Integrinβ3通过PCYT1A介导的胆碱代谢促BCSC富集与干性维持的机制研究
项目介绍
AI项目解读
基本信息
- 批准号:
- 项目类别:青年科学基金项目
- 资助金额:30万
- 负责人:
- 依托单位:
- 学科分类:
- 结题年份:
- 批准年份:2021
- 项目状态:未结题
- 起止时间:2021至
- 项目参与者:文思阳;
- 关键词:
项目摘要
结项摘要
项目成果
期刊论文列表
专著列表
科研奖励列表
会议论文列表
专利列表
数据更新时间:{{ journalArticles.updateTime }}
{{
item.title }}
{{ item.translation_title }}
- DOI:{{ item.doi || "--"}}
- 发表时间:{{ item.publish_year || "--" }}
- 期刊:{{ item.journal_name }}
- 影响因子:{{ item.factor || "--"}}
- 作者:{{ item.authors }}
- 通讯作者:{{ item.author }}
数据更新时间:{{ journalArticles.updateTime }}
{{ item.title }}
- 作者:{{ item.authors }}
数据更新时间:{{ monograph.updateTime }}
{{ item.title }}
- 作者:{{ item.authors }}
数据更新时间:{{ sciAawards.updateTime }}
{{ item.title }}
- 作者:{{ item.authors }}
数据更新时间:{{ conferencePapers.updateTime }}
{{ item.title }}
- 作者:{{ item.authors }}
数据更新时间:{{ patent.updateTime }}
其他文献
微RNA-374c-5p通过靶向LIMK1增强人乳腺癌MDA-MB-231/DDP细胞对顺铂的敏感性
- DOI:--
- 发表时间:2020
- 期刊:肿瘤
- 影响因子:--
- 作者:乔伊娜;文思阳;孙可馨;阴嘉莉;万雪颖;曾欢;杨丽萍;柳满然
- 通讯作者:柳满然
稳定干扰癌相关成纤维细胞中YAP1表达可抑制乳腺癌MDA-MB-231细胞迁移和侵袭
- DOI:--
- 发表时间:2019
- 期刊:肿瘤
- 影响因子:--
- 作者:杜燕娥;李跟友;段 亮;丁小娟;王 玎;文思阳;赵茂嘉;侯懿烜
- 通讯作者:侯懿烜
其他文献
{{
item.title }}
{{ item.translation_title }}
- DOI:{{ item.doi || "--" }}
- 发表时间:{{ item.publish_year || "--"}}
- 期刊:{{ item.journal_name }}
- 影响因子:{{ item.factor || "--" }}
- 作者:{{ item.authors }}
- 通讯作者:{{ item.author }}
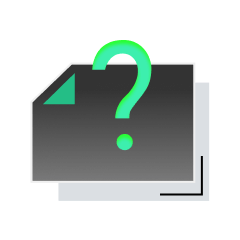
内容获取失败,请点击重试
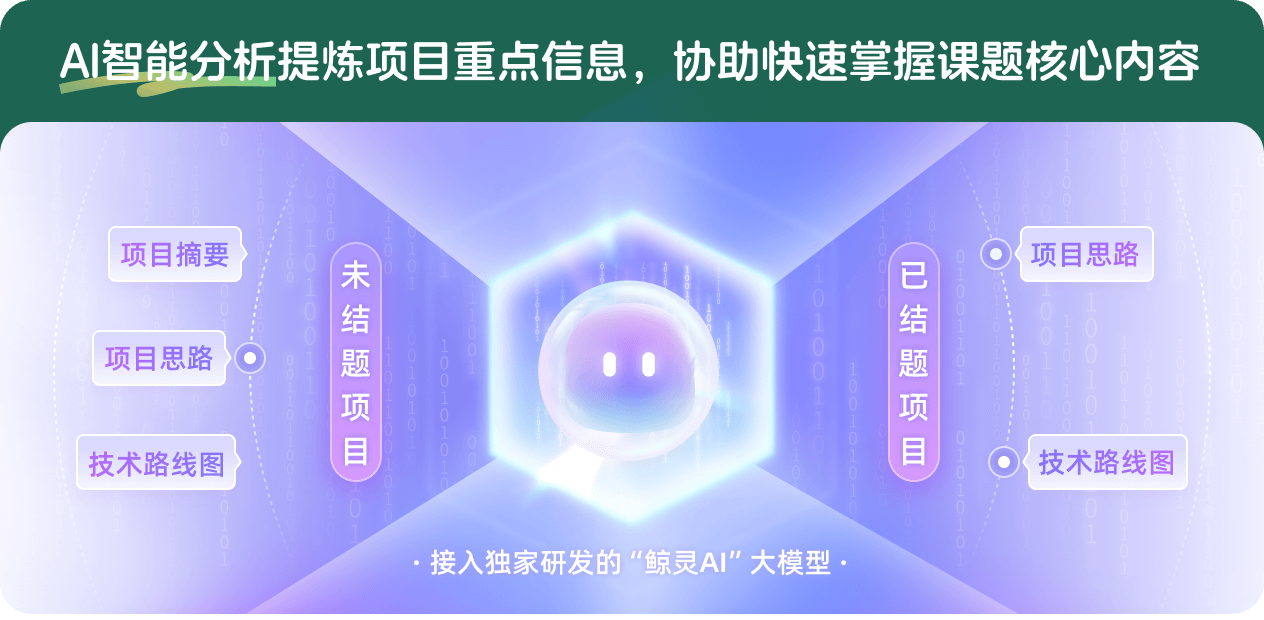
查看分析示例
此项目为未结题,我已根据课题信息分析并撰写以下内容,帮您拓宽课题思路:
AI项目摘要
AI项目思路
AI技术路线图
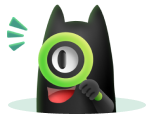
请为本次AI项目解读的内容对您的实用性打分
非常不实用
非常实用
1
2
3
4
5
6
7
8
9
10
您认为此功能如何分析更能满足您的需求,请填写您的反馈:
相似国自然基金
{{ item.name }}
- 批准号:{{ item.ratify_no }}
- 批准年份:{{ item.approval_year }}
- 资助金额:{{ item.support_num }}
- 项目类别:{{ item.project_type }}
相似海外基金
{{
item.name }}
{{ item.translate_name }}
- 批准号:{{ item.ratify_no }}
- 财政年份:{{ item.approval_year }}
- 资助金额:{{ item.support_num }}
- 项目类别:{{ item.project_type }}