Ghrelin调节Wnt/β-catenin 促进中脑神经干细胞定向分化治疗帕金森病的机制研究
项目介绍
AI项目解读
基本信息
- 批准号:31500837
- 项目类别:青年科学基金项目
- 资助金额:19.0万
- 负责人:
- 依托单位:
- 学科分类:C0901.分子与细胞神经生物学
- 结题年份:2018
- 批准年份:2015
- 项目状态:已结题
- 起止时间:2016-01-01 至2018-12-31
- 项目参与者:宋宁; 荆昭; 王维维; 贾凤菊; 宫冰; 刘志国; 唐婷婷;
- 关键词:
项目摘要
Neural stem cells (NSCs) transplantation is widely used in Parkinson’s disease therapy research. However, the main questions of transplanted NSCs are low survival rate and low differentiation rate of dopaminergic neurons (DA neurons). Ghrelin has neuroprotective effect and can enhance neurogenesis in adult. Moreover, another important role of ghrelin in nervous system is to influence the differentiation of DA neurons. Our previous studies showed that ghrelin could enhance the differentiation of midbrain NSCs to DA neurons, and also could up regulate mRNA expressions of Wnt1 and Wnt3a. In order to illustrate the underlying molecular mechanism, in the present study, we detected the differentiation behaviors changes of midbrain NSCs after treated by ghrelin. Meanwhile, the protein expressions changes of Wnt/β-catenin signaling pathway in differentiating DA neurons were detected to reflect the relationship between Wnt and ghrelin. Furthermore, we observed the effect of transplanted midbrain NSCs which induced by ghrelin in PD animal models. This project will investigate the effect of ghrelin on NSCs behaviors and the related mechanism; evaluate the efficacy of ghrelin on NSCs transplantation therapy strategy in PD. We hope that this study could supply some new evidences for NSCs transplantation theory of PD therapy, NSCs induced differentiations and the effect of ghrelin on neuroregeneration.
神经干细胞(NSCs)移植已广泛应用于帕金森病(PD)治疗的研究,但移植的NSCs面临着存活率低和向多巴胺(DA)能神经元分化少的问题。研究发现ghrelin可促进NSCs增殖,影响其向DA能神经元分化,但机制不明。我们前期研究发现ghrelin可提高中脑NSCs向DA能神经元的分化比例,促进Wnt1和Wnt3a的表达。为了研究ghrelin对中脑NSCs向DA能分化的作用机制,本研究利用NSCs培养和细胞移植等方法,在细胞和组织水平观察ghrelin对NSCs向DA能神经元分化程度的影响,检测不同分化时间点,DA能神经元中Wnt1和Wnt3a表达量,及Wnt/β-catenin通路蛋白表达及磷酸化程度;最后将ghrelin基因修饰的NSCs移植于PD动物模型上,观察DA能神经元的存活、DA释放和动物运动行为改善的情况。本项目将为NSCs的定向诱导并移植治疗PD提供一定的实验依据。
结项摘要
Ghrelin对多巴胺(DA)能神经元具有保护作用,研究发现ghrelin可促进NSCs增殖,影响神经元分化,但其对中脑神经干细胞(NSCs)的作用和机制不明。本研究首先观察了ghrelin对中脑NSCs增殖、分化和迁移等生物学行为的影响,发现ghrelin可通过促进中脑NSCs的增殖和向神经元分化等作用促进神经再生。我们也发现ghrelin可促进中脑NSCs向DA能神经元分化,并对其机制进行探讨。本研究观察到ghrelin处理后中脑NSCs 胞内 Wnt1和Wnt3a表达水平增加,并阐明了ghrelin对DA能神经元分化的影响是通过调控Wnt/ß-catenin信号实现。本项目将为NSCs的定向诱导移植治疗PD和ghrelin作为神经保护药物的开发,提供一定的实验依据。
项目成果
期刊论文数量(9)
专著数量(0)
科研奖励数量(0)
会议论文数量(3)
专利数量(0)
Biometal Dyshomeostasis and Toxic Metal Accumulations in the Development of Alzheimer's Disease.
阿尔茨海默病发展过程中的生物金属动态平衡失调和有毒金属积累
- DOI:10.3389/fnmol.2017.00339
- 发表时间:2017
- 期刊:Frontiers in molecular neuroscience
- 影响因子:4.8
- 作者:Li Y;Jiao Q;Xu H;Du X;Shi L;Jia F;Jiang H
- 通讯作者:Jiang H
Cell-Cell Connection Enhances Proliferation and Neuronal Differentiation of Rat Embryonic Neural Stem/Progenitor Cells.
