面向大数据高通量仿真的异构计算数据通信建模与优化
项目介绍
AI项目解读
基本信息
- 批准号:61602037
- 项目类别:青年科学基金项目
- 资助金额:20.0万
- 负责人:
- 依托单位:
- 学科分类:F0202.系统软件、数据库与工业软件
- 结题年份:2019
- 批准年份:2016
- 项目状态:已结题
- 起止时间:2017-01-01 至2019-12-31
- 项目参与者:卿杜政; 李凤霞; 何景; 贾娜娜; 王彬; 赵伟;
- 关键词:
项目摘要
Modeling and simulation technology is widely used in military, aerospace, aviation and other important areas. It is a key technology that the U.S. and other developed countries are striving to develop. Big data brings new opportunities and challenges to the simulation technology. CPU and GPU or other heterogeneous many-core processor collaborative computing is considered to be the inevitable trend to solve the big data high-throughput simulation problems. However, the communication bottleneck of cpu/gpu collaborative computing not only decrease the computation performance but also put forward higher requirements on simulation development, thus it becomes a key problem restricting the technology popularization and application.This research focus on the cpu/gpu data communication problem in heterogeneous collaborative computing and investigate the data communication features and data transmission patterns on directive-based mixed code. The research will analyse the data flow characteristics and data communication rules of mixed program contain directives. The data flow aware communication statement migration and asynchronization, optimal communication fusion and hiding will all be studied in the research. Through the modified data flow analysis approach, the data communication model and optimization theory for heterogeneous mixed program will be established, this will lay the theoretical and technical basis for promoting the development and application of big data high-throughput simulation.
建模与仿真技术广泛应用于军事、航天、航空等重要领域,是欧美等发达国家竞相发展的关键技术。大数据给仿真技术带来新的机遇和挑战,CPU与GPU等异构众核处理器协同计算是解决大数据高通量仿真计算问题的必然趋势。然而,CPU/GPU协同计算时的通信瓶颈不仅阻碍其充分发挥计算性能也对仿真开发提出了更高的要求,成为制约该项技术推广应用的关键问题。本项目以CPU/GPU异构协同计算的数据通信为研究对象,对包含编译制导语句的混合程序数据通信特征和数据传输规律进行基础科学研究。具体内容包括:分析包含编译制导语句的混合程序数据流特征和数据通信规律;研究数据流信息感知的通信指令迁移方法与最优化通信融合模型;研究数据流信息感知的通信指令异步化方法与最优通信隐藏机制。通过改造的数据流分析方法,建立面向异构计算的混合程序数据通信模型与优化理论,为推动我国大数据高通量仿真发展与应用奠定理论和技术基础。
结项摘要
高通量仿真是以云计算、大数据和物联网发展为引导的新一代仿真技术,本项目主要关注大数据高通量仿真中异构计算环境的通信和建模优化问题。当仿真系统建立在大数据中心和物联网边缘设备之上时,会面临很多的性能问题,其中最典型的就是与数据相关的传输、存储和执行延迟。本项目主要研究高通量仿真中异构计算环境CPU与GPU协同计算时的数据传输优化;高通量仿真中异构环境数据存储优化以及仿真服务部署优化三个方面的问题。设计了基于数据流特征和数据通信规律的数据传输指令迁移方法和基于核函数数据交换指令合并的数据通信优化方法,将高通量异构计算环境中数据传输平均提高了32.5%。分析和设计了基于线性哈希的动态可扩展高通量仿真小文件存储方法,设计的单级索引结构能够在减少内存消耗的同时提高数据访问性能,其中数据存储性能与原有存储系统HDFS中提供的多种方案相比性能提升数十倍,影响更关键的是数据的随机访问性能,该方法比HDFS性能提升40%,与MapFile相比性能平均提升5倍,比HAR性能平均提升4倍。因此可以看出本项目提出的数据访问方法能够在大数据高通量仿真中起到非常关键的作用,拥有良好的应用前景。提出了基于深度强化学习模型的高通量仿真服务边缘侧部署优化方法,该方法在满足众多约束的前提下将仿真服务尽量部署在边缘侧,使仿真模型和服务生成的数据尽量本地存储和处理,在必要的时候经过网络存储到云中,这样进一步的减少了数据通信,提高仿真的执行性能。实验效果良好,与现有最好方法相比,本项目提出的方法可以将在本地执行的仿真服务数量提高13.4%,总体响应时间提高5%。综上所述,本项目在几个方面的研究成果能够有效的提高高通量仿真的性能,在数据传输、存储与执行方面取得了良好的效果,对于推动我国大数据高通量仿真的发展具有推动意义。
