利用信息处理的观点探究视网膜层次化结构的组织原理
项目介绍
AI项目解读
基本信息
- 批准号:61403375
- 项目类别:青年科学基金项目
- 资助金额:23.0万
- 负责人:
- 依托单位:
- 学科分类:F0609.认知与神经科学启发的人工智能
- 结题年份:2017
- 批准年份:2014
- 项目状态:已结题
- 起止时间:2015-01-01 至2017-12-31
- 项目参与者:余淼; 王伟; 张铁林; 崔海楠; 傅康平; 张婷;
- 关键词:
项目摘要
The information processing in retina is a typically feed-forward and hierarchical procedure. Visual stimuli pass from the photoreceptor to bipolar cell, and then go to ganglion cell. Along this way, the number of relevant neurons reduces to 1.2 million from 150 million. Why does such a hierarchical structure exist in the retina? Does this hierarchical structure meet with "efficient coding" under the principle of energy minimization? In addition to the serially hierarchical structure, there also exist two parallel photo-sensory systems in the retina: rod system and cone system, which have different but complementary structures and functions. What is the principle of this kind of organizational structure? This proposal aims to study these questions from an information-processing viewpoint. The main research topics contain: 1) research on the principle of the retina’s hierarchical organization; 2) research on the theoretical models of the parallel organization consisting of rod system and cone system which are existed in the retina. The research results would not only be valuable in explaining the principles of the retina’s inherent hierarchical structure, but also provide a solid reference for explaining why the deep neural network can exhibit excellent performance in many traditional computer vision tasks, such as object classification and recognition. Finally,as an application of the theoretical studies of the principle of retina’s hierarchical structure, this proposal would use the research results of the first two topics to build and learn adaptive deep neural networks for a better performance.
视网膜的信息处理过程是一种典型的前馈层次化方式,视觉信号逐次经过感光细胞、双极细胞、神经节细胞进行处理,神经元的数量也从1.5亿多个压缩到120多万个,视网膜中为什么会存在这种层次化结构?这种层次化结构是否满足能量最小化准测下的“有效编码”原理?此外,在视网膜的这种层次化结构中,还存在两类并行的感光系统:视杆系统和视锥系统,这两类系统的结构和功能迥异而又互补,这其中的组织原理又是什么?本项目将从信息处理的观点对这些问题进行探究,主要内容包括:1)研究视网膜层次化结构的组织原理;2)研究视网膜中存在视杆和视锥两个子系统的组织原理。这些研究内容不仅能够解释视网膜层次化结构的内在机理,而且能够为解释深度神经网络在物体分类和识别等方面呈现出的优良性质提供有力参考和借鉴。作为理论成果的应用,本项目还将把前两项研究内容的成果应用在自适应深度神经网络的构建与学习中,以提高深度神经网络的性能。
结项摘要
