托单位:
- 学科分类:A0403.贝叶斯统计与统计应用
- 结题年份:2017
- 批准年份:2014
- 项目状态:已结题
- 起止时间:2015-01-01 至2017-12-31
- 项目参与者:冯峥晖; 李迎星; 钟威; 张传海; 范云菲; 张诗悦;
- 关键词:
项目摘要
The penalty-based variable selection technique is one of the appealing methods to identify significant variables in the high dimensional models. But for the ultrahigh dimensional models where the number of predictors increases exponentially with the sample size, an extra screening step is often needed to roughly reduce the model dimension. This project, however, aims to propose a different ultrahigh dimensional variable selection scheme, called the thresholded partial correlation approach (TPC). The TPC is based on the idea of partial correlation learning and the partial faithfulness for the ultrahigh dimensional linear models, and it can be extended to the partially linear models via the partial residual approach. This method relaxes the normality assumption of the predictors and responses to the elliptical contoured distribution, and does not need the two-stage procedure as most current methods do, in the ultrahigh dimensional setting, to obtain the final sparse model. Furthermore, we will establish the theoretical properties of this method under a different assumption framework from the literature, which allows us to broaden the usage of this variable selection scheme. We will prove the model consistency of the TPC and the sure screening property of the first step of TPC for both linear models and partially linear models. The simulation examples will be conducted to compare the TPC and the penalty-based variable selection approaches, and the application of this method to the genetic research and financial research will also be provided.
惩罚回归是在大数据模型中提取有用信息的办法之一。但对自变量个数随样本容量指数增长的超高维模型,我们通常需要一步额外的独立扫描步骤来降低模型维数。然而本项目旨在提出一种来源于不同理念框架的超高维变量选择方法——偏相关系数截断法。总体来说,此方法是基于偏相关系数体系以及部分忠实性理念的逐步检验法。它不仅可以处理超高维线性模型,也可以结合部分残差法扩展应用于超高维部分线性模型中。该方法将模型自变量和因变量的正态假设条件放宽为椭圆分布以适应真实数据通常面临的重尾性;它可以直接作用于超高维模型,无需进行传统的两步法;另外,为使本方法应用更加广泛,我们将从与传统方法不同的正则条件框架出发构造该方法的大样本性质,包括模型选择相合性、第一步检验的确定扫描性等。我们将利用模拟实验来比较偏相关系数截断法和传统的惩罚回归对于超高维模型的变量选择结果,并且将本方法应用于生物基因学领域和金融股票研究领域的数据分析。
结项摘要
惩罚回归是在大数据模型中提取有用信息的办法之一。但对自变量个数随样本容量指数增长的超高维模型,我们通常需要一步额外的独立扫描步骤来降低模型维数。然而本项目旨在提出一种来源于不同理念框架的超高维变量选择方法——偏相关系数截断法。总体来说,此方法是基于偏相关系数体系以及部分忠实性理念的逐步检验法。它不仅可以处理超高维线性模型,也可以结合部分残差法扩展应用于超高维部分线性模型中。该方法将模型自变量和因变量的正态假设条件放宽为椭圆分布以适应真实数据通常面临的重尾性;它可以直接作用于超高维模型,无需进行传统的两步法;另外,为使本方法应用更加广泛,我们将从与传统方法不同的正则条件框架出发构造该方法的大样本性质,包括模型选择相合性、第一步检验的确定扫描性等。我们将利用模拟实验来比较偏相关系数截断法和传统的惩罚回归对于超高维模型的变量选择结果,并且将本方法应用于生物基因学领域和金融股票研究领域的数据分析。
项目成果
期刊论文数量(5)
专著数量(0)
科研奖励数量(0)
会议论文数量(0)
专利数量(0)
2HiGWAS: a unifying high-dimensional platform to infer the global genetic architecture of trait development
2HiGWAS:一个统一的高维平台,用于推断性状发展的全球遗传架构
- DOI:10.1093/bib/bbv002
- 发表时间:2015-11-01
- 期刊:BRIEFINGS IN BIOINFORMATICS
- 影响因子:9.5
- 作者:Jiang, Libo;Liu, Jingyuan;Wu, Rongling
- 通讯作者:Wu, Rongling
Model-Free Conditional Independence Feature Screening For Ultrahigh Dimensional Data.
