面向知识学习与资源高效利用的复杂非线性系统自适应评判控制
项目介绍
AI项目解读
基本信息
- 批准号:61773373
- 项目类别:面上项目
- 资助金额:66.0万
- 负责人:
- 依托单位:
- 学科分类:F0301.控制理论与技术
- 结题年份:2021
- 批准年份:2017
- 项目状态:已结题
- 起止时间:2018-01-01 至2021-12-31
- 项目参与者:朱圆恒; 赵博; 马宏文; 徐延才; 陈亚冉; 梁明明; 唐振韬; 林桥; 李本凯;
- 关键词:
项目摘要
With the rapid development of information technology, such as artificial intelligence, big data, and deep learning, adaptive critic and reinforcement learning are regarded as promising schemes involving evaluation component to accomplish intelligent optimization. Nowadays, increasing communication burdens are often brought in by network-based techniques. In addition, more and more dynamical systems are encountered with complex uncertain factors and the difficulty of building accurately mathematical model. In this project, the adaptive critic control theory and methods of complex nonlinear systems are investigated toward knowledge learning and efficient resource utilization. First, the adaptive optimal control approaches of complex nonlinear systems are developed based on an improved learning mechanism, followed by sufficient discussions on their robustness performance. Then, the event-triggered self-learning robust control and adaptive H∞ control of nonlinear systems are studied respectively, thereby extending the framework of traditional adaptive critic designs. Moreover, the novel adaptive critic control policies of nonlinear systems are established by using a mixed data and event driven scheme. Finally, the neural network implementation and closed-loop stability of the present methods are performed in detail. In addition, by carrying out experimental simulation and application study on practical plants, such as power systems and overhead crane systems, the effectiveness and superiority of the present approaches are verified. Through improving the adaptive critic learning mechanism and efficiently utilizing data and communication resources, it is important to note that the given strategies can not only strengthen the results of control theory with data-based and event-triggered formulations, but also provide new avenues to robust control and H∞ control designs of complex nonlinear systems. Therefore, it will be beneficial for the construction of advanced automation techniques and intelligent systems as well as of great significance both in theory and application.
