实时数据流处理系统中负载均衡与容错机制的融合方法研究
项目介绍
AI项目解读
基本信息
- 批准号:61802273
- 项目类别:青年科学基金项目
- 资助金额:25.0万
- 负责人:
- 依托单位:
- 学科分类:F0202.系统软件、数据库与工业软件
- 结题年份:2021
- 批准年份:2018
- 项目状态:已结题
- 起止时间:2019-01-01 至2021-12-31
- 项目参与者:陈伟; 赵艳; 王怡婷; 单双利; 胡嘉欣; 何芙珍;
- 关键词:
项目摘要
The data are time-sensitive in many areas, which means the data are valuable only when they are in the "fresh" state. In the recent years, the research on Distributed Stream Processing Engine(DSPE), which is used for processing large-scale real-time data, has become an indispensable part in the development of data science and big data technology. However, the intrinsic characteristics of stream data, such as its continuity, dynamic, and unpredictability, bring great challenges in the design of a high-performance and high-availability DSPE. .This project focus on the current issues in DSPE, such as the lack of algorithm which produces a node-wise lightweight adaptive workload balancing, the high cost of resilient processing architecture and the high cost of fault tolerance, pursuing a win-win fusion strategy of load-balancing and fault-tolerance mechanism. In particular, our research in this project will include the following several aspects: (1)A fast and data position sensitive workload balance theory according to the incoming data and task instances; (2)A light-weight workload deployment and adjustment strategy in the fusion method of load-balancing and fault-tolerance mechanism under dynamic workload; (3) The data backup and recovery mechanism under a specific data partition scheme of workload balance. We believe the outcome of this project will help to build a theoretical basis for the future real time system design. It could also be applied to the existing systems to improve the system performance, in terms of the throughput and processing latency, against dynamic workloads. Overall, the project is of great value both theoretically and practically.
