多源数据机器学习中的隐私保护技术研究
项目介绍
AI项目解读
基本信息
- 批准号:61902290
- 项目类别:青年科学基金项目
- 资助金额:30.0万
- 负责人:
- 依托单位:
- 学科分类:F0205.网络与系统安全
- 结题年份:2022
- 批准年份:2019
- 项目状态:已结题
- 起止时间:2020-01-01 至2022-12-31
- 项目参与者:--
- 关键词:
项目摘要
Machine learning has aroused a lot of attention and has been used successfully in many domains. Generally, the training of models requires large, representative datasets, which may be collected from a large number of users and contain sensitive information. And the collected data would be stored and computed by service providers or delegated to an untrusted cloud, which make the privacy issues prominent and severe. .. To solve the privacy issues in multi-source-data machine learning, we first study the problem of adaptive adjustment of privacy policy and propose an adaptive privacy-preserving mechanism to meet the real-time dynamic requirements of users for privacy preservation in complex situations. To preserve the privacy during model training, a privacy-preserving asynchronous machine learning framework with multiple parties is proposed. On the basis of ensuring efficiency, we also design a multi-key privacy-preserving training mechanism and a multi-level privacy-preserving model for diversified multi-key encrypted data. Finally, we study the deterministic fine-grained deletion problem of privacy data. Based on the key-deriving encryption, a kind of key-updating operation for bloke data is designed to achieve the fine-grained deletion. And based on the authentication and signature, a publicly verifiable feedback mechanism for machine learning is also proposed to ensuring the deletion of privacy data.
随着机器学习服务的快速发展,其面临的隐私泄露问题也逐渐受到国家和社会的重视。本项目以机器学习模型训练和应用为中心,从理论方案和系统验证两个角度出发研究多源数据机器学习服务中面临的数据安全和隐私泄露问题:研究任务感知的隐私策略自适应选择问题,设计提出针对不同学习任务和场景的自适应隐私保护机制,从而满足用户在复杂情况下对隐私保护方案的实时动态需求;研究多源数据的机器学习模型安全训练问题,设计面向异步机器学习和多密钥、多样化隐私数据的模型训练和预测机制,在保证计算效率的基础上,解决现有隐私保护方案存在的模型训练准确度低、计算开销大、可用性难以保证等问题;研究隐私数据粒度化删除的确定性反馈机制,设计基于密钥派生加密的块节点密钥更新操作,支持分块或部分数据的粒度化删除操作,提出基于认证签名技术的可公开验证反馈机制,为机器学习服务中隐私数据删除提供可信、可控机制。