随着人工智能、大数据、深度学习等信息技术的快速发展,自适应评判与强化学习成为融合评价机制、实现智能优化的重要方法。鉴于网络化技术带来通信负担加剧,越来越多的动态系统存在复杂不确定因素、难以精确建模等,本项目面向知识学习与资源高效利用,研究复杂非线性系统的自适应评判控制理论与方法。首先,提出基于改进学习机制的自适应优化控制方法并讨论鲁棒性能。其次,研究非线性系统事件驱动自学习鲁棒控制和自适应H∞控制,推广传统的自适应评判体系。再次,建立数据、事件混合驱动的新型自适应评判控制策略。最后,探讨神经网络实现,分析控制系统稳定性,并针对电力、吊车等实际系统开展仿真实验与应用研究。通过改善自适应评判学习机制,高效利用数据、通信资源,本项目能够深化基于数据和事件驱动的控制理论成果,为复杂非线性系统的鲁棒控制和H∞控制设计开辟新途径,也将有助于构建先进自动化技术与智能系统,具有重要的理论意义和应用价值。
结项摘要
复杂动态系统的优化控制设计是控制领域的研究热点之一。由于实际非线性系统往往存在着不确定性、关联、建模困难等复杂因素,而且网络化技术的普及加剧了控制系统的通信负担,在智能科学与技术的有力推动下,获取人工智能驱动的先进优化控制方案正在成为相关领域的研究难点。本项目面向知识学习与资源高效利用,研究复杂非线性系统的自适应评判控制理论与方法,旨在逐步完善自适应评判控制的研究框架。首先,提出采用改进学习机制的自适应优化控制方法并研究其鲁棒性能,针对一般连续时间非线性系统的自适应优化控制、不确定系统的自学习鲁棒镇定与跟踪控制、关联系统的自学习分散控制设计、含有输入约束非线性系统的自学习干扰抑制等问题,分别获得了新颖的研究成果。其次,探讨非线性系统的事件驱动自学习鲁棒控制和自适应H∞控制,得到实现鲁棒镇定和保成本控制的先进设计策略,并在考虑外部扰动的情况下获得事件驱动干扰抑制方案,由此显著推广了自适应评判方法。再次,充分利用知识学习与提高资源利用效率的要求,初步建立了针对连续时间系统的数据、事件混合驱动自适应评判控制架构,在数据驱动控制和事件驱动控制方面分别深化了自适应评判控制理论。最后,开展神经网络实现与控制系统稳定性分析,并针对电力、吊车等实际系统进行仿真应用研究,验证了相关技术的有效性。此外,项目组成员也在强化学习与智能控制、污水处理过程智能优化控制等方面进行了延伸,为在未来开展更加深入的研究工作打下了基础。通过开展本项工作,深化了不确定环境下非线性系统自适应优化控制的研究成果,显著扩大了自适应评判方法的使用范围,由此推动了复杂非线性系统智能优化控制的研究进展。本项目提出的一系列方法能够应用于不确定环境下几类电力系统的优化控制设计,也为开展其他复杂系统尤其是污水处理过程的智能评判控制设计提供了一定的技术支撑,从而有助于构建先进自动化与智能系统,具有潜在可观的经济效益和社会效益。
项目成果
期刊论文数量(37)
专著数量(2)
科研奖励数量(3)
会议论文数量(17)
专利数量(6)
Event-Triggered Adaptive Critic Control Design for Discrete-Time Constrained Nonlinear Systems
离散时间约束非线性系统的事件触发自适应临界控制设计
- DOI:10.1109/tsmc.2018.2868510
- 发表时间:2020-09
- 期刊:IEEE Transactions on Systems Man Cybernetics-Systems
- 影响因子:8.7
- 作者:Ha Mingming;Wang Ding;Liu Derong
- 通讯作者:Liu Derong
Data-Driven Iterative Adaptive Critic Control Toward an Urban Wastewater Treatment Plant
针对城市污水处理厂的数据驱动迭代自适应批评控制
- DOI:10.1109/tie.2020.3001840
- 发表时间:2021-08-01
- 期刊:IEEE TRANSACTIONS ON INDUSTRIAL ELECTRONICS
- 影响因子:7.7
- 作者:Wang, Ding;Ha, Mingming;Qiao, Junfei
- 通讯作者:Qiao, Junfei
Neural network robust tracking control with adaptive critic framework for uncertain nonlinear systems
不确定非线性系统自适应批评框架的神经网络鲁棒跟踪控制
- DOI:10.1016/j.neunet.2017.09.005
- 发表时间:2018-01-01
- 期刊:NEURAL NETWORKS
- 影响因子:7.8
- 作者:Wang, Ding;Liu, Derong;Li, Hongyi
- 通讯作者:Li, Hongyi
A data-based neural policy learning strategy towards robust tracking control design for uncertain dynamic systems
基于数据的神经策略学习策略,用于不确定动态系统的鲁棒跟踪控制设计
- DOI:10.1080/00207721.2021.2023685
- 发表时间:2022-01
- 期刊:International Journal of Systems Science
- 影响因子:4.3
- 作者:Wang Ding;Xu Xin
- 通讯作者:Xu Xin