在众多领域,数据具有时效性,即数据的价值更多聚集于其"新鲜"状态下。近年来,对用于海量数据实时处理的分布式数据流系统研究已经成为数据科学与大数据技术发展不可或缺的部分。然而,数据流的持续、动态及不可预知等固有特性给数据流系统的高性能、高可用机制设计带来了极大挑战。在此背景下,本项目以如何使负载均衡与容错机制“互利共赢”地共存于系统为导向,针对当前数据流处理系统中轻量级自适应负载均衡算法匮乏、弹性处理架构代价大及容错成本高等问题,拟设计一套同时实现处理节点高性能和系统高可用的均衡容错融合方案。研究内容包括:(1)根据数据及节点负载情况快速、位置敏感的均衡负载分配理论;(2)动态负载下,均衡容错融合机制中轻量级负载部署、调整方案;(3)均衡负载限定数据划分方式下的数据备份/恢复机制。项目的研究成果既为相关实时系统的设计提供理论基础,又可直接应用到现有系统中,具有较高的理论研究和实际应用价值。
结项摘要
随着信息技术的飞速发展,海量数据的及时处理分析需要日趋突出。海量实时数据处理技术能够助力更大程度地发掘数据价值。本项目以研究能够以较小资源开销为代价,保证实时数据流处理系统持续正确地处在高性能处理状态的技术为目标,分别探索了实时数据流处理系统中的并行处理的高性能方案、弹性架构的低代价方法及处理节点的强容错策略。基于此目标,本项目通过(1)负载均衡的理论保证,(2)动态负载的均衡策略;(3)均衡敏感的容错机制三个方面的研究内容展开工作。本项目的研究成果可以被直接应用到基于并行架构的各类实时数据流处理系统上,能够较显著地提升现有系统性能,进而催生更多大数据应用移植到实时处理系统上,让更多行业可以借助实时大数据处理来发掘聚集于数据“新鲜”状态下的价值。
项目成果
期刊论文数量(13)
专著数量(0)
科研奖励数量(0)
会议论文数量(13)
专利数量(0)
On workflow aware location-based service composition for personal trip planning
基于工作流感知的基于位置的个人旅行规划服务组合
- DOI:10.1016/j.future.2019.03.010
- 发表时间:2019-09
- 期刊:Future Generation Computer Systems-The International Journal of eScience
- 影响因子:7.5
- 作者:Xu Jiajie;Chen Jing;Zhou Rui;Fang Junhua;Liu Chengfei
- 通讯作者:Liu Chengfei
MTLM: a multi-task learning model for travel time estimation
MTLM:用于行程时间估计的多任务学习模型
- DOI:10.1007/s10707-020-00422-x
- 发表时间:2022
- 期刊:GeoInformatica (CCF-B类国际期刊)
- 影响因子:--
- 作者:Saijun Xu;Ruoqian Zhang;Wanjun Cheng;Jiajie Xu
- 通讯作者:Jiajie Xu
Scalable and Adaptive Joins for Trajectory Data in Distributed Stream System
分布式流系统中轨迹数据的可扩展和自适应连接
- DOI:10.1007/s11390-019-1940-x
- 发表时间:2019
- 期刊:Journal of Computer Science and Technology
- 影响因子:0.7
- 作者:Fang Jun Hua;Zhao Peng Peng;Liu An;Li Zhi Xu;Zhao Lei
- 通讯作者:Zhao Lei
Destination Prediction A Deep Learning based Approach
目的地预测基于深度学习的方法
- DOI:10.1109/tkde.2019.2932984
- 发表时间:2019
- 期刊:IEEE Transactions on Knowledge and Data Engineering
- 影响因子:8.9
- 作者:Jiajie Xu;Jing Zhao;Rui Zhou;Chengfei Liu;Pengpeng Zhao;Lei Zhao
- 通讯作者:Lei Zhao
TrajVAE: A Variational AutoEncoder model for trajectory generation
