数据分布自学习深度神经网络概率模型与应用
项目介绍
AI项目解读
基本信息
- 批准号:61906093
- 项目类别:青年科学基金项目
- 资助金额:27.0万
- 负责人:
- 依托单位:
- 学科分类:F0601.人工智能基础
- 结题年份:2022
- 批准年份:2019
- 项目状态:已结题
- 起止时间:2020-01-01 至2022-12-31
- 项目参与者:--
- 关键词:
项目摘要
Unsupervised representation learning played an important role in the developement of deep neural networks. This project is inspired from the association and memory mechanism of brain and develops data distribution adaptive deep neural network probabilistic model based on unsupervised representation learning modeling theory. This model aims to learn the complex probabilistic distribution of data in order to achieve numerous machine learning tasks. By this modeling method, a probabilistic model for architecture optimization of deep neural networks is established. It models the distribution of data directly based on the network architecture and an objective function without incorporating with network weights is derived. This significantly reduces the computational complexity of objectives in traditional architecture optimization methods. Meanwhile, the distribution adaptive neural network architecture will be learned. Finally, the probabilistic model is applied to a typical problem in which the data are difficult to label, i.e., change detection in multi-temporal remote sensing images. The model is used to solve the difficulty of comparison between heterogeneous multi-source and unregistered remote sensing images. This project comes from practical problems and aims to develop novel deep neural network learning and architecture optimization models. It explores new ideas dealing with machine learning problems and solves typical practical problems based on theoretical researches.
无监督表示学习在深度神经网络的发展中发挥了重要的作用。本课题受人类大脑对感官信息的关联与记忆机制启发,在无监督表示学习建模理论的基础上,发展基于深度神经网络的分布自学习概率模型,学习数据的复杂分布,从而完成各类机器学习任务。然后利用概率建模理论,建立网络结构优化的概率模型,利用网络结构直接对数据分布进行建模,设计不需要网络权重参与的目标函数,解决网络结构优化中目标函数运算复杂度高的问题,同时学习适应数据分布的网络结构。最后我们将概率模型应用到典型的样本标记困难的问题中,即多时遥感影像变化检测,解决多源异质、配准困难场景下难以进行比较的问题。本课题以神经网络的分布学习概率建模为理论基础,发展新的深度神经网络学习与结构优化模型,理论结合实际,解决典型的困难问题,形成具有源头创新的研究成果。
结项摘要
大规模、种类丰富、非结构化的大数据标注困难,给智能化的方法应用带来很大的挑战。本项目提出一种新的深度学习模型,即深度概率模型,通过定义基于深度神经网络的能量函数,直接对训练数据分布进行建模,提出基于优化的采样方法解决求解最大似然过程中的采样难题,该模型对标记数据和无标记数据都能够很好地学习数据分布,因此在分类、数据重构和修复、风格转换等方面都具有较好的性能。基于概率模型,本项目提出团结构网络优化,即自组织网络连接,进一步模拟大脑中的核团网络结构,利用网络连接建立概率模型,通过演化算法进行优化,最终得到能够表示数据分布的网络连接结构,实验发现,该结构对无关变量即背景具有很强的鲁棒性,采用纯色背景的手写体数据进行训练,在有噪声背景的测试数据集依然能够保持较好的性能,这对于传统的神经网络是无法做到的。另外,本项目针对多源异质遥感影像提出基于概率模型的变化检测方法,多源异质遥感影像是来源于不同成像传感器且数据结构差异很大的多时图像,变化检测十分困难,目前方法不多且大部分基于有监督学习,这在实际应用中较难实现,通过设计双边网络结构和基于特征差异的能量函数,建立多时图像分布学习模型,该网络模型对有环境干扰的同源及多源异质图像都能够实现精确检测,性能远超对比方法。最后,对有监督变化检测、目标跟踪、检测、场景分类等应用进行了研究,探索概率模型的应用场景。
项目成果
期刊论文数量(12)
专著数量(0)
科研奖励数量(0)
会议论文数量(6)
专利数量(4)
Joint Variation Learning of Fusion and Difference Features for Change Detection in Remote Sensing Images
遥感图像变化检测融合特征和差分特征的联合变分学习
- DOI:10.1109/tgrs.2022.3226778
- 发表时间:2022
- 期刊:IEEE Transactions on Geoscience and Remote Sensing
- 影响因子:8.2
- 作者:Kaixuan Jiang;Wenhua Zhang;Jia Liu;Fang Liu;Liang Xiao
- 通讯作者:Liang Xiao
Learning Pairwise Potential CRFs in Deep Siamese Network for Change Detection
在深度连体网络中学习成对潜在条件随机场以进行变化检测
- DOI:10.3390/rs14040841
- 发表时间:2022-02
- 期刊:Remote Sensing
- 影响因子:5
- 作者:Dalong Zheng;Zhihui Wei;Zebin Wu;Jia Liu
- 通讯作者:Jia Liu
A Probabilistic Model Based on Bipartite Convolutional Neural Network for Unsupervised Change Detection
基于二分卷积神经网络的无监督变化检测概率模型
- DOI:10.1109/tgrs.2021.3071347
- 发表时间:2022
- 期刊:IEEE Transactions on Geoscience and Remote Sensing
- 影响因子:8.2
- 作者:Liu Jia;Zhang Wenhua;Liu Fang;Xiao Liang
- 通讯作者:Xiao Liang
Biophysical Model: A Promising Method in the Study of the Mechanism of Propofol: A Narrative Review.