结项摘要
机器学习正成为数字经济发展的新引擎,特别是在移动互联网、大数据、超级计算、传感网、脑科学等新理论新技术以及经济社会发展强烈需求的共同驱动下,机器学习将进一步赋能各行各业,推动数字经济深入发展。然而,在大规模数据中进行机器学习所面临的安全问题也正受到越来越广泛的关注。本项目从机器学习服务中数据采集认证、隐私数据安全模型训练、数据安全管理三个方面展开研究,具体如下:. 在数据采集匿名认证方面,针对三因子认证协议中存在的攻击,提出了涵盖多种新型攻击的三因子认证协议安全模型,提出了能够真正实现数据采集匿名认证的协议设计新方法,并设计了三因子匿名认证协议。. 在隐私数据模型安全训练方面,提出了基于轻量级安全计算协议、差分隐私、同态加密等隐私计算技术的模型训练方法与细粒度模型发布机制,实现了机器学习模型训练过程中数据隐私保护,并确保模型训练后实现公平发布利用。同时,设计抗成员推理攻击方案,在模型发布时,个性化的添加差分隐私噪声,从而实现模型发布后的抗推理攻击。. 在数据安全管理方面,提出了基于穿刺加密、属性加密的数据权限撤销方案,从而实现数据在利用完毕后可以高效的细粒度权限撤销,确保用户数据安全利用。考虑到模型训练过程中面临的后门攻击、拜占庭攻击等问题,设计模型修复方案,实现模型训练后的优化与修复,确保训练模型的准确性。. 通过本项目的研究,项目组初步构建了机器学习数据安全利用框架,提出了一套隐私数据安全利用框架,为机器学习服务的大规模安全应用提供支撑。
项目成果
期刊论文数量(17)
专著数量(0)
科研奖励数量(3)
会议论文数量(2)
专利数量(2)
Secure and Usable Handshake Based Pairing for Wrist-Worn Smart Devices on Different Users
为不同用户的腕戴式智能设备提供安全且可用的基于握手的配对
- DOI:10.1007/s11036-021-01781-x
- 发表时间:2021-05
- 期刊:Mobile Networks and Applications volume
- 影响因子:--
- 作者:Guichuan Zhao;Qi Jiang;Xiaohan Huang;Xindi Ma;Youliang Tian;Jianfeng Ma
- 通讯作者:Jianfeng Ma
An efficient three-factor remote user authentication protocol based on BPV-FourQ for internet of drones
基于BPV-FourQ的高效无人机互联网三因素远程用户认证协议
- DOI:10.1007/s12083-021-01130-5
- 发表时间:2021-04-19
- 期刊:PEER-TO-PEER NETWORKING AND APPLICATIONS
- 影响因子:4.2
- 作者:Zhang, Naijian;Jiang, Qi;Ma, Jianfeng
- 通讯作者:Ma, Jianfeng
TrustAccess: A Trustworthy Secure Ciphertext-Policy and Attribute Hiding Access Control Scheme Based on Blockchain
TrustAccess:一种基于区块链的可信安全密文策略和属性隐藏访问控制方案
- DOI:10.1109/tvt.2020.2967099
- 发表时间:2020-06-01
- 期刊:IEEE TRANSACTIONS ON VEHICULAR TECHNOLOGY
- 影响因子:6.8
- 作者:Gao, Sheng;Piao, Guirong;Ma, Jianfeng
- 通讯作者:Ma, Jianfeng
Privacy‐preserving generative framework for images against membership inference attacks
隐私 - 保护图像生成框架免受成员推理攻击
- DOI:10.1049/cmu2.12507
- 发表时间:--
- 期刊:IET Communications
- 影响因子:1.6
- 作者:Ruikang Yang;Jianfeng Ma;Yinbin Miao;Xindi Ma
- 通讯作者:Xindi Ma
Unified Biometric Privacy Preserving Three-Factor Authentication and Key Agreement for Cloud-Assisted Autonomous Vehicles
