高复杂性脑电数据的数据挖掘及其在癫痫性发作自动检测中的应用
项目介绍
AI项目解读
基本信息
- 批准号:61473223
- 项目类别:面上项目
- 资助金额:81.0万
- 负责人:
- 依托单位:
- 学科分类:F0603.机器学习
- 结题年份:2018
- 批准年份:2014
- 项目状态:已结题
- 起止时间:2015-01-01 至2018-12-31
- 项目参与者:M· Brandon Westover; 贾建; 万青; 王博; 崔新月; 宋江玲; 胡文凤;
- 关键词:
项目摘要
Continuous Electroencephalography monitoring in the Intensive Care Unit environment (ICU-EEG) has become an essential component of top-tier medical care for patients with acute neurological injuries. ICU-EEG data presents a special challenge for computational analysis because of its high complexity, large scale, massive variability, imbalance, and the large number and varied character of both external and physiological background noise that can contaminate signals acquired in the ICU environment. Despite the urgent medical demand for automated analysis of ICU-EEG data, very little research has been done to bring the existing data mining to ICU-EEG data, and to develop the novel theoretical advances that will be needed to cope with the unique challenges posed by mining this data. This project focuses on developing fundamental theory and methods of data mining for such highly complicated EEG data systematically, with a focus on automated detection of epileptic seizures. Firstly, a multi-element feature extraction method will be developed, where 2-D features in frequency domain, linear and nonlinear features in time domain, and the features based on the location of seizure origin and the spatial pattern of seizure spread throughout the brain over time are all extracted and combined. Secondly, a semi-supervised domain transferred (SSDT) learning model will be constructed on the basis of L_0.5 sparse regularization and manifold regularization. Fast algorithms for solving the SSDT model will be designed and the convergence of the algorithms will be analyzed. Thirdly, an ensemble classification system based on boosting and cascaded classifier methods from computer vision will be adapted to epileptic seizure detection in ICU-EEG data. Finally, the application of the automatic epileptic seizure detection will be tested, refined, and validated on a large set of ICU-EEG recordings to verify the feasibility and efficiency of the above constructed system. The project results will provide significant