生物物理模型:异丙酚作用机制研究的一种有前景的方法:叙述性综述
- DOI:10.1155/2022/8202869
- 发表时间:2022
- 期刊:COMPUTATIONAL INTELLIGENCE AND NEUROSCIENCE
- 影响因子:--
- 作者:Li, Zhen;Liu, Jia;Liang, Huazheng
- 通讯作者:Liang, Huazheng
Sparse Feature Clustering Network for Unsupervised SAR Image Change Detection
用于无监督SAR图像变化检测的稀疏特征聚类网络
- DOI:10.1109/tgrs.2022.3167745
- 发表时间:2022
- 期刊:IEEE Transactions on Geoscience and Remote Sensing
- 影响因子:8.2
- 作者:Wenhua Zhang;Licheng Jiao;Fang Liu;Shuyuan Yang;Wei Song;Jia Liu
- 通讯作者:Jia Liu
数据更新时间:{{ journalArticles.updateTime }}
{{
item.title }}
{{ item.translation_title }}
- DOI:{{ item.doi || "--"}}
- 发表时间:{{ item.publish_year || "--" }}
- 期刊:{{ item.journal_name }}
- 影响因子:{{ item.factor || "--"}}
- 作者:{{ item.authors }}
- 通讯作者:{{ item.author }}
数据更新时间:{{ journalArticles.updateTime }}
{{ item.title }}
- 作者:{{ item.authors }}
数据更新时间:{{ monograph.updateTime }}
{{ item.title }}
- 作者:{{ item.authors }}
数据更新时间:{{ sciAawards.updateTime }}
{{ item.title }}
- 作者:{{ item.authors }}
数据更新时间:{{ conferencePapers.updateTime }}
{{ item.title }}
- 作者:{{ item.authors }}
数据更新时间:{{ patent.updateTime }}
其他文献
美洲大蠊不同提取物清除自由基及抗脂质过氧化活性研究
- DOI:10.13386/j.issn1002-0306.2016.13.008
- 发表时间:2016
- 期刊:食品工业科技
- 影响因子:--
- 作者:唐霞光;张春妹;刘嘉;肖培云;杨永寿
- 通讯作者:杨永寿
无细胞外泌体疗法促进软骨修复的研究进展