项目成果
期刊论文数量(5)
专著数量(0)
科研奖励数量(0)
会议论文数量(4)
专利数量(1)
基于微服务的边缘侧仿真方法及框架研究
- DOI:10.16182/j.issn1004731x.joss.201812006
- 发表时间:2018
- 期刊:系统仿真学报
- 影响因子:--
- 作者:翟岩龙;孙文心;包天虹;杨凯;卿杜政
- 通讯作者:卿杜政
Efficient State Management for Scaling Out Stateful Operators in Stream Processing Systems
在流处理系统中横向扩展有状态算子的高效状态管理
- DOI:10.1089/big.2018.0093
- 发表时间:2019-09
- 期刊:Big Data
- 影响因子:4.6
- 作者:Mudassar Muhammad;Zhai Yanlong;Liao Lejian
- 通讯作者:Liao Lejian
A weakly supervised method for makeup-invariant face verification
一种弱监督的化妆不变人脸验证方法
- DOI:10.1016/j.patcog.2017.01.011
- 发表时间:2017-06
- 期刊:Pattern Recognition
- 影响因子:8
- 作者:YaoSun;Lejian Ren;Zhen Wei;Bin Liu;Yanlong Zhai;Si Liu i
- 通讯作者:Si Liu i
高通量仿真中数据存储与访问技术研究
- DOI:10.16182/j.issn1004731x.joss.201709019
- 发表时间:2017
- 期刊:系统仿真学报
- 影响因子:--
- 作者:王子硕;翟岩龙;陶文俊;杨浩;张晗;卿杜政
- 通讯作者:卿杜政
数据更新时间:{{ journalArticles.updateTime }}
{{
item.title }}
{{ item.translation_title }}
- DOI:{{ item.doi || "--"}}
- 发表时间:{{ item.publish_year || "--" }}
- 期刊:{{ item.journal_name }}
- 影响因子:{{ item.factor || "--"}}
- 作者:{{ item.authors }}
- 通讯作者:{{ item.author }}
数据更新时间:{{ journalArticles.updateTime }}
{{ item.title }}
- 作者:{{ item.authors }}
数据更新时间:{{ monograph.updateTime }}
{{ item.title }}
- 作者:{{ item.authors }}
数据更新时间:{{ sciAawards.updateTime }}
{{ item.title }}
- 作者:{{ item.authors }}
数据更新时间:{{ conferencePapers.updateTime }}
{{ item.title }}
- 作者:{{ item.authors }}
数据更新时间:{{ patent.updateTime }}
其他文献
其他文献
{{
item.title }}
{{ item.translation_title }}
- DOI:{{ item.doi || "--" }}
- 发表时间:{{ item.publish_year || "--"}}
- 期刊:{{ item.journal_name }}
- 影响因子:{{ item.factor || "--" }}
- 作者:{{ item.authors }}
- 通讯作者:{{ item.author }}
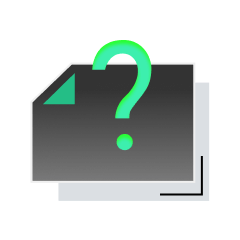
内容获取失败,请点击重试
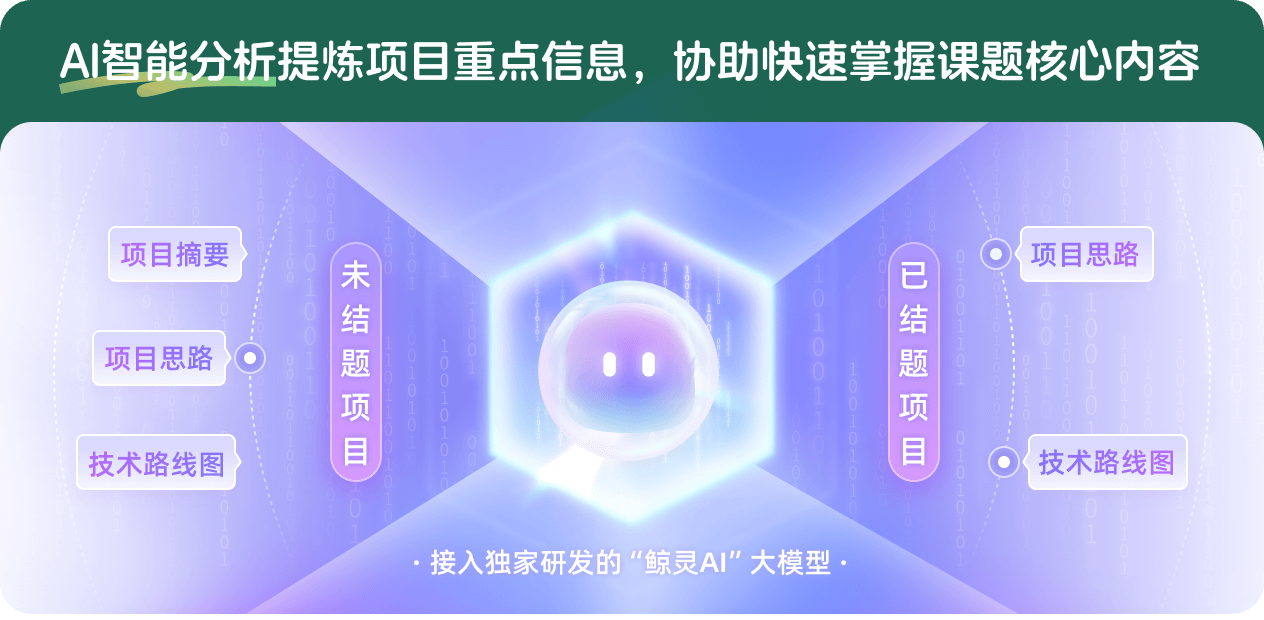
查看分析示例
此项目为已结题,我已根据课题信息分析并撰写以下内容,帮您拓宽课题思路:
AI项目摘要
AI项目思路
AI技术路线图
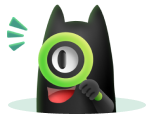
请为本次AI项目解读的内容对您的实用性打分
非常不实用
非常实用
1
2
3
4
5
6
7
8
9
10
您认为此功能如何分析更能满足您的需求,请填写您的反馈:
相似国自然基金
{{ item.name }}
- 批准号:{{ item.ratify_no }}
- 批准年份:{{ item.approval_year }}
- 资助金额:{{ item.support_num }}
- 项目类别:{{ item.project_type }}
相似海外基金
{{
item.name }}
{{ item.translate_name }}
- 批准号:{{ item.ratify_no }}
- 财政年份:{{ item.approval_year }}
- 资助金额:{{ item.support_num }}
- 项目类别:{{ item.project_type }}