theoretical advances, methods and a system for extracting the essential data features, clarifying the appropriate learning framework for highly complicated biomedical data, and will yield a much needed practical solution to the problem of epileptic seizure detection which can be used to assist physicians in caring for critically ill patients.
面向重症监护癫痫病人的脑电图是一类具有超大规模、多变、非平衡、含复杂噪声的高复杂性脑电数据,有关此类数据的数据挖掘是医学临床诊断的急需,但目前几乎还是空白。本项目拟以癫痫性发作自动检测为背景,聚焦对高复杂性脑电数据数据挖掘的基本理论与方法展开系统研究。拟提出包含二维表示的频域特征、融合线性与非线性的时域特征、以及刻画发作起始位置及其演变过程的空间模式特征的特征提取方法;建立基于L_0.5稀疏正则化理论与流行正则化理论的异域半监督转移学习模型,设计快速求解算法并对其收敛性进行系统分析;构造基于boosting思想及计算机视觉的完整、可解释的综合分类集成系统;以癫痫性发作的自动检测为应用来验证所研制系统的可用性与有效性。项目成果将为高复杂性脑电数据的本质特征提取、高复杂性脑电数据的学习模式挖掘、实用癫痫性发作自动检测系统研发等提供重要的理论、方法与系统基础,亦可直接用于相关医疗诊断的辅助工具。
结项摘要
面向重症监护病人的脑电图是一类具有超大规模、多变、非平衡、含复杂噪声的高复杂性脑电数据,有关此类数据的数据挖掘是医学临床诊断的急需,但目前儿乎还是空白。源于这一现状,本课题以癫痫性发作自动检测为背景,研究了高复杂性脑电数据的综合特征提取方法。分别从时频域分析、非线性动力学分析等不同角度设计了多种新的脑电特征提取方法,并提出了融合癫痫脑电特征,对脑电信号在癫痫发作时的本质变化进行了多方位刻画。研究了混合结构的统一学习模型、算法与理论。构建了相关的神经计算模型并分析其动力学性质,展开了复杂脑电的数据平衡方法以及神经计算模型参数辨识算法的研究,并提出了一种新的神经生理系统复杂性分析框架。研究了综合分类集成系统的实现与关键技术。通过对已有分类技术的综合比较,提出了一种基于超限学习机的分类系统;并结合logistic回归,超限学习机,支撑向量机、决策树,提出了一种基于多混合分类器的综合分类集成系统。研究了所提技术在重症监护病人癫痫性发作自动检测中的应用。建立了典型异常放电模式的脑电数据库以及高复杂性脑电数据库,提出了复杂脑电的伪迹去除方法,并在基于模型和数据共同驱动的癫痫性发作早期检测及发作追踪方面展开了具体的应用研究,取得令人满意的结果。项目成果将为高复杂性脑电数据的本质特征提取与学习模式挖掘、以及脑功能障碍临床辅助诊断系统的实现提供理论依据与技术基础。
项目成果
期刊论文数量(19)
专著数量(0)
科研奖励数量(1)
会议论文数量(0)
专利数量(0)
癫痫脑电的特征提取方法综述
- DOI:10.16152/j.cnki.xdxbzr.2016-06-001
- 发表时间:2016
- 期刊:西北大学学报(自然科学版)
- 影响因子:--
- 作者:张瑞;宋江玲;胡文凤
- 通讯作者:胡文凤
一种新的癫痫脑电融合特征提取方法
- DOI:10.16152/j.cnki.xdxbzr.2016-06-004
- 发表时间:2016
- 期刊:西北大学学报(自然科学版)
- 影响因子:--
- 作者:李艳艳;杨陈军;野梅娜;张瑞
- 通讯作者:张瑞
Automated detection of epileptic EEGs using a novel fusion feature and extreme learning machine
使用新颖的融合功能和极限学习机自动检测癫痫脑电图
- DOI:10.1016/j.neucom.2015.10.070
- 发表时间:2016-01-29
- 期刊:NEUROCOMPUTING
- 影响因子:6
- 作者:Song, Jiang-Ling;Hu, Wenfeng;Zhang, Rui
- 通讯作者:Zhang, Rui
Application of extreme learning machine to epileptic seizure detection based on lagged Poincare plots
极限学习机在基于滞后庞加莱图的癫痫发作检测中的应用
- DOI:10.1007/s11045-016-0419-y
- 发表时间:2017
- 期刊:Multidimensional Systems and Signal Processing
- 影响因子:2.5
- 作者:Song Jiang-Ling;Zhang Rui;Zhang R
- 通讯作者:Zhang R
非平衡数据处理方法在癫痫发作检测中的应用
- DOI:10.16152/j.cnki.xdxbzr.2016-06-002
- 发表时间:2016
