大规模开放课程数据中因果关系挖掘方法研究
项目介绍
AI项目解读
基本信息
- 批准号:61877050
- 项目类别:面上项目
- 资助金额:45.0万
- 负责人:
- 依托单位:
- 学科分类:F0701.教育信息科学与技术
- 结题年份:2022
- 批准年份:2018
- 项目状态:已结题
- 起止时间:2019-01-01 至2022-12-31
- 项目参与者:袁新瑞; 陈晓璇; 何绯娟; 宋凌云; 陈静; 刘文强; 吴楠楠; 亢文英; 马龙;
- 关键词:
项目摘要
The focus of MOOC data mining is the correlation between learning effects and learning behaviors. In contrast to causality, the conclusion reached by the correlation analysis of MOOC data is that it is difficult to provide decision support and intervention in a given context. Therefore, the proposal intends to adopt some novel technology to carry out causality extraction and empirical testing methods from MOOC data. The research contents include: .1..Three key problems of causality mining from MOOC data, which have high-dimensional, massive and sparse features, will be solved such as causal diagram construction, consistency identification and hidden variable discovery; .2..Tools and software of causality mining and analysis will be developed. Then verify the effective of our proposed approach in this proposal on MOOC platform...This study extends the research on correlation analysis of educational data to causality mining and benefit to promote the development of educational data mining. The research results of the proposal help to reveal the valuable rules and patterns of learning in large-scale online learning...Expected results include: publishing more than 15 high-level academic papers; applying for 3 to 5 national invention patents; and developing software of causality mining for MOOC data.
当前,MOOC数据挖掘关注的重点是学习效果与学习行为间的相关性。与因果关系相比,相关性分析得到的结论很难提供决策支持和干预手段。为此,本项目拟围绕MOOC数据,对因果关系挖掘开展理论方法研究与实证测试。内容包括:一、针对MOOC数据高维、海量、稀疏特点,重点解决因果图构建、因果图一致性检验以及隐变量发现三项关键问题;二、研制面向MOOC数据的因果关系挖掘与分析工具软件,依托Coursera等MOOC平台数据,对所提方法进行测试、验证与完善。本项目将教育数据相关性分析的研究延伸到因果关系挖掘,有助于促进教育数据挖掘领域的发展,揭示大规模在线学习中有价值的学习规律和模式,为在MOOC平台上构建智能导学提供理论与关键技术支撑。. 预期成果:发表高水平学术论文15篇以上;申请国家发明专利3-5项;研制面向MOOC数据的因果关系挖掘与分析软件。
结项摘要
