空地一体化参考系无关QKD研究
项目介绍
AI项目解读
基本信息
- 批准号:
- 项目类别:面上项目
- 资助金额:57万
- 负责人:
- 依托单位:
- 学科分类:
- 结题年份:
- 批准年份:2021
- 项目状态:未结题
- 起止时间:2021至
- 项目参与者:孙仕海;
- 关键词:
项目摘要
结项摘要
项目成果
期刊论文数量(0)
专著数量(0)
科研奖励数量(0)
会议论文数量(0)
专利数量(0)
数据更新时间:{{ journalArticles.updateTime }}
{{
item.title }}
{{ item.translation_title }}
- DOI:{{ item.doi || "--"}}
- 发表时间:{{ item.publish_year || "--" }}
- 期刊:{{ item.journal_name }}
- 影响因子:{{ item.factor || "--"}}
- 作者:{{ item.authors }}
- 通讯作者:{{ item.author }}
数据更新时间:{{ journalArticles.updateTime }}
{{ item.title }}
- 作者:{{ item.authors }}
数据更新时间:{{ monograph.updateTime }}
{{ item.title }}
- 作者:{{ item.authors }}
数据更新时间:{{ sciAawards.updateTime }}
{{ item.title }}
- 作者:{{ item.authors }}
数据更新时间:{{ conferencePapers.updateTime }}
{{ item.title }}
- 作者:{{ item.authors }}
数据更新时间:{{ patent.updateTime }}
其他文献
Wavelength-selected photon-number-splitting attack against plug-and-play quantum key distribution systems with decoy states(针对诱骗态的即插即用量子密钥分发系统的波长选择的光子数分流攻击)
- DOI:--
- 发表时间:2012
- 期刊:Physical Review A
- 影响因子:2.9
- 作者:江木生;孙仕海;李春燕;梁林梅
- 通讯作者:梁林梅
Partially random phase attack to the practical two-way quantum-key-distribution system(实际双向系统的部分随机相位攻击)
- DOI:--
- 发表时间:2012
- 期刊:Physical Review A
- 影响因子:2.9
- 作者:孙仕海;高明;江木生;李春燕;梁林梅
- 通讯作者:梁林梅
Experimental demonstration of an active phase randomization and monitor module for quantum key distribution(量子密钥分发主动相位随机化和控制模块的实验实现)
- DOI:--
- 发表时间:2012
- 期刊:Applied Physics Letters
- 影响因子:4
- 作者:孙仕海;梁林梅
- 通讯作者:梁林梅
Single-photon-detection attack on the phase-coding continuous-variable quantum cryptography(针对相位编码连续变量量子密码的单光子探测攻击)
- DOI:--
- 发表时间:2012
- 期刊:Physical Review A
- 影响因子:2.9
- 作者:孙仕海;江木生;梁林梅
- 通讯作者:梁林梅
其他文献
{{
item.title }}
{{ item.translation_title }}
- DOI:{{ item.doi || "--" }}
- 发表时间:{{ item.publish_year || "--"}}
- 期刊:{{ item.journal_name }}
- 影响因子:{{ item.factor || "--" }}
- 作者:{{ item.authors }}
- 通讯作者:{{ item.author }}
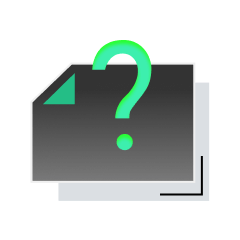
内容获取失败,请点击重试
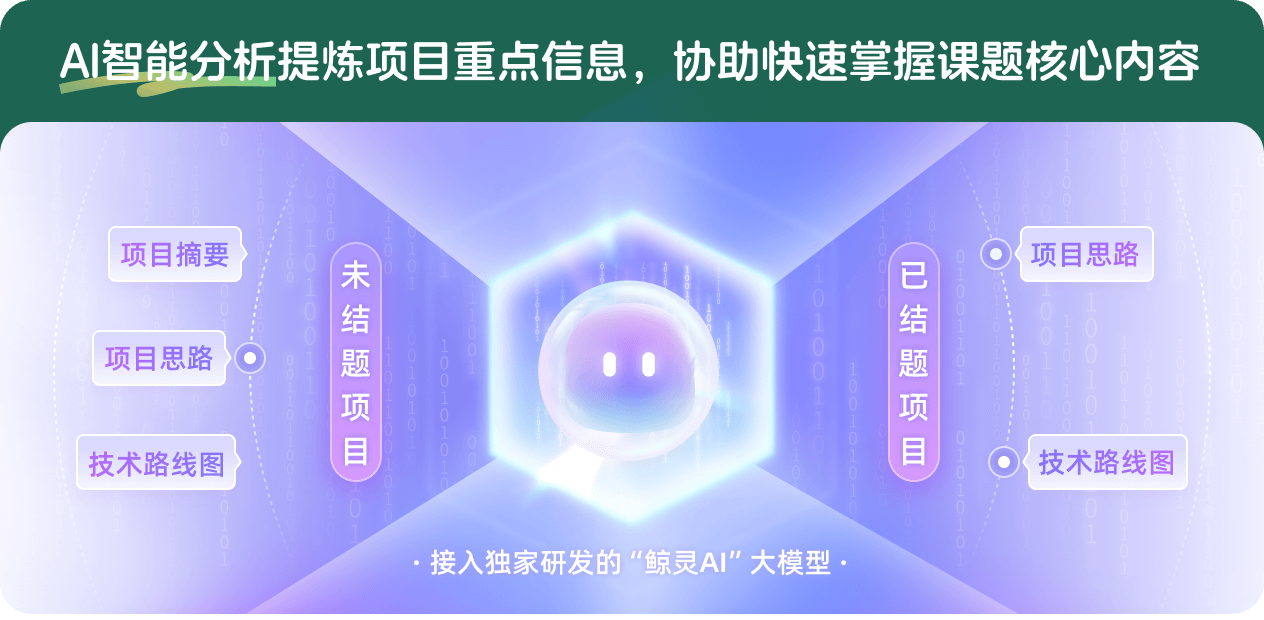
查看分析示例
此项目为未结题,我已根据课题信息分析并撰写以下内容,帮您拓宽课题思路:
AI项目摘要
AI项目思路
AI技术路线图
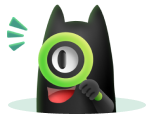
请为本次AI项目解读的内容对您的实用性打分
非常不实用
非常实用
1
2
3
4
5
6
7
8
9
10
您认为此功能如何分析更能满足您的需求,请填写您的反馈:
孙仕海的其他基金
空地一体化参考系无关QKD研究
- 批准号:62171485
- 批准年份:2021
- 资助金额:57.00 万元
- 项目类别:面上项目
实际量子密钥分发系统安全性研究
- 批准号:11674397
- 批准年份:2016
- 资助金额:67.0 万元
- 项目类别:面上项目
相似国自然基金
{{ item.name }}
- 批准号:{{ item.ratify_no }}
- 批准年份:{{ item.approval_year }}
- 资助金额:{{ item.support_num }}
- 项目类别:{{ item.project_type }}
相似海外基金
{{
item.name }}
{{ item.translate_name }}
- 批准号:{{ item.ratify_no }}
- 财政年份:{{ item.approval_year }}
- 资助金额:{{ item.support_num }}
- 项目类别:{{ item.project_type }}