无模型条件独立特征筛选
- DOI:10.1007/s11425-016-0186-8
- 发表时间:2017-03
- 期刊:Science China. Mathematics
- 影响因子:--
- 作者:Wang L;Liu J;Li Y;Li R
- 通讯作者:Li R
Feature Screening and Variable Selection for Partially Linear Models with Ultrahigh-dimensional Longitudinal Data
超高维纵向数据部分线性模型的特征筛选和变量选择
- DOI:10.1016/j.neucom.2015.09.122
- 发表时间:2016
- 期刊:Neurocomputing
- 影响因子:6
- 作者:Jingyuan Liu
- 通讯作者:Jingyuan Liu
A selective overview of feature screening for ultrahigh-dimensional data.
超高维数据特征筛选的选择性概述
- DOI:10.1007/s11425-015-5062-9
- 发表时间:2015-10
- 期刊:Science China. Mathematics
- 影响因子:--
- 作者:JingYuan L;Wei Z;RunZe LI
- 通讯作者:RunZe LI
数据更新时间:{{ journalArticles.updateTime }}
{{
item.title }}
{{ item.translation_title }}
- DOI:{{ item.doi || "--"}}
- 发表时间:{{ item.publish_year || "--" }}
- 期刊:{{ item.journal_name }}
- 影响因子:{{ item.factor || "--"}}
- 作者:{{ item.authors }}
- 通讯作者:{{ item.author }}
数据更新时间:{{ journalArticles.updateTime }}
{{ item.title }}
- 作者:{{ item.authors }}
数据更新时间:{{ monograph.updateTime }}
{{ item.title }}
- 作者:{{ item.authors }}
数据更新时间:{{ sciAawards.updateTime }}
{{ item.title }}
- 作者:{{ item.authors }}
数据更新时间:{{ conferencePapers.updateTime }}
{{ item.title }}
- 作者:{{ item.authors }}
数据更新时间:{{ patent.updateTime }}
其他文献
基于居民感知的社区低碳环境教育评价—以衡阳市为例
- DOI:--
- 发表时间:2016
- 期刊:衡阳师范学院学报
- 影响因子:--
- 作者:杨立国;李苏毅;刘婧媛;等
- 通讯作者:等
嗅鞘细胞移植入淀粉样前体蛋白转基因小鼠脑内降低淀粉样蛋白沉积
- DOI:--
- 发表时间:--
- 期刊:Acta Anatomica Sinica
- 影响因子:--