细胞间连接增强大鼠胚胎神经干/祖细胞的增殖和神经元分化
- DOI:10.3389/fncel.2017.00200
- 发表时间:2017
- 期刊:Frontiers in cellular neuroscience
- 影响因子:5.3
- 作者:Jiao Q;Li X;An J;Zhang Z;Chen X;Tan J;Zhang P;Lu H;Liu Y
- 通讯作者:Liu Y
Oxidative Stress Regulated Iron Regulatory Protein IRP2 Through FBXL5-Mediated Ubiquitination-Proteasome Way in SH-SY5Y Cells
SH-SY5Y细胞中氧化应激通过FBXL5介导的泛素化-蛋白酶体途径调节铁调节蛋白IRP2
- DOI:10.3389/fnins.2019.00020
- 发表时间:2019-01-29
- 期刊:FRONTIERS IN NEUROSCIENCE
- 影响因子:4.3
- 作者:Jiao, Qian;Du, Xixun;Jiang, Hong
- 通讯作者:Jiang, Hong
自噬在神经干细胞中作用的研究进展
- DOI:--
- 发表时间:2016
- 期刊:生理学报
- 影响因子:--
- 作者:焦倩;杜希恂;谢俊霞;姜宏
- 通讯作者:姜宏
The neurological effects of ghrelin in brain diseases: Beyond metabolic functions
生长素释放肽对脑部疾病的神经影响:超越代谢功能
- DOI:10.1016/j.neubiorev.2016.12.010
- 发表时间:2017-02-01
- 期刊:NEUROSCIENCE AND BIOBEHAVIORAL REVIEWS
- 影响因子:8.2
- 作者:Jiao, Qian;Du, Xixun;Jiang, Hong
- 通讯作者:Jiang, Hong
数据更新时间:{{ journalArticles.updateTime }}
{{
item.title }}
{{ item.translation_title }}
- DOI:{{ item.doi || "--"}}
- 发表时间:{{ item.publish_year || "--" }}
- 期刊:{{ item.journal_name }}
- 影响因子:{{ item.factor || "--"}}
- 作者:{{ item.authors }}
- 通讯作者:{{ item.author }}
数据更新时间:{{ journalArticles.updateTime }}
{{ item.title }}
- 作者:{{ item.authors }}
数据更新时间:{{ monograph.updateTime }}
{{ item.title }}
- 作者:{{ item.authors }}
数据更新时间:{{ sciAawards.updateTime }}
{{ item.title }}
- 作者:{{ item.authors }}
数据更新时间:{{ conferencePapers.updateTime }}
{{ item.title }}
- 作者:{{ item.authors }}
数据更新时间:{{ patent.updateTime }}
其他文献
红景天苷通过抑制凋亡相关蛋白的表达保护缺氧对培养神经干细胞的损伤
- DOI:--
- 发表时间:2013
- 期刊:南方医科大学学报
- 影响因子:--