- DOI:10.3760/cma.j.issn.1001-9030.2019.08.059
- 发表时间:2019
- 期刊:中华实验外科杂志
- 影响因子:--
- 作者:刘嘉;潘建锋;陈维华
- 通讯作者:陈维华
云南松松针中原花青素的含量测定研究
- DOI:--
- 发表时间:2016
- 期刊:大理大学学报
- 影响因子:--
- 作者:刘嘉;肖培云;刘光明;杨永寿
- 通讯作者:杨永寿
非常规突发事件情景演化机理及集群决策模式研究
- DOI:--
- 发表时间:--
- 期刊:武汉理工大学学报(社会科学版)
- 影响因子:--
- 作者:陈刚;谢科范;刘嘉;吴倩
- 通讯作者:吴倩
有待用风电机组且传输受限电网的源网协调调度方法(英文)
- DOI:--
- 发表时间:2013
- 期刊:电力系统自动化
- 影响因子:--
- 作者:刘嘉;严干贵;郑太一;徐广鑫
- 通讯作者:徐广鑫
其他文献
{{
item.title }}
{{ item.translation_title }}
- DOI:{{ item.doi || "--" }}
- 发表时间:{{ item.publish_year || "--"}}
- 期刊:{{ item.journal_name }}
- 影响因子:{{ item.factor || "--" }}
- 作者:{{ item.authors }}
- 通讯作者:{{ item.author }}
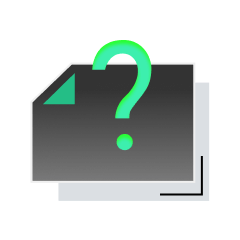
内容获取失败,请点击重试
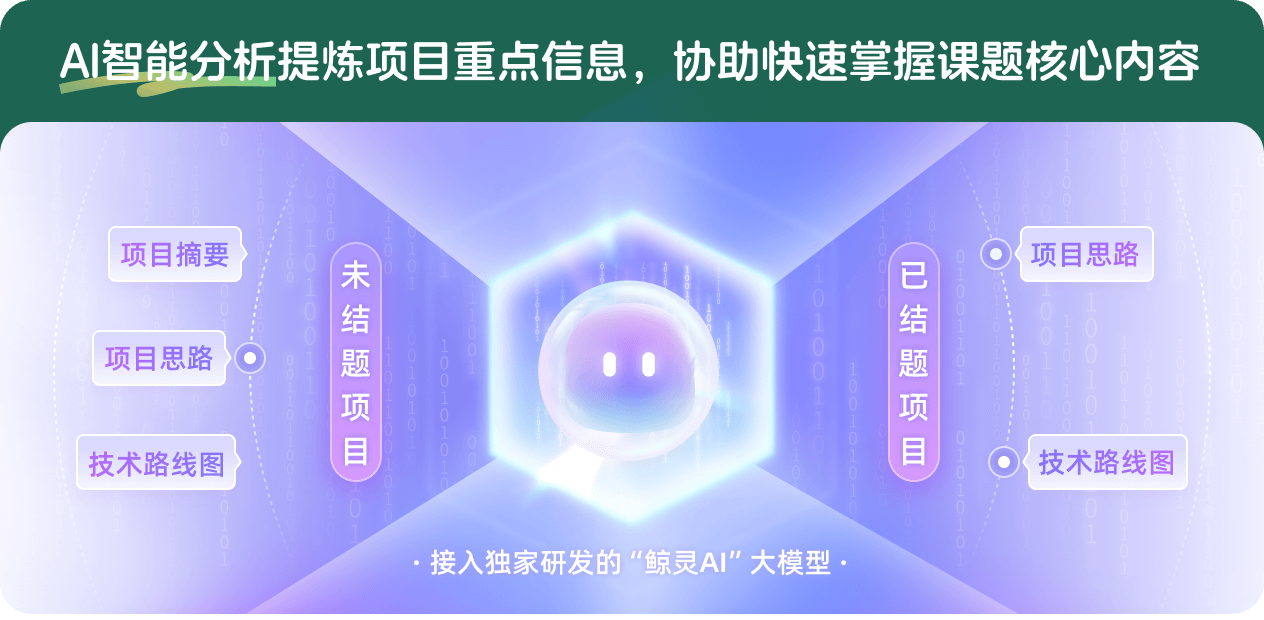
查看分析示例
此项目为已结题,我已根据课题信息分析并撰写以下内容,帮您拓宽课题思路:
AI项目摘要
AI项目思路
AI技术路线图
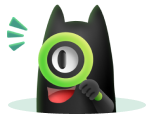
请为本次AI项目解读的内容对您的实用性打分
非常不实用
非常实用
1
2
3
4
5
6
7
8
9
10
您认为此功能如何分析更能满足您的需求,请填写您的反馈:
刘嘉的其他基金
类脑深度关联学习模型与应用
- 批准号:62276133
- 批准年份:2022
- 资助金额:53 万元
- 项目类别:面上项目
相似国自然基金
{{ item.name }}
- 批准号:{{ item.ratify_no }}
- 批准年份:{{ item.approval_year }}
- 资助金额:{{ item.support_num }}
- 项目类别:{{ item.project_type }}
相似海外基金
{{
item.name }}
{{ item.translate_name }}
- 批准号:{{ item.ratify_no }}
- 财政年份:{{ item.approval_year }}
- 资助金额:{{ item.support_num }}
- 项目类别:{{ item.project_type }}