网络化多自主体系统的分布式优化及其在传感器网络中的应用
项目介绍
AI项目解读
基本信息
- 批准号:61304038
- 项目类别:青年科学基金项目
- 资助金额:25.0万
- 负责人:
- 依托单位:
- 学科分类:F0301.控制理论与技术
- 结题年份:2016
- 批准年份:2013
- 项目状态:已结题
- 起止时间:2014-01-01 至2016-12-31
- 项目参与者:黄双喜; 苏薇; 张玉利; 丁见亚; 李柳熙; 林金表; 李爽; 秦宸;
- 关键词:
项目摘要
This project mainly studies the distributed optimization over networked multi-agent systems and its applications in sensor networks.The goal of distributed optimization is to ensure that each agent achieves a global objective by using local communication and information processing, and the collaboration among agents. The interaction between agents is described by a network toplogy. It arises in various application domains including sensor networks,distributed computation, multi-agent coordination, large-scale machine learning, intelligent transportation and etc. For a global objective formed by a sum of agents' cost functions, a distributed optimization algorithm is proposed by integrating ideas of the Newton and consensus algorithms, whose performance is to be evaluated as well. The striking feature of the algorithm lies in the ease of implementation in a distributed manner, fast convergence, scalability and etc. The joint effect of channel uncertainties and the variation of the network topology is to be quantified. Then, the algorithm is tailored to solve several important application issues in sensor networks such as distributed localization and tracking, self network configuration, distributed task assignment and etc.
本项目重点研究网络化多自主体系统的分布式优化问题及其在传感器网络中的应用。分布式优化仅利用局部的通信与信息处理技术和自主体之间的协作使得各个自主体均实现全局优化目标。自主体之间的相互作用通过一网络拓扑结构来描述。其广泛应用于传感器网络、分布式计算、多自主体协调、大规模机器学习、智能交通等领域。针对全局目标为单个自主体的成本函数的和函数,构造基于牛顿优化算法和趋同算法的分布式优化算法,并分析算法的性能。该算法具有易分布式实现、收敛速度快、扩展性强等特点。分别刻画具有不确定性的网络信道和时变拓扑结构对算法性能的影响。基于提出的分布式优化算法,解决传感器网络中诸如分布式目标定位与跟踪、自组网、最优任务分配等重点应用问题。
结项摘要
现有的优化过程往往利用中心处理器来实现对的历史采样数据建模。本项目拟基于现实的大数据应用场景,为提高决策的有效性,采用分布式优化方法进行大数据优化决策的研究。首次通过引进松弛变量,将原始分布式优化问题转化为优化问题的上镜图形式(epigraph form)。基于此,有效地克服了现有分布式优化算法只适用于平衡图的局限,并很好地应用于时变拓扑图的情形。此外,研究成果应用于大规模网络的中心度测量的分布式计算问题、移动传感器网络节点协同定位问题,并讨论了多种不确定网络环境对优化的影响,大部分研究成果发表在控制系统两个权威期刊 Automatica 和 IEEE Trans. on Automatic Control (IEEE TAC) 上。依托本项目,发表 IEEE TAC 和Automatica 上发表 5 篇论文,长文 2 篇,在投 IEEE TAC 论文 2 篇。在 Springer 出版社出版一作专著一部。
项目成果
期刊论文数量(8)
专著数量(1)
科研奖励数量(0)
会议论文数量(6)
专利数量(0)
Quantized identification of ARMA systems with colored measurement noise
具有彩色测量噪声的 ARMA 系统的量化识别
- DOI:10.1016/j.automatica.2015.12.013
- 发表时间:2016-04
- 期刊:Automatica
- 影响因子:6.4
- 作者:Chengpu Yu;Keyou You;Lihua Xie
- 通讯作者:Lihua Xie
Data rate theorems for stabilization of networked linear systems
