面向机器人的Spiking神经网络小储备池计算理论及其应用研究
项目介绍
AI项目解读
基本信息
- 批准号:61473051
- 项目类别:面上项目
- 资助金额:80.0万
- 负责人:
- 依托单位:
- 学科分类:F0306.自动化检测技术与装置
- 结题年份:2018
- 批准年份:2014
- 项目状态:已结题
- 起止时间:2015-01-01 至2018-12-31
- 项目参与者:李秀敏; 李楠; 赖俊峰; 敖伟; 罗小锁; 陈强; 王玮; 向剑; 钟玲;
- 关键词:
项目摘要
The rapid development of robotic applications makes increasing demands of robot system design tools such as artificial neural networks. But as the third generation of neural network and has good prospects in the field of robotics, Spiking Neural Network (SNN) has not been widely used. On this issue, this project aims to carry out research from the modeling study, then the application of typical robot, and finally into the basic research of SNN toolbox, with the application of reservoir computing of SNN as a starting point. By analyzing the advantages and challenges of SNN in the field of robotics, we propose the concept of "small reservoir" which serves as a core, study a series of questions such as, the modeling of SNN with small reservoir, the construction of reservoir topology, and then build computational model with the optimal performance and the smallest size. With the guidance of mechanism research and statistical analysis, the method of optimization of topology and parameter, the index of information entropy and accuracy, we plans to design a method with multi-mechanism integration and multi-objective optimization, to obtain suitable spiking neural networks for specific target task. In addition, with the representation of multi-task robots and autonomous robots in complex environments, we also investigate the application of complex robot, and finally design and implement a tool system of such methods.
机器人应用的迅猛发展对人工神经网络等机器人系统设计工具提出了越来越高的要求,但作为第三代神经网络并在机器人领域具有很好前景的Spiking神经网络(SNN)却没有得到广泛应用。本项目围绕这一问题,以SNN的储备池计算模型为切入点,开展从模型研究到典型机器人应用,再到SNN工具箱的应用基础研究。通过分析SNN在机器人领域的优势与挑战,提出"小储备池"的概念,并以此为核心,研究小储备池SNN的神经元模型构建、储备池拓扑结构构建等一系列问题,进而构建具有最优性能和最小规模的计算模型。以机理研究和统计分析为指导,以拓扑优化、参数优化等为手段,以信息墒、精度等为指标,设计多机制综合多目标优化方法,获得适合目标任务的Spiking神经网络。同时,以多任务机器人和复杂环境中的自主机器人以代表,研究其在复杂机器人上的应用,进而设计并实现出该类方法的工具体系。
结项摘要
机器人应用的迅猛发展对人工神经网络等机器人系统设计工具提出了越来越高的要求,但作为第三代神经网络并在机器人领域具有很好前景的Spiking神经网络(SNN)却没有得到广泛应用。基于当前存在的问题,本项目以SNN的储备池计算模型为切入点,开展从模型研究到典型机器人应用,再到SNN工具箱的应用基础研究。具体从小储备池神经网络的神经元模型、储备池网络拓扑、神经网络构建与应用,以及软件设计工具等四大方面进行研究。首先在神经元方面,研究了HH、IF、IZH等经典的脉冲神经网络(SNN)神经元模型,以及IZH+Delay模型、Bursting模型及概念机(Conceptor)模型;其次在在储备池拓扑结构方面,我们研究了小世界多簇、环状结构,以及主动占优结构等多类型神经元混合结构对网络计算新能的影响。并将网络运用到了时间序列预测、图像分类、移动机器人障碍物检测上;最后设计制作了脉冲神经网络仿真实验平台系统,为将Spiking神经网络小储备池计算理论应用到机器人控制决策系统中提供了硬件实现平台。
项目成果
期刊论文数量(7)
专著数量(0)
科研奖励数量(0)
会议论文数量(5)
专利数量(6)
A priori data-driven multi-clustered reservoir generation algorithm for echo state network.