Self-learning robust control synthesis and trajectory tracking of uncertain dynamics
不确定动力学的自学习鲁棒控制综合与轨迹跟踪
- DOI:--
- 发表时间:2022
- 期刊:IEEE Transactions on Cybernetics
- 影响因子:11.8
- 作者:Ding Wang;Long Cheng;Jun Yan
- 通讯作者:Jun Yan
数据更新时间:{{ journalArticles.updateTime }}
{{
item.title }}
{{ item.translation_title }}
- DOI:{{ item.doi || "--"}}
- 发表时间:{{ item.publish_year || "--" }}
- 期刊:{{ item.journal_name }}
- 影响因子:{{ item.factor || "--"}}
- 作者:{{ item.authors }}
- 通讯作者:{{ item.author }}
数据更新时间:{{ journalArticles.updateTime }}
{{ item.title }}
- 作者:{{ item.authors }}
数据更新时间:{{ monograph.updateTime }}
{{ item.title }}
- 作者:{{ item.authors }}
数据更新时间:{{ sciAawards.updateTime }}
{{ item.title }}
- 作者:{{ item.authors }}
数据更新时间:{{ conferencePapers.updateTime }}
{{ item.title }}
- 作者:{{ item.authors }}
数据更新时间:{{ patent.updateTime }}
其他文献
摇摆墙布置对框架抗震性能的影响
- DOI:10.19701/j.jzjg.2018.s2.093
- 发表时间:2018
- 期刊:建筑结构
- 影响因子:--
- 作者:李楠;王鼎;郝圣旺
- 通讯作者:郝圣旺
Sensor array calibration method in presence of gain/phase uncertainties and position perturbations using the spatial- and time-domain information of the auxiliary sources
存在增益/相位不确定性和位置扰动时使用辅助源的空间和时域信息的传感器阵列校准方法
- DOI:10.1007/s11045-014-0284-5
- 发表时间:2015-07
- 期刊:Multidimensional Systems and Signal Processing
- 影响因子:2.5
- 作者:王鼎;吴瑛
- 通讯作者:吴瑛
信号传播速度未知下水下多基地声纳定位算法
- DOI:10.12382/bgxb.2021.0134
- 发表时间:2022
- 期刊:兵工学报
- 影响因子:--
- 作者:范超;王鼎;杨宾;尹洁昕
- 通讯作者:尹洁昕
以丝素蛋白微球为模板制备介孔二氧化硅空心微球
- DOI:--
- 发表时间:--
- 期刊:化工新型材料
- 影响因子:--
- 作者:王鼎;朱晶心;陈松;贾兰
- 通讯作者:贾兰
细胞间相互作用影响iPSc来源肝细胞的成熟
- DOI:--
- 发表时间:2016
- 期刊:中国细胞生物学学报
- 影响因子:--
- 作者:王鼎;郭丽媛;李伟强
- 通讯作者:李伟强
其他文献
{{
item.title }}
{{ item.translation_title }}
- DOI:{{ item.doi || "--" }}
- 发表时间:{{ item.publish_year || "--"}}
- 期刊:{{ item.journal_name }}
- 影响因子:{{ item.factor || "--" }}
- 作者:{{ item.authors }}
- 通讯作者:{{ item.author }}
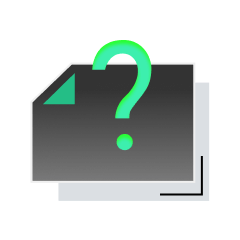
内容获取失败,请点击重试
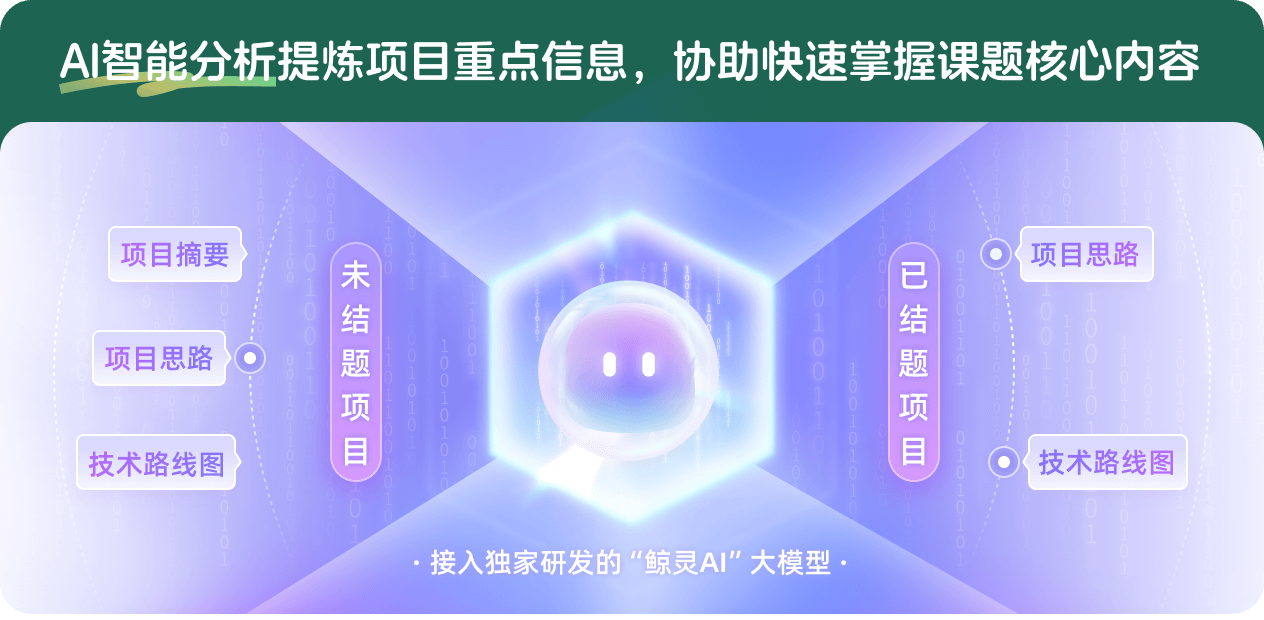
查看分析示例
此项目为已结题,我已根据课题信息分析并撰写以下内容,帮您拓宽课题思路:
AI项目摘要
AI项目思路
AI技术路线图
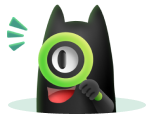
请为本次AI项目解读的内容对您的实用性打分
非常不实用
非常实用
1
2
3
4
5
6
7
8
9
10
您认为此功能如何分析更能满足您的需求,请填写您的反馈:
相似国自然基金
{{ item.name }}
- 批准号:{{ item.ratify_no }}
- 批准年份:{{ item.approval_year }}
- 资助金额:{{ item.support_num }}
- 项目类别:{{ item.project_type }}
相似海外基金
{{
item.name }}
{{ item.translate_name }}
- 批准号:{{ item.ratify_no }}
- 财政年份:{{ item.approval_year }}
- 资助金额:{{ item.support_num }}
- 项目类别:{{ item.project_type }}