学习者在MOOC学习过程中产生了大量行为数据,同时MOOC平台也提供了大量学习资源数据以及学习者基本属性数据。对这些海量MOOC数据进行统计分析,将有助于揭示人类更深层次的认知机理,挖掘有价值的学习规律与模式。本项目重点围绕MOOC数据,对因果关系挖掘开展理论方法研究与实证测试。内容包括:一、针对MOOC数据高维、海量、稀疏特点,重点解决MOOC数据辍学行为因果图构建方法研究;二、研制面向MOOC数据的辍学行为因果关系挖掘与分析工具软件。提出了MOOC数据中辍学行为因果关系挖掘研究框架。提出两阶段的因果图构建方法,最后将本研究提出MOOC日志的因果关系挖掘方法整合到智慧教育示范应用系统中,验证了模型的有效性。通过分析自动构建的MOOC数据因果图以及专家的指导,我们找到了两个成因的学习行为:1)课程论坛寻求帮助行为;2)自主做课后习题行为。围绕上述研究成果,我们在主流期刊会议上发表20余篇论文,申请国家发明专利3项,软著2项,培养研究生10人。研究成果还将对智能导学、个性化推荐和学习评价提供辅助决策的依据。
项目成果
期刊论文数量(15)
专著数量(0)
科研奖励数量(0)
会议论文数量(4)
专利数量(3)
Co-Training Semi-Supervised Deep Learning for Sentiment Classification of MOOC Forum Posts
用于 MOOC 论坛帖子情感分类的协同训练半监督深度学习
- DOI:10.3390/sym12010008
- 发表时间:2020-01-01
- 期刊:SYMMETRY-BASEL
- 影响因子:2.7
- 作者:Chen, Jing;Feng, Jun;Liu, Yang
- 通讯作者:Liu, Yang
A parallel neural network structure for sentiment classification of MOOCs discussion forums
MOOCs 论坛情感分类的并行神经网络结构
- DOI:10.3233/jifs-191572
- 发表时间:2020-01-01
- 期刊:JOURNAL OF INTELLIGENT & FUZZY SYSTEMS
- 影响因子:2
- 作者:Gao, Yi;Sun, Xia;Feng, Jun
- 通讯作者:Feng, Jun
Convolutional Recurrent Neural Networks with a Self-Attention Mechanism for Personnel Performance Prediction
具有自注意力机制的卷积循环神经网络用于人员绩效预测
- DOI:10.3390/e21121227
- 发表时间:2019-12-16
- 期刊:Entropy
- 影响因子:2.7
- 作者:Xue X;Feng J;Gao Y;Liu M;Zhang W;Sun X;Zhao A;Guo S
- 通讯作者:Guo S
Word Representation Learning Based on Bidirectional GRUs With Drop Loss for Sentiment Classification
基于带有 Drop Loss 的双向 GRU 的词表示学习用于情感分类
- DOI:10.1109/tsmc.2019.2940097
- 发表时间:2021-07
- 期刊:IEEE Transactions on Systems, Man and Cybernetics: Systems
- 影响因子:--
- 作者:Xia Sun;Yi Gao;Richard Sutcliffe;Shou-Xi Guo;Xin Wang;Jun Feng
- 通讯作者:Jun Feng
Interpretable visual reasoning: A survey
可解释的视觉推理:一项调查
- DOI:10.1016/j.imavis.2021.104194
- 发表时间:2021-06
- 期刊:Image and Vision Computing
- 影响因子:4.7
- 作者:Feijuan He;Yaxian Wang;Xianglin Miao;Xia Sun
- 通讯作者:Xia Sun
数据更新时间:{{ journalArticles.updateTime }}
{{
item.title }}
{{ item.translation_title }}
- DOI:{{ item.doi || "--"}}