一种先验数据驱动的 Echo 状态网络多集群储层生成算法
- DOI:10.1371/journal.pone.0120750
- 发表时间:2015
- 期刊:PloS one
- 影响因子:3.7
- 作者:Li X;Zhong L;Xue F;Zhang A
- 通讯作者:Zhang A
Computational modeling of spiking neural network with learning rules from STDP and intrinsic plasticity
具有 STDP 学习规则和内在可塑性的尖峰神经网络的计算模型
- DOI:10.1016/j.physa.2017.08.053
- 发表时间:2018-02-01
- 期刊:PHYSICA A-STATISTICAL MECHANICS AND ITS APPLICATIONS
- 影响因子:3.3
- 作者:Li, Xiumin;Wang, Wei;Song, Yongduan
- 通讯作者:Song, Yongduan
Reservoir Computing with Both Neuronal Intrinsic Plasticity and Multi-Clustered Structure
兼具神经元固有可塑性和多簇结构的储层计算
- DOI:10.1007/s12559-017-9467-3
- 发表时间:2017-05
- 期刊:Cognitive Computation
- 影响因子:5.4
- 作者:Xue Fangzheng;Li Qian;Zhou Hongjun;Li Xiumin
- 通讯作者:Li Xiumin
Liquid computing of spiking neural network with multi-clustered and active-neuron-dominant structure
多簇主动神经元主导结构的尖峰神经网络的液体计算
- DOI:10.1016/j.neucom.2017.03.022
- 发表时间:2017-06-21
- 期刊:NEUROCOMPUTING
- 影响因子:6
- 作者:Li, Xiumin;Liu, Hui;Song, Yongduan
- 通讯作者:Song, Yongduan
The combination of circle topology and leaky integrator neurons remarkably improves the performance of echo state network on time series prediction.
圆形拓扑和漏积分神经元的结合显着提高了回声状态网络的时间序列预测性能
- DOI:10.1371/journal.pone.0181816
- 发表时间:2017
- 期刊:PloS one
- 影响因子:3.7
- 作者:Xue F;Li Q;Li X
- 通讯作者:Li X
数据更新时间:{{ journalArticles.updateTime }}
{{
item.title }}
{{ item.translation_title }}
- DOI:{{ item.doi || "--"}}
- 发表时间:{{ item.publish_year || "--" }}
- 期刊:{{ item.journal_name }}
- 影响因子:{{ item.factor || "--"}}
- 作者:{{ item.authors }}
- 通讯作者:{{ item.author }}
数据更新时间:{{ journalArticles.updateTime }}
{{ item.title }}
- 作者:{{ item.authors }}
数据更新时间:{{ monograph.updateTime }}
{{ item.title }}
- 作者:{{ item.authors }}
数据更新时间:{{ sciAawards.updateTime }}
{{ item.title }}
- 作者:{{ item.authors }}
数据更新时间:{{ conferencePapers.updateTime }}