包含视网膜在内的早期视觉系统的信息处理过程是一种典型的前馈层次化方式,为什么会存在这种层次化结构?这种层次化结构是否满足能量最小化准测下的“有效编码”原理?此外,除了这种层次化结构中,单个区域内神经元群的组织结构的存在内在机理是什么?本项目将从信息处理的观点对这些问题进行探究,主要内容包括:1)研究早期视觉系统的层次化结构的组织原理;2)研究单个区域内神经元群的组织结构。这些研究内容不仅能够解释了早期视觉系统层次化结构的内在机理,而且能够为解释深度神经网络在物体分类等方面呈现出的优良性质提供有力参考和借鉴。作为理论成果的应用,本项目还将把前两项研究内容的成果应用在深度神经网络的构建与学习中,提高了深度神经网络在物体分类、语义分割等领域的性能。本项目在执行期内,共计发表国际期刊论文5篇,国际会议论文4篇,申请发明专利4项;项目负责人由助理研究员升聘为副研究员,培养博士研究生4名。
项目成果
期刊论文数量(5)
专著数量(0)
科研奖励数量(0)
会议论文数量(4)
专利数量(4)
Aggregating Rich Hierarchical Features for Scene Classification in Remote Sensing Imagery
聚合丰富的层次特征以进行遥感图像的场景分类
- DOI:10.1109/jstars.2017.2705419
- 发表时间:2017-05
- 期刊:IEEE Journal of Selected Topics in Applied Earth Observations and Remote Sensing
- 影响因子:5.5
- 作者:Guoli Wang;Bin Fan;Shiming Xiang;Chunhong Pan
- 通讯作者:Chunhong Pan
Semantic labeling in very high resolution images via a self-cascaded convolutional neural network
通过自级联卷积神经网络对超高分辨率图像进行语义标记
- DOI:10.1016/j.isprsjprs.2017.12.007
- 发表时间:2018-11-01
- 期刊:ISPRS JOURNAL OF PHOTOGRAMMETRY AND REMOTE SENSING
- 影响因子:12.7
- 作者:Liu, Yongcheng;Fan, Bin;Pan, Chunhong
- 通讯作者:Pan, Chunhong
数据更新时间:{{ journalArticles.updateTime }}
{{
item.title }}
{{ item.translation_title }}
- DOI:{{ item.doi || "--"}}
- 发表时间:{{ item.publish_year || "--" }}
- 期刊:{{ item.journal_name }}
- 影响因子:{{ item.factor || "--"}}
- 作者:{{ item.authors }}
- 通讯作者:{{ item.author }}
数据更新时间:{{ journalArticles.updateTime }}
{{ item.title }}
- 作者:{{ item.authors }}
数据更新时间:{{ monograph.updateTime }}
{{ item.title }}
- 作者:{{ item.authors }}
数据更新时间:{{ sciAawards.updateTime }}
{{ item.title }}
- 作者:{{ item.authors }}
数据更新时间:{{ conferencePapers.updateTime }}
{{ item.title }}
- 作者:{{ item.authors }}
数据更新时间:{{ patent.updateTime }}
其他文献
其他文献
{{
item.title }}
{{ item.translation_title }}
- DOI:{{ item.doi || "--" }}
- 发表时间:{{ item.publish_year || "--"}}
- 期刊:{{ item.journal_name }}
- 影响因子:{{ item.factor || "--" }}
- 作者:{{ item.authors }}
- 通讯作者:{{ item.author }}
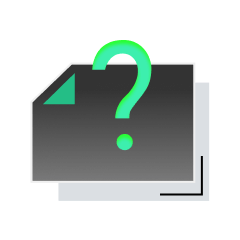
内容获取失败,请点击重试
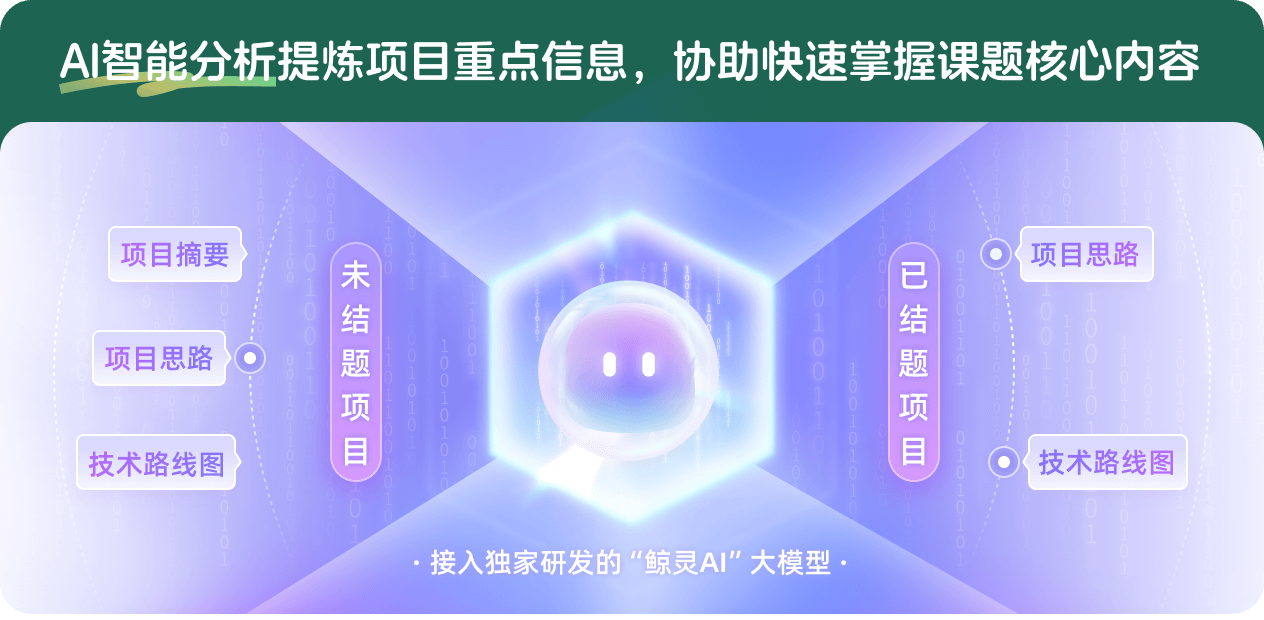
查看分析示例
此项目为已结题,我已根据课题信息分析并撰写以下内容,帮您拓宽课题思路:
AI项目摘要
AI项目思路
AI技术路线图
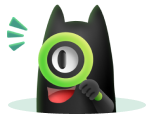
请为本次AI项目解读的内容对您的实用性打分
非常不实用
非常实用
1
2
3
4
5
6
7
8
9
10
您认为此功能如何分析更能满足您的需求,请填写您的反馈:
孔庆群的其他基金
基于大脑组织结构和神经回放多样性的连续学习方法研究
- 批准号:62372453
- 批准年份:2023
- 资助金额:50.00 万元
- 项目类别:面上项目
相似国自然基金
{{ item.name }}
- 批准号:{{ item.ratify_no }}
- 批准年份:{{ item.approval_year }}
- 资助金额:{{ item.support_num }}
- 项目类别:{{ item.project_type }}
相似海外基金
{{
item.name }}
{{ item.translate_name }}
- 批准号:{{ item.ratify_no }}
- 财政年份:{{ item.approval_year }}
- 资助金额:{{ item.support_num }}
- 项目类别:{{ item.project_type }}