云辅助自动驾驶汽车的统一生物识别隐私保护三因素身份验证和密钥协议
- DOI:10.1109/tvt.2020.2971254
- 发表时间:2020-09-01
- 期刊:IEEE TRANSACTIONS ON VEHICULAR TECHNOLOGY
- 影响因子:6.8
- 作者:Jiang, Qi;Zhang, Ning;Choo, Kim-Kwang Raymond
- 通讯作者:Choo, Kim-Kwang Raymond
数据更新时间:{{ journalArticles.updateTime }}
{{
item.title }}
{{ item.translation_title }}
- DOI:{{ item.doi || "--"}}
- 发表时间:{{ item.publish_year || "--" }}
- 期刊:{{ item.journal_name }}
- 影响因子:{{ item.factor || "--"}}
- 作者:{{ item.authors }}
- 通讯作者:{{ item.author }}
数据更新时间:{{ journalArticles.updateTime }}
{{ item.title }}
- 作者:{{ item.authors }}
数据更新时间:{{ monograph.updateTime }}
{{ item.title }}
- 作者:{{ item.authors }}
数据更新时间:{{ sciAawards.updateTime }}
{{ item.title }}
- 作者:{{ item.authors }}
数据更新时间:{{ conferencePapers.updateTime }}
{{ item.title }}
- 作者:{{ item.authors }}
数据更新时间:{{ patent.updateTime }}
其他文献
轻量级位置感知推荐系统隐私保护框架
- DOI:--
- 发表时间:2017
- 期刊:计算机学报
- 影响因子:--
- 作者:马鑫迪;李辉;马建峰;习宁;姜奇;高胜;卢笛
- 通讯作者:卢笛
室内定位系统中指纹库的优化方法
- DOI:--
- 发表时间:2015
- 期刊:西安电子科技大学学报
- 影响因子:--
- 作者:马鑫迪;马建峰;高胜
- 通讯作者:高胜
其他文献
{{
item.title }}
{{ item.translation_title }}
- DOI:{{ item.doi || "--" }}
- 发表时间:{{ item.publish_year || "--"}}
- 期刊:{{ item.journal_name }}
- 影响因子:{{ item.factor || "--" }}
- 作者:{{ item.authors }}
- 通讯作者:{{ item.author }}
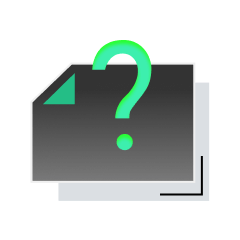
内容获取失败,请点击重试
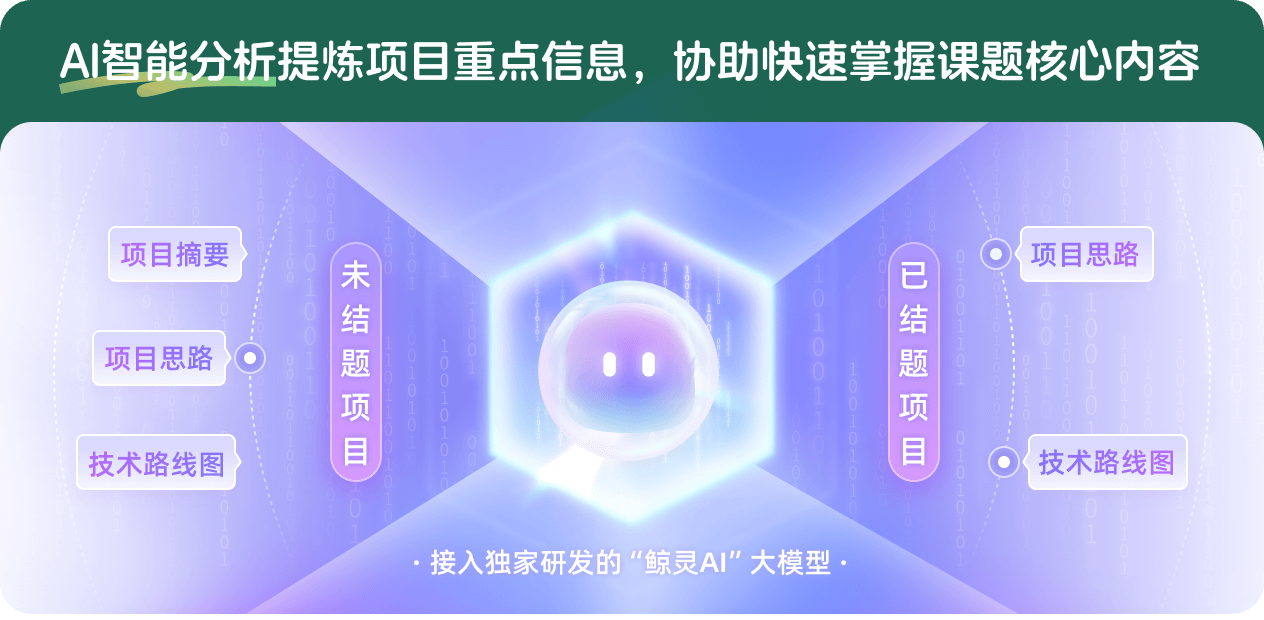
查看分析示例
此项目为已结题,我已根据课题信息分析并撰写以下内容,帮您拓宽课题思路:
AI项目摘要
AI项目思路
AI技术路线图
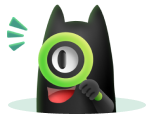
请为本次AI项目解读的内容对您的实用性打分
非常不实用
非常实用
1
2
3
4
5
6
7
8
9
10
您认为此功能如何分析更能满足您的需求,请填写您的反馈:
马鑫迪的其他基金
异构异质密态数据计算的联邦模型安全训练推理研究
- 批准号:62372350
- 批准年份:2023
- 资助金额:50 万元
- 项目类别:面上项目
相似国自然基金
{{ item.name }}
- 批准号:{{ item.ratify_no }}
- 批准年份:{{ item.approval_year }}
- 资助金额:{{ item.support_num }}
- 项目类别:{{ item.project_type }}
相似海外基金
{{
item.name }}
{{ item.translate_name }}
- 批准号:{{ item.ratify_no }}
- 财政年份:{{ item.approval_year }}
- 资助金额:{{ item.support_num }}
- 项目类别:{{ item.project_type }}