- 作者:曹敬丽;LIU Jing-yuan;XIAO Juan;JIANG Xiao-rong;REN Yu-shui;WANG Hong-mei;ZHOU Chang-man;HUANG Hong-yun;刘婧媛;肖娟;姜晓荣;任玉水;王洪美;周长满;黄红云;CAO Jing-li
- 通讯作者:CAO Jing-li
成人鼻腔嗅区黏膜细胞的分离培养与免疫学特点
- DOI:--
- 发表时间:--
- 期刊:Acta Anatomica Sinica
- 影响因子:--
- 作者:王洪美;REN Yu-shui;TIAN Guo-zhong;JIANG Xiao-rong;CAO Jing-li;LIU Jing-yuan;ZHOU Chang-man;HUANG Hong-yun;任玉水;田国忠;姜晓荣;曹敬丽;刘婧媛;周长满;黄红云;WANG Hong-mei
- 通讯作者:WANG Hong-mei
氮掺杂碳纤维负载镍钴硒化物的制备及其电催化析氢性能
- DOI:--
- 发表时间:2022
- 期刊:无机化学学报
- 影响因子:--
- 作者:于静;张婷;刘琦;刘婧媛;王君
- 通讯作者:王君
其他文献
{{
item.title }}
{{ item.translation_title }}
- DOI:{{ item.doi || "--" }}
- 发表时间:{{ item.publish_year || "--"}}
- 期刊:{{ item.journal_name }}
- 影响因子:{{ item.factor || "--" }}
- 作者:{{ item.authors }}
- 通讯作者:{{ item.author }}
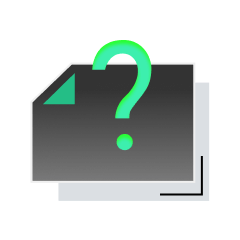
内容获取失败,请点击重试
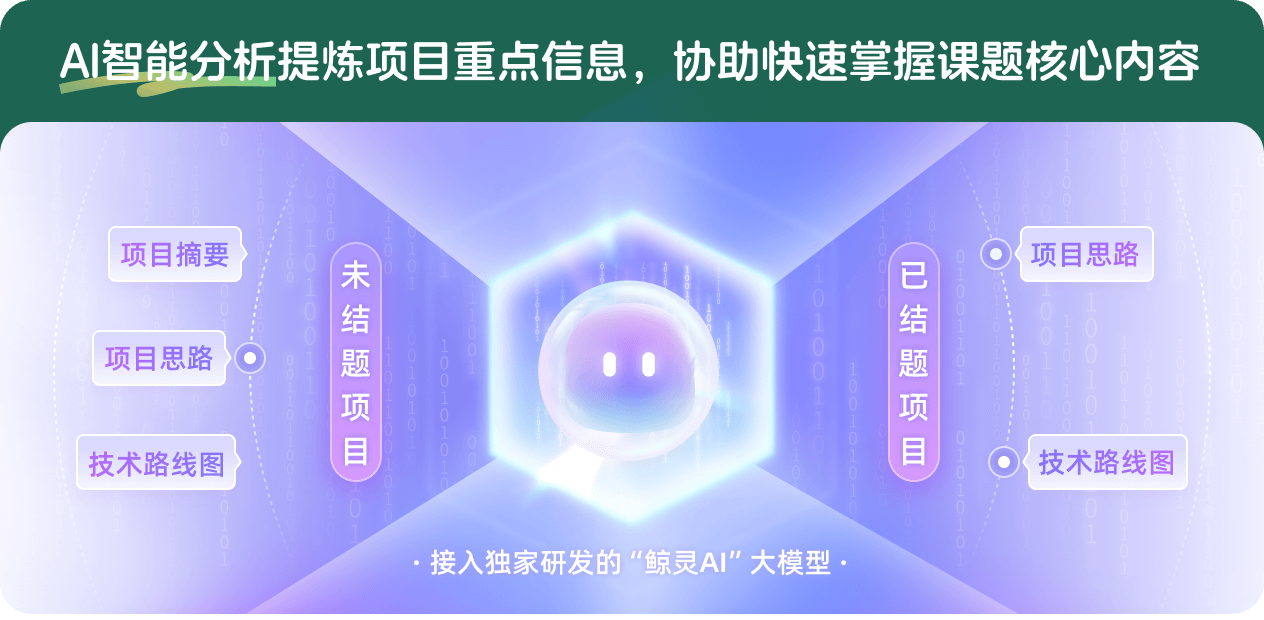
查看分析示例
此项目为已结题,我已根据课题信息分析并撰写以下内容,帮您拓宽课题思路:
AI项目摘要
AI项目思路
AI技术路线图
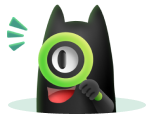
请为本次AI项目解读的内容对您的实用性打分
非常不实用
非常实用
1
2
3
4
5
6
7
8
9
10
您认为此功能如何分析更能满足您的需求,请填写您的反馈:
刘婧媛的其他基金
错误发现率控制方法的统计理论及应用
- 批准号:12271456
- 批准年份:2022
- 资助金额:46 万元
- 项目类别:面上项目
高维模型误差项分布的研究及应用
- 批准号:11771361
- 批准年份:2017
- 资助金额:48.0 万元
- 项目类别:面上项目
相似国自然基金
{{ item.name }}
- 批准号:{{ item.ratify_no }}
- 批准年份:{{ item.approval_year }}
- 资助金额:{{ item.support_num }}
- 项目类别:{{ item.project_type }}
相似海外基金
{{
item.name }}
{{ item.translate_name }}
- 批准号:{{ item.ratify_no }}
- 财政年份:{{ item.approval_year }}
- 资助金额:{{ item.support_num }}
- 项目类别:{{ item.project_type }}