- 作者:祁存芳;张军峰;陈新林;张建水;杨蓬勃;焦倩;张蓬勃;吕海侠;刘勇
- 通讯作者:刘勇
Early low-dose ghrelin intervention via mini-osmotic pumps could protect against the progressive dopaminergic neuron loss in Parkinson’s disease mice
通过微型渗透泵进行早期低剂量生长素释放肽干预可以防止帕金森病小鼠的进行性多巴胺能神经元丧失
- DOI:10.21203/rs.3.rs-30892/v1
- 发表时间:2020-06
- 期刊:Neurology of Aging
- 影响因子:--
- 作者:焦玲玲;杜希恂;贾凤菊;李勇;朱德晓;唐婷婷;焦倩;姜宏
- 通讯作者:姜宏
ghrelin对体外培养中脑神经干细胞细胞活力的影响
- DOI:--
- 发表时间:2016
- 期刊:青岛大学医学院学报
- 影响因子:--
- 作者:宫冰;焦倩;杜希恂;姜宏
- 通讯作者:姜宏
基于CiteSpace可视化分析针刺抗抑郁研究热点和趋势
- DOI:--
- 发表时间:2022
- 期刊:中医药导报
- 影响因子:--
- 作者:孟超;图娅;李军;焦倩;谭雨晴;姜会梨
- 通讯作者:姜会梨
PHBHHx 膜表面亲水改性及其与神经干细胞生物相容性
- DOI:--
- 发表时间:2012
- 期刊:生物工程学报
- 影响因子:--
- 作者:焦倩;陈新林;王媛媛;张雅利
- 通讯作者:张雅利
其他文献
{{
item.title }}
{{ item.translation_title }}
- DOI:{{ item.doi || "--" }}
- 发表时间:{{ item.publish_year || "--"}}
- 期刊:{{ item.journal_name }}
- 影响因子:{{ item.factor || "--" }}
- 作者:{{ item.authors }}
- 通讯作者:{{ item.author }}
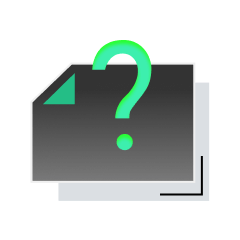
内容获取失败,请点击重试
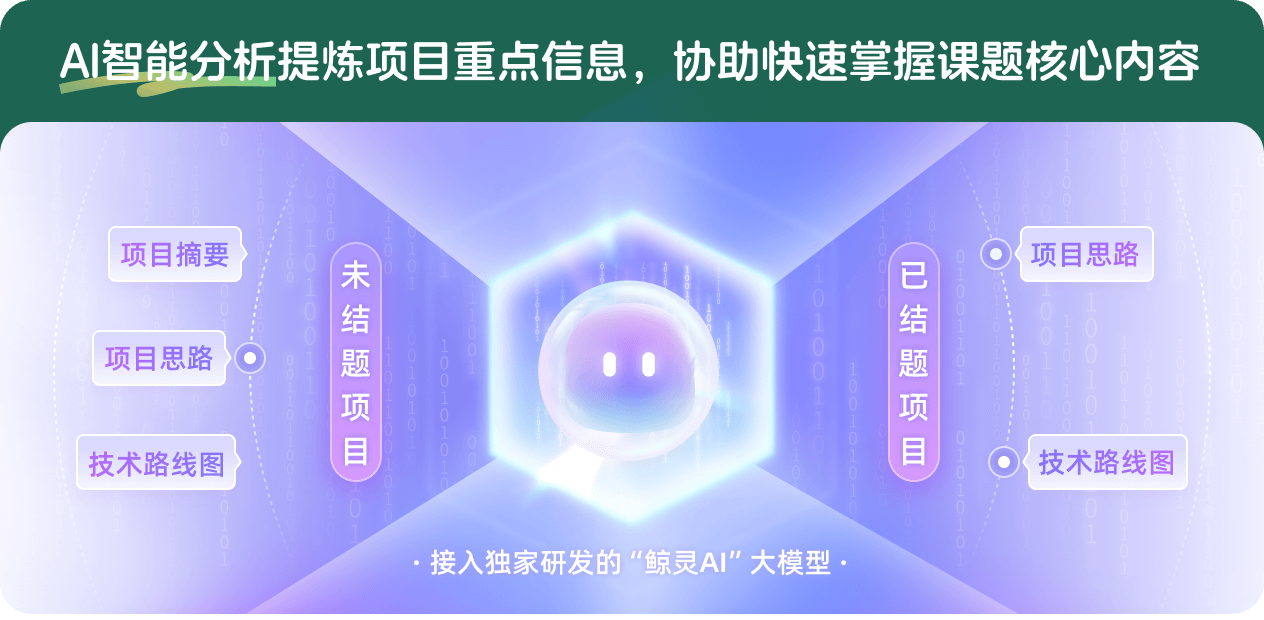
查看分析示例
此项目为已结题,我已根据课题信息分析并撰写以下内容,帮您拓宽课题思路:
AI项目摘要
AI项目思路
AI技术路线图
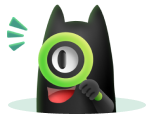
请为本次AI项目解读的内容对您的实用性打分
非常不实用
非常实用
1
2
3
4
5
6
7
8
9
10
您认为此功能如何分析更能满足您的需求,请填写您的反馈:
焦倩的其他基金
OTUD3通过PTEN信号通路调节帕金森病神经发生的机制研究
- 批准号:82071429
- 批准年份:2020
- 资助金额:55 万元
- 项目类别:面上项目
相似国自然基金
{{ item.name }}
- 批准号:{{ item.ratify_no }}
- 批准年份:{{ item.approval_year }}
- 资助金额:{{ item.support_num }}
- 项目类别:{{ item.project_type }}
相似海外基金
{{
item.name }}
{{ item.translate_name }}
- 批准号:{{ item.ratify_no }}
- 财政年份:{{ item.approval_year }}
- 资助金额:{{ item.support_num }}
- 项目类别:{{ item.project_type }}