用于稳定网络线性系统的数据速率定理
- DOI:--
- 发表时间:2014
- 期刊:Control Theory & Applications
- 影响因子:--
- 作者:You, Ke-You;Xie, Li-Hua
- 通讯作者:Xie, Li-Hua
Stability conditions for multi-sensor state estimation over a lossy network
有损网络上多传感器状态估计的稳定性条件
- DOI:10.1016/j.automatica.2014.12.022
- 发表时间:2015-03
- 期刊:Automatica
- 影响因子:6.4
- 作者:Sui, Tianju;You, Keyou;Fu, Minyue
- 通讯作者:Fu, Minyue
Distributed Algorithms for Computation of Centrality Measures in Complex Networks
复杂网络中中心性度量计算的分布式算法
- DOI:10.1109/tac.2016.2604373
- 发表时间:2017-05-01
- 期刊:IEEE TRANSACTIONS ON AUTOMATIC CONTROL
- 影响因子:6.8
- 作者:You, Keyou;Tempo, Roberto;Qiu, Li
- 通讯作者:Qiu, Li
Recursive algorithms for parameter estimation with adaptive quantizer
使用自适应量化器进行参数估计的递归算法
- DOI:10.1016/j.automatica.2014.11.018
- 发表时间:2015-02
- 期刊:Automatica
- 影响因子:6.4
- 作者:You, Keyou
- 通讯作者:You, Keyou
数据更新时间:{{ journalArticles.updateTime }}
{{
item.title }}
{{ item.translation_title }}
- DOI:{{ item.doi || "--"}}
- 发表时间:{{ item.publish_year || "--" }}
- 期刊:{{ item.journal_name }}
- 影响因子:{{ item.factor || "--"}}
- 作者:{{ item.authors }}
- 通讯作者:{{ item.author }}
数据更新时间:{{ journalArticles.updateTime }}
{{ item.title }}
- 作者:{{ item.authors }}
数据更新时间:{{ monograph.updateTime }}
{{ item.title }}
- 作者:{{ item.authors }}
数据更新时间:{{ sciAawards.updateTime }}
{{ item.title }}
- 作者:{{ item.authors }}
数据更新时间:{{ conferencePapers.updateTime }}
{{ item.title }}
- 作者:{{ item.authors }}
数据更新时间:{{ patent.updateTime }}
其他文献
Trends in extreme learning machine: a review
极限学习机的趋势:回顾
- DOI:--
- 发表时间:2015
- 期刊:Neural Networks
- 影响因子:7.8
- 作者:黄高;宋士吉;黄广斌;游科友
- 通讯作者:游科友
其他文献
{{
item.title }}
{{ item.translation_title }}
- DOI:{{ item.doi || "--" }}
- 发表时间:{{ item.publish_year || "--"}}
- 期刊:{{ item.journal_name }}
- 影响因子:{{ item.factor || "--" }}
- 作者:{{ item.authors }}
- 通讯作者:{{ item.author }}
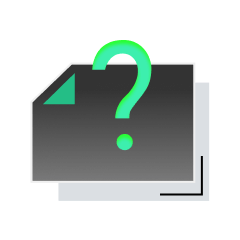
内容获取失败,请点击重试
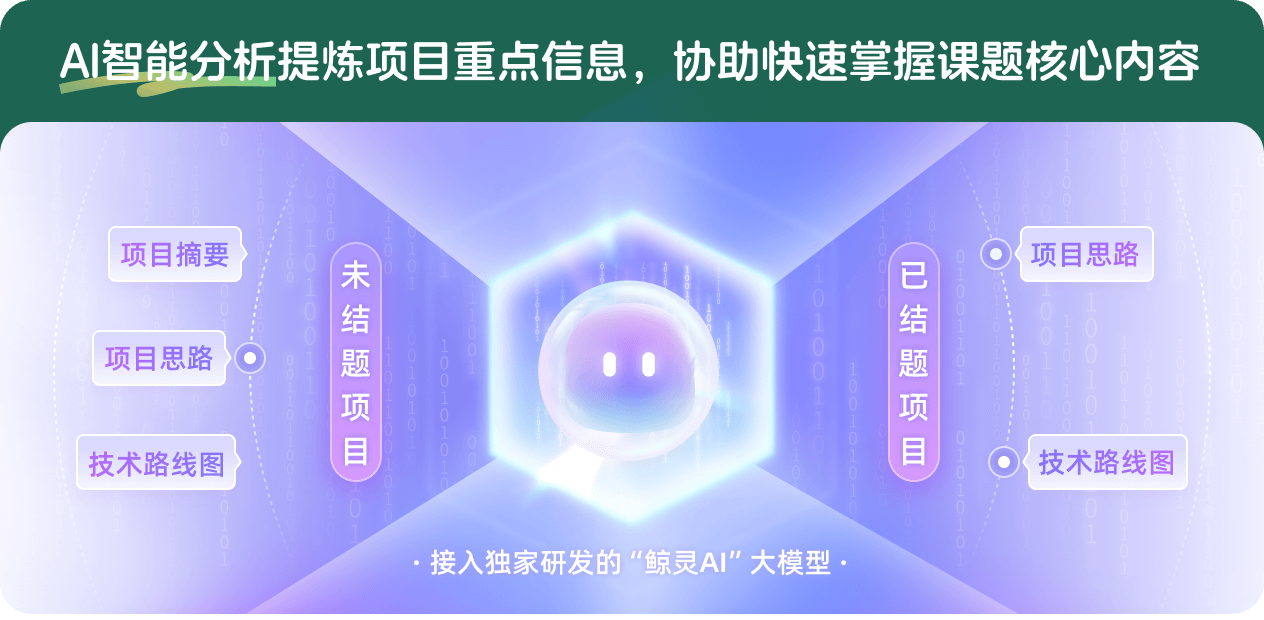
查看分析示例
此项目为已结题,我已根据课题信息分析并撰写以下内容,帮您拓宽课题思路:
AI项目摘要
AI项目思路
AI技术路线图
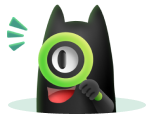
请为本次AI项目解读的内容对您的实用性打分
非常不实用
非常实用
1
2
3
4
5
6
7
8
9
10
您认为此功能如何分析更能满足您的需求,请填写您的反馈:
游科友的其他基金
面向重载列车虚拟耦合运行的协同控制理论与方法
- 批准号:62033006
- 批准年份:2020
- 资助金额:292 万元
- 项目类别:重点项目
缆控采样器的水动力学模型及其运动控制
- 批准号:41576101
- 批准年份:2015
- 资助金额:75.0 万元
- 项目类别:面上项目
相似国自然基金
{{ item.name }}
- 批准号:{{ item.ratify_no }}
- 批准年份:{{ item.approval_year }}
- 资助金额:{{ item.support_num }}
- 项目类别:{{ item.project_type }}
相似海外基金
{{
item.name }}
{{ item.translate_name }}
- 批准号:{{ item.ratify_no }}
- 财政年份:{{ item.approval_year }}
- 资助金额:{{ item.support_num }}
- 项目类别:{{ item.project_type }}