鲁棒低秩张量恢复问题的非凸算法研究
项目介绍
AI项目解读
基本信息
- 批准号:11901600
- 项目类别:青年科学基金项目
- 资助金额:27.1万
- 负责人:
- 依托单位:
- 学科分类:A0405.连续优化
- 结题年份:2022
- 批准年份:2019
- 项目状态:已结题
- 起止时间:2020-01-01 至2022-12-31
- 项目参与者:--
- 关键词:
项目摘要
Tensor-based modeling and computation have emerged and drawn a lot of attentions with the advent of big data era and the demands for high dimensional data processing. Robust low-rank tensor recovery problem, also known as tensor or higher order robust principle component analysis, is one kind of tensor optimization problems which recovers tensors with low-rank and sparse structure from limited measurements. It has a wide range of applications in data mining, machine learning, image processing, collaborative filtering, computer vision and so on. This project aims to study the non-convex algorithms for robust low-rank tensor recovery problem, which mainly includes the following three parts: The first is to introduce the non-convex relaxation models for robust low-rank tensor recovery problem, and design highly efficient and robust algorithms for the obtained large scale non-convex tensor optimization problem. The second is to establish the convergence analysis for the proposed algorithms, as well as the exact recovery theory of the non-convex relaxation models for recovering low-rank and sparse tensors. The third is to conduct numerical experiments on synthetic data, and apply the proposed algorithms in image processing, computer vision and other practical problems. The project is of important significance in both academic researches and practical applications, since it not only gives a new idea to the study on algorithms for robust low-rank tensor recovery problem, promotes the developments of tensor computation, tensor optimization and non-convex programming, but also provides theoretical and methodological support for solving the practical problems in real-world applications.
随着大数据时代的来临及处理高维数据的实际需求,基于张量的建模与计算应运而生并受到广泛关注。鲁棒低秩张量恢复问题作为一类刻画张量低秩性及稀疏性的张量优化问题,在数据挖掘、机器学习、图像处理、协同过滤和计算机视觉等领域都具有重要的应用。本项目将考察该问题的非凸算法研究,主要内容包括:第一是针对鲁棒低秩张量恢复问题构建非凸松弛模型,并设计高效稳定、适合求解大规模问题的数值算法;第二是针对所提的算法建立收敛性分析,及针对相关的非凸松弛模型建立精确恢复理论;第三是进行数值实验,并根据实际问题的特性将所获的研究成果应用到图像处理、计算机视觉等应用领域。本项目不仅为鲁棒低秩张量恢复问题的算法研究提供了新的思路,而且能够促进张量计算、张量优化与非凸规划的发展,并为相关应用领域中实际问题的研究提供有效的理论支撑和求解保障,具有重要的学术研究意义和实际应用价值。
结项摘要