- 期刊:西北大学学报(自然科学版)
- 影响因子:--
- 作者:野梅娜;李艳艳;杨陈军;张瑞
- 通讯作者:张瑞
数据更新时间:{{ journalArticles.updateTime }}
{{
item.title }}
{{ item.translation_title }}
- DOI:{{ item.doi || "--"}}
- 发表时间:{{ item.publish_year || "--" }}
- 期刊:{{ item.journal_name }}
- 影响因子:{{ item.factor || "--"}}
- 作者:{{ item.authors }}
- 通讯作者:{{ item.author }}
数据更新时间:{{ journalArticles.updateTime }}
{{ item.title }}
- 作者:{{ item.authors }}
数据更新时间:{{ monograph.updateTime }}
{{ item.title }}
- 作者:{{ item.authors }}
数据更新时间:{{ sciAawards.updateTime }}
{{ item.title }}
- 作者:{{ item.authors }}
数据更新时间:{{ conferencePapers.updateTime }}
{{ item.title }}
- 作者:{{ item.authors }}
数据更新时间:{{ patent.updateTime }}
其他文献
基于熵权-TOPSIS的多能互补联供系统优化配置研究
- DOI:10.13245/j.hust.220204
- 发表时间:2021
- 期刊:华中科技大学学报(自然科学版)
- 影响因子:--
- 作者:张东;张彬;张瑞;安周建
- 通讯作者:安周建
哈尔滨黄土炭屑记录的中晚更新世以来古火事件及其古植被——古气候意义
- DOI:--
- 发表时间:2022
- 期刊:生态学报
- 影响因子:--
- 作者:张瑞;迟云平;谢远云;康春国;吴鹏;孙磊;魏振宇
- 通讯作者:魏振宇
近61年来长江荆南三口水系连通性演变特征
- DOI:--
- 发表时间:2019
- 期刊:长江流域资源与环境
- 影响因子:--
- 作者:李景保;于丹丹;张瑞;杨波;代稳;何蒙;徐志
- 通讯作者:徐志
一种连续的谱聚类优化模型
- DOI:--
- 发表时间:2018
- 期刊:计算数学
- 影响因子:--
- 作者:刘歆;吴国宝;张瑞;张在坤
- 通讯作者:张在坤
A double-layered optimisation approach for the integrated due date assignment and scheduling problem
综合到期日分配和调度问题的双层优化方法
- DOI:10.1080/00207543.2011.571440
- 发表时间:2012-01
- 期刊:International Journal of Production Research
- 影响因子:9.2
- 作者:张瑞
- 通讯作者:张瑞
其他文献
{{
item.title }}
{{ item.translation_title }}
- DOI:{{ item.doi || "--" }}
- 发表时间:{{ item.publish_year || "--"}}
- 期刊:{{ item.journal_name }}
- 影响因子:{{ item.factor || "--" }}
- 作者:{{ item.authors }}
- 通讯作者:{{ item.author }}
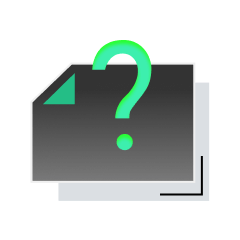
内容获取失败,请点击重试
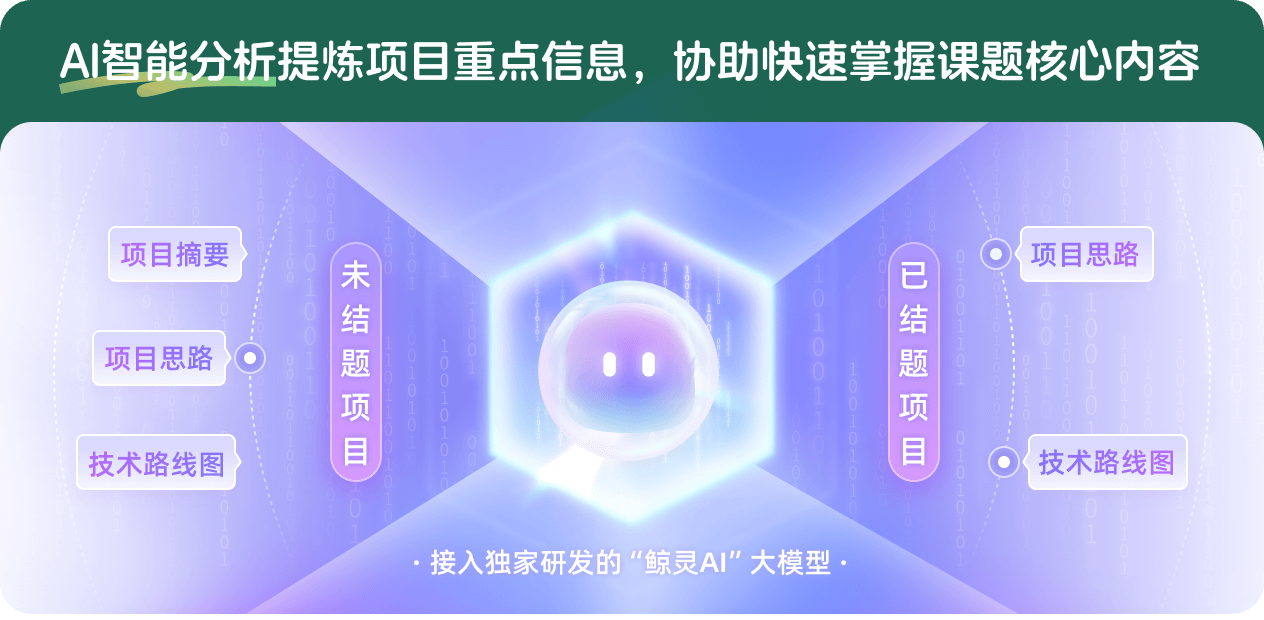
查看分析示例
此项目为已结题,我已根据课题信息分析并撰写以下内容,帮您拓宽课题思路:
AI项目摘要
AI项目思路
AI技术路线图
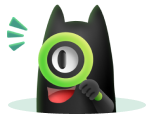
请为本次AI项目解读的内容对您的实用性打分
非常不实用
非常实用
1
2
3
4
5
6
7
8
9
10
您认为此功能如何分析更能满足您的需求,请填写您的反馈:
张瑞的其他基金
关于ELMs技术的理论评价及其在数据挖掘中的应用研究
- 批准号:61075050
- 批准年份:2010
- 资助金额:30.0 万元
- 项目类别:面上项目
相似国自然基金
{{ item.name }}
- 批准号:{{ item.ratify_no }}
- 批准年份:{{ item.approval_year }}
- 资助金额:{{ item.support_num }}
- 项目类别:{{ item.project_type }}
相似海外基金
{{
item.name }}
{{ item.translate_name }}
- 批准号:{{ item.ratify_no }}
- 财政年份:{{ item.approval_year }}
- 资助金额:{{ item.support_num }}
- 项目类别:{{ item.project_type }}