- 发表时间:{{ item.publish_year || "--" }}
- 期刊:{{ item.journal_name }}
- 影响因子:{{ item.factor || "--"}}
- 作者:{{ item.authors }}
- 通讯作者:{{ item.author }}
数据更新时间:{{ journalArticles.updateTime }}
{{ item.title }}
- 作者:{{ item.authors }}
数据更新时间:{{ monograph.updateTime }}
{{ item.title }}
- 作者:{{ item.authors }}
数据更新时间:{{ sciAawards.updateTime }}
{{ item.title }}
- 作者:{{ item.authors }}
数据更新时间:{{ conferencePapers.updateTime }}
{{ item.title }}
- 作者:{{ item.authors }}
数据更新时间:{{ patent.updateTime }}
其他文献
耀斑爆发期间电流的突然增加
- DOI:10.14005/j.cnki.issn1672-7673.20211213.001
- 发表时间:2022
- 期刊:天文研究与技术
- 影响因子:--
- 作者:王楠;梁红飞;杨丽平;孙霞;李子涵
- 通讯作者:李子涵
多胺氧化酶活性及木质素沉积与菊花花芽分化的关系
- DOI:--
- 发表时间:2014
- 期刊:华北农学报
- 影响因子:--
- 作者:徐东花;郭俊娥;徐璐;孙霞;孙宪芝
- 通讯作者:孙宪芝
基于GPS技术的小学生放学路径调查与学区服务半径研究
- DOI:--
- 发表时间:--
- 期刊:南方建筑
- 影响因子:--
- 作者:孙霞;李早;李瑾;叶茂盛
- 通讯作者:叶茂盛
土壤铅污染对糜子生长和光合生理特征的影响
- DOI:--
- 发表时间:2020
- 期刊:新疆农业大学学报
- 影响因子:--
- 作者:胡洋;丛孟菲;张梦瑶;冯平;吾买尔夏提·塔汉;侯天钰;孙霞;贾宏涛
- 通讯作者:贾宏涛
亚精胺参与菊花花芽分化的调控研究
- DOI:--
- 发表时间:2014
- 期刊:北京林业大学学报
- 影响因子:--
- 作者:郭俊娥;李田;孙宪芝;孙霞;郑成淑
- 通讯作者:郑成淑
其他文献
{{
item.title }}
{{ item.translation_title }}
- DOI:{{ item.doi || "--" }}
- 发表时间:{{ item.publish_year || "--"}}
- 期刊:{{ item.journal_name }}
- 影响因子:{{ item.factor || "--" }}
- 作者:{{ item.authors }}
- 通讯作者:{{ item.author }}
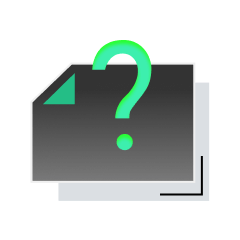
内容获取失败,请点击重试
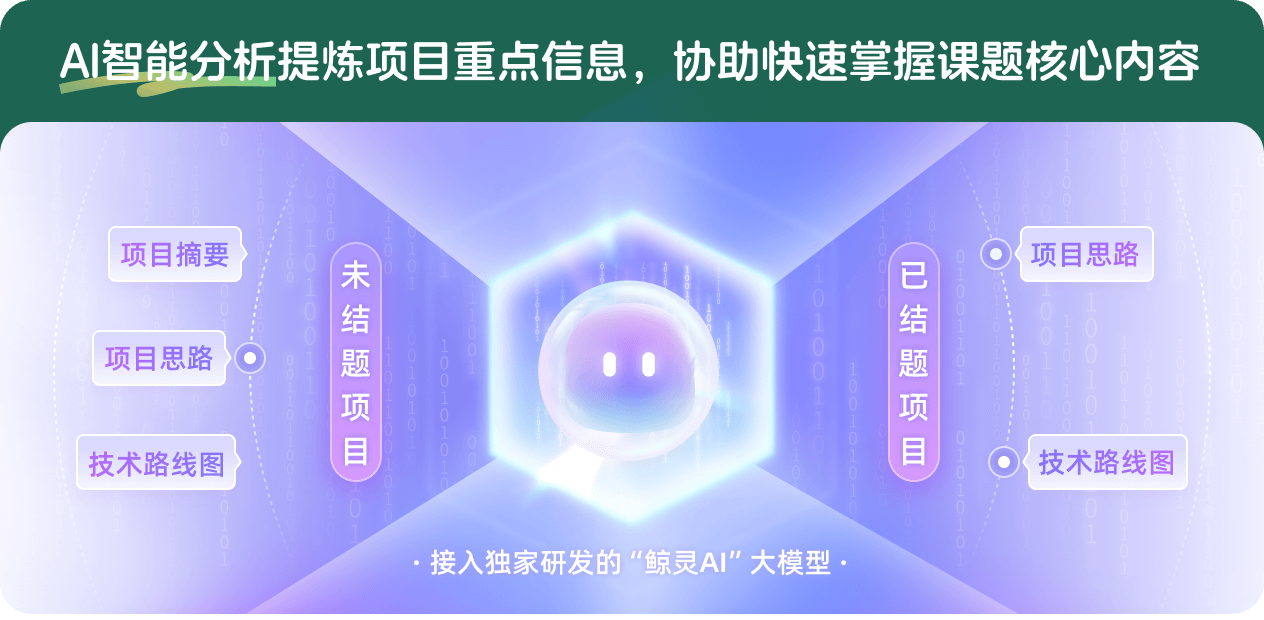
查看分析示例
此项目为已结题,我已根据课题信息分析并撰写以下内容,帮您拓宽课题思路:
AI项目摘要
AI项目思路
AI技术路线图
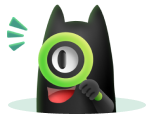
请为本次AI项目解读的内容对您的实用性打分
非常不实用
非常实用
1
2
3
4
5
6
7
8
9
10
您认为此功能如何分析更能满足您的需求,请填写您的反馈:
孙霞的其他基金
支持分层细粒度分面检索的分面分类树构建方法研究
- 批准号:61202184
- 批准年份:2012
- 资助金额:23.0 万元
- 项目类别:青年科学基金项目
相似国自然基金
{{ item.name }}
- 批准号:{{ item.ratify_no }}
- 批准年份:{{ item.approval_year }}
- 资助金额:{{ item.support_num }}
- 项目类别:{{ item.project_type }}
相似海外基金
{{
item.name }}
{{ item.translate_name }}
- 批准号:{{ item.ratify_no }}
- 财政年份:{{ item.approval_year }}
- 资助金额:{{ item.support_num }}
- 项目类别:{{ item.project_type }}