{{ item.title }}
- 作者:{{ item.authors }}
数据更新时间:{{ patent.updateTime }}
其他文献
基于仿生机器鱼的人工生命模型
- DOI:--
- 发表时间:--
- 期刊:重庆理工大学学报:自然科学
- 影响因子:--
- 作者:金旭东;薛方正
- 通讯作者:薛方正
CPLD六倍频在无刷直流电机速度采集中的应用
- DOI:--
- 发表时间:--
- 期刊:传感器与微系统
- 影响因子:--
- 作者:钟灶生;薛方正;田小玲;敬成林;ZHONG Zao-sheng;XUE Fang-zheng;TIAN Xiao-ling;JING Cheng-lin
- 通讯作者:JING Cheng-lin
Implementation method of predictive control for biped robot stabilization walking pattern
双足机器人稳定行走模式预测控制的实现方法
- DOI:--
- 发表时间:--
- 期刊:Yi Qi Yi Biao Xue Bao/chinese Journal of Scientific Instrument
- 影响因子:--
- 作者:敬成林;薛方正;张华;李祖枢;Jing Chenglin;Xue Fangzheng;Zhang Hua;Li Zushu
- 通讯作者:Li Zushu
双足机器人预观控制的ZMP补偿步行模式研究
- DOI:--
- 发表时间:--
- 期刊:计算机工程与应用
- 影响因子:--
- 作者:敬成林;李祖枢;薛方正;JING Chenglin;LI Zushu;XUE Fangzheng
- 通讯作者:XUE Fangzheng
分布式电源在仿人机器人控制系统中的应用
- DOI:--
- 发表时间:--
- 期刊:传感器与微系统
- 影响因子:--
- 作者:钟灶生;薛方正;敬成林;ZHONG Zao-sheng;XUE Fang-zheng;JING Cheng-lin
- 通讯作者:JING Cheng-lin
其他文献
{{
item.title }}
{{ item.translation_title }}
- DOI:{{ item.doi || "--" }}
- 发表时间:{{ item.publish_year || "--"}}
- 期刊:{{ item.journal_name }}
- 影响因子:{{ item.factor || "--" }}
- 作者:{{ item.authors }}
- 通讯作者:{{ item.author }}
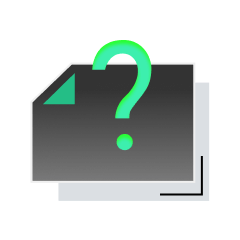
内容获取失败,请点击重试
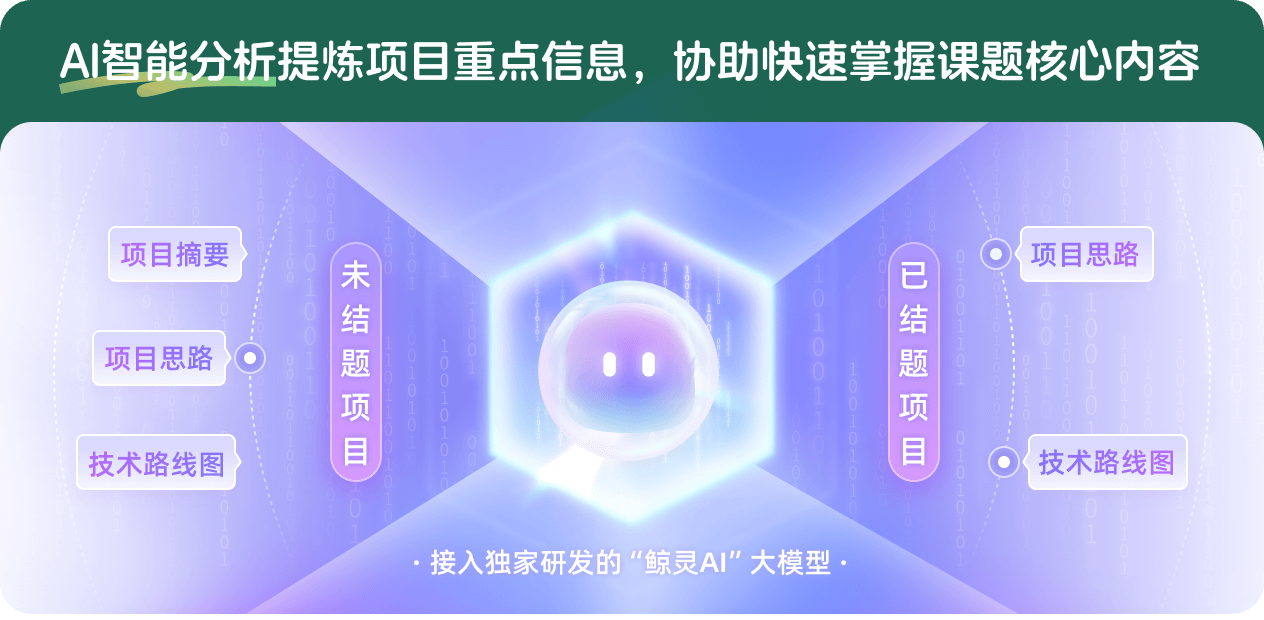
查看分析示例
此项目为已结题,我已根据课题信息分析并撰写以下内容,帮您拓宽课题思路:
AI项目摘要
AI项目思路
AI技术路线图
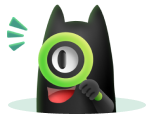
请为本次AI项目解读的内容对您的实用性打分
非常不实用
非常实用
1
2
3
4
5
6
7
8
9
10
您认为此功能如何分析更能满足您的需求,请填写您的反馈:
薛方正的其他基金
仿人机器人大范围动态快速步行关键技术研究
- 批准号:60905053
- 批准年份:2009
- 资助金额:17.0 万元
- 项目类别:青年科学基金项目
相似国自然基金
{{ item.name }}
- 批准号:{{ item.ratify_no }}
- 批准年份:{{ item.approval_year }}
- 资助金额:{{ item.support_num }}
- 项目类别:{{ item.project_type }}
相似海外基金
{{
item.name }}
{{ item.translate_name }}
- 批准号:{{ item.ratify_no }}
- 财政年份:{{ item.approval_year }}
- 资助金额:{{ item.support_num }}
- 项目类别:{{ item.project_type }}