TrajVAE:用于轨迹生成的变分自动编码器模型
- DOI:10.1016/j.neucom.2020.03.120
- 发表时间:2021-01-15
- 期刊:NEUROCOMPUTING
- 影响因子:6
- 作者:Chen, Xinyu;Xu, Jiajie;Liu, Chengfei
- 通讯作者:Liu, Chengfei
数据更新时间:{{ journalArticles.updateTime }}
{{
item.title }}
{{ item.translation_title }}
- DOI:{{ item.doi || "--"}}
- 发表时间:{{ item.publish_year || "--" }}
- 期刊:{{ item.journal_name }}
- 影响因子:{{ item.factor || "--"}}
- 作者:{{ item.authors }}
- 通讯作者:{{ item.author }}
数据更新时间:{{ journalArticles.updateTime }}
{{ item.title }}
- 作者:{{ item.authors }}
数据更新时间:{{ monograph.updateTime }}
{{ item.title }}
- 作者:{{ item.authors }}
数据更新时间:{{ sciAawards.updateTime }}
{{ item.title }}
- 作者:{{ item.authors }}
数据更新时间:{{ conferencePapers.updateTime }}
{{ item.title }}
- 作者:{{ item.authors }}
数据更新时间:{{ patent.updateTime }}
其他文献
支持通信数据查询分析的分布式计算系统
- DOI:--
- 发表时间:2014
- 期刊:华东师范大学学报(自然科学版)
- 影响因子:--
- 作者:晁平复;郑芷凌;房俊华;张蓉
- 通讯作者:张蓉
分布式可扩展数据流连接算法
- DOI:--
- 发表时间:2016
- 期刊:华东师范大学学报(自然科学版)
- 影响因子:--
- 作者:王晓桐;房俊华;张蓉
- 通讯作者:张蓉
支持非等值连接的分布式数据流处理系统
- DOI:--
- 发表时间:2017
- 期刊:华东师范大学学报(自然科学版)
- 影响因子:--
- 作者:陈明珠;王晓桐;房俊华;张蓉
- 通讯作者:张蓉
其他文献
{{
item.title }}
{{ item.translation_title }}
- DOI:{{ item.doi || "--" }}
- 发表时间:{{ item.publish_year || "--"}}
- 期刊:{{ item.journal_name }}
- 影响因子:{{ item.factor || "--" }}
- 作者:{{ item.authors }}
- 通讯作者:{{ item.author }}
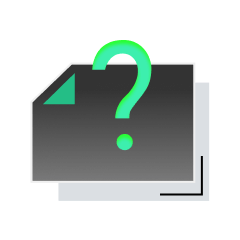
内容获取失败,请点击重试
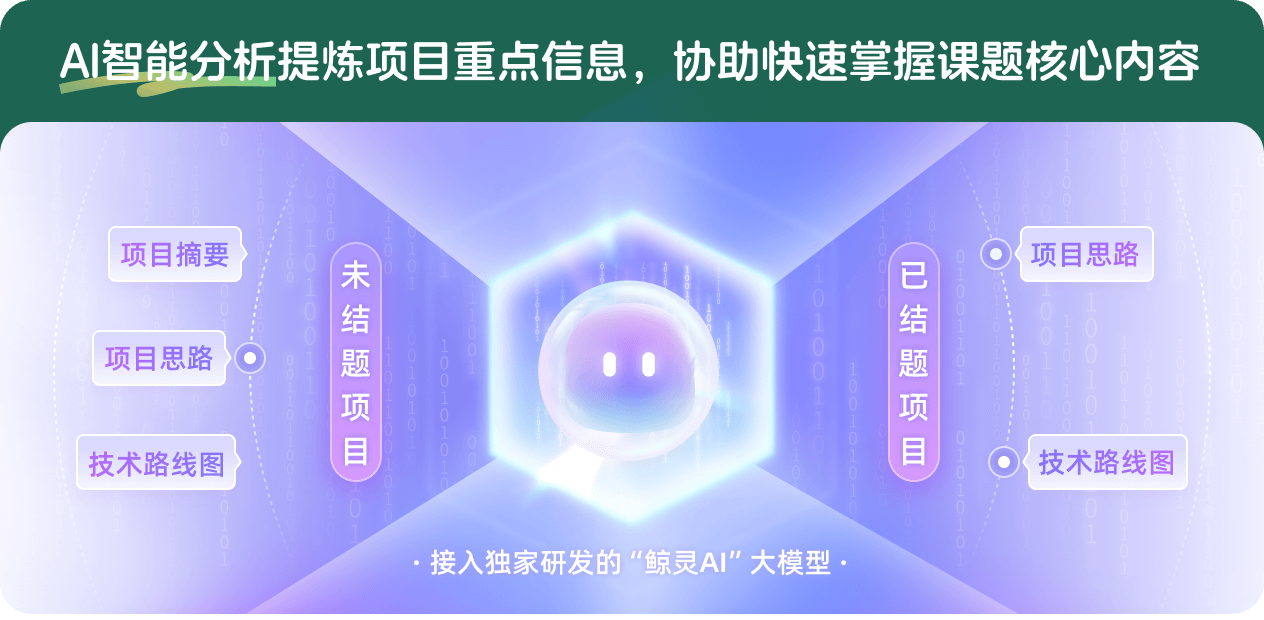
查看分析示例
此项目为已结题,我已根据课题信息分析并撰写以下内容,帮您拓宽课题思路:
AI项目摘要
AI项目思路
AI技术路线图
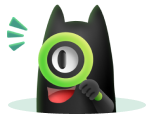
请为本次AI项目解读的内容对您的实用性打分
非常不实用
非常实用
1
2
3
4
5
6
7
8
9
10
您认为此功能如何分析更能满足您的需求,请填写您的反馈:
相似国自然基金
{{ item.name }}
- 批准号:{{ item.ratify_no }}
- 批准年份:{{ item.approval_year }}
- 资助金额:{{ item.support_num }}
- 项目类别:{{ item.project_type }}
相似海外基金
{{
item.name }}
{{ item.translate_name }}
- 批准号:{{ item.ratify_no }}
- 财政年份:{{ item.approval_year }}
- 资助金额:{{ item.support_num }}
- 项目类别:{{ item.project_type }}