项目以结构张量相关优化问题为中心进行了一系列的相关研究。研究的主要内容为低秩张量恢复问题的非凸算法及其收敛性分析、张量互补问题的解集理论及其稀疏解和极小元解的快速求解算法与理论分析。第一,项目在Tucker秩框架下建立了张量鲁棒主成分分析的非凸模型,设计邻近交替线性极小化算法和参数自适应学习的非凸交替方向法,并进行理论分析与数值实验。第二,项目建立了闭凸锥上共正张量互补问题解的可行性与可解性之间的等价条件,并弱化了该问题的可解性条件;提出求解隐式Z张量互补问题的单调下降迭代算法,并建立其全局线性收敛率;设计具有有限终止性的低维方法求解广义Z张量定义的齐次多项式不等式组的极小元,并建立极小元的存在性结果等。
项目成果
期刊论文数量(4)
专著数量(0)
科研奖励数量(0)
会议论文数量(0)
专利数量(0)
Two methods for finding a sparse solution of the linear complementarity problem with a Z-matrix
寻找 Z 矩阵线性互补问题稀疏解的两种方法
- DOI:--
- 发表时间:--
- 期刊:Pacific Journal of Optimization
- 影响因子:0.2
- 作者:Yu-Fan Li;Zheng-Hai Huang;Nana Dai
- 通讯作者:Nana Dai
Three classes of copositive-type tensors and tensor complementarity problems
三类余型张量及张量互补问题
- DOI:--
- 发表时间:2021
- 期刊:Pacific Journal of Optimization
- 影响因子:0.2
- 作者:Zhang Ting;Huang Zheng-Hai;Li Yu-Fan
- 通讯作者:Li Yu-Fan
A fixed point iterative method for tensor complementarity problems with the implicit Z-tensors
隐式 Z 张量张量互补问题的不动点迭代方法
- DOI:10.1007/s10898-022-01263-8
- 发表时间:2022-12
- 期刊:Journal of Global Optimization
- 影响因子:1.8
- 作者:Zheng-Hai Huang;Yu-Fan Li;Yong Wang
- 通讯作者:Yong Wang
数据更新时间:{{ journalArticles.updateTime }}
{{
item.title }}
{{ item.translation_title }}
- DOI:{{ item.doi || "--"}}
- 发表时间:{{ item.publish_year || "--" }}
- 期刊:{{ item.journal_name }}
- 影响因子:{{ item.factor || "--"}}
- 作者:{{ item.authors }}
- 通讯作者:{{ item.author }}
数据更新时间:{{ journalArticles.updateTime }}
{{ item.title }}
- 作者:{{ item.authors }}
数据更新时间:{{ monograph.updateTime }}
{{ item.title }}
- 作者:{{ item.authors }}
数据更新时间:{{ sciAawards.updateTime }}
{{ item.title }}
- 作者:{{ item.authors }}
数据更新时间:{{ conferencePapers.updateTime }}
{{ item.title }}
- 作者:{{ item.authors }}
数据更新时间:{{ patent.updateTime }}
其他文献
其他文献
{{
item.title }}
{{ item.translation_title }}
- DOI:{{ item.doi || "--" }}
- 发表时间:{{ item.publish_year || "--"}}
- 期刊:{{ item.journal_name }}
- 影响因子:{{ item.factor || "--" }}
- 作者:{{ item.authors }}
- 通讯作者:{{ item.author }}
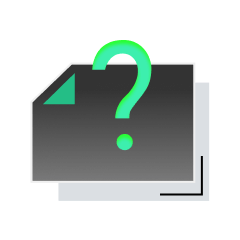
内容获取失败,请点击重试
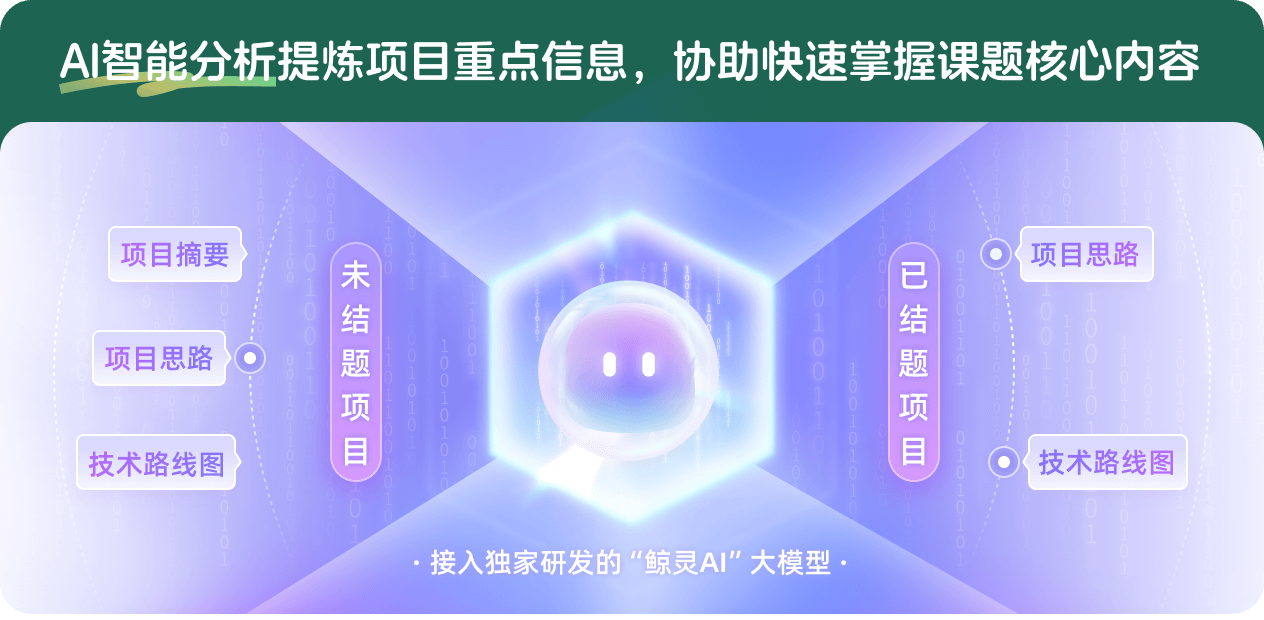
查看分析示例
此项目为已结题,我已根据课题信息分析并撰写以下内容,帮您拓宽课题思路:
AI项目摘要
AI项目思路
AI技术路线图
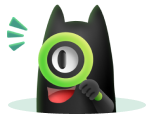
请为本次AI项目解读的内容对您的实用性打分
非常不实用
非常实用
1
2
3
4
5
6
7
8
9
10
您认为此功能如何分析更能满足您的需求,请填写您的反馈:
相似国自然基金
{{ item.name }}
- 批准号:{{ item.ratify_no }}
- 批准年份:{{ item.approval_year }}
- 资助金额:{{ item.support_num }}
- 项目类别:{{ item.project_type }}
相似海外基金
{{
item.name }}
{{ item.translate_name }}
- 批准号:{{ item.ratify_no }}
- 财政年份:{{ item.approval_year }}
- 资助金额:{{ item.support_num }}
- 项目类别:{{ item.project_type }}