介孔柱撑粘土固体酸的催化界面调控及合成双酚F机制研究
项目介绍
AI项目解读
基本信息
- 批准号:51672077
- 项目类别:面上项目
- 资助金额:62.0万
- 负责人:
- 依托单位:
- 学科分类:E0205.无机非金属基复合材料
- 结题年份:2020
- 批准年份:2016
- 项目状态:已结题
- 起止时间:2017-01-01 至2020-12-31
- 项目参与者:欧恩才; 王龙禄; 张书渠; 刘冉; 吴献章; 陈席; 陈优;
- 关键词:
项目摘要
Bisphenol F is a new chemical raw material with a broad application prospect,while is still relying on importing so far in our country. The conventional production procecess using liquid protonic acids has some major drawbacks such as difficulties in the separation and recovery of pure product from the reaction medium, handling of the reagents problems due to corrosive nature of reagents and formation of inorganic wastes et al. Increasing concern for developing environment-friendly chemical process, several attempts have been reported to replace the conventional processes by using solid catalysts. Among them, zeolites showed promising activity for bisphenol synthesis, but a major problem associated with zeolites is low production and deactivation of the catalyst during the progress of the reaction. It is of great of importance to develop new solid acids with high activities and selectivity for the hydroxyalkylation. Applicant’s previous studies found that the interface features of the catalysts were closely related to the activities and distribution of the isomers, while the related systematic studies is still a blank page. This project plans to develop in-situ growth of mesoporous ZrO2 between the two-dimensional nanl clay layer to prepare mesoporous pillared clays for the first time, to monitor and control the apace size, active-sites and the proportion of B/L by using the catalyst in-situ characterization system, to furtherly study adsorption-catalysis synergy effect on the catalytic interface, to explore the control factors of catalyst activity and selectivity of catalysts, to clarify the interface process and mechanism of the catalytic interface such as mass transfer and electron tranport and to reveal the quantitative law beteeen the catalytic interface regulation and catalytic efficiency. These results will be the important theoretical bases and technological support for the design of low cost green catalysts with excellent performace, targeting to catalysing bisphenol F synthesis.
双酚F是一种应用前景巨大的新型化工原料,我国基本依赖进口。目前双酚F合成主要以液体质子酸为催化剂,存在环境污染、收率低及产物难分离等问题。开展固体酸催化合成双酚F研究成为该领域的热点,其中分子筛具有良好的催化活性,但存在产率低、易失活等缺点,开发高效固体酸催化剂是关键。申请者前期研究发现,固体酸催化剂的催化界面特性对双酚F合成的催化活性及异构体分布起关键作用,相关系统研究鲜见报道。本项目首次在粘土二维纳米片层间原位构筑规整ZrO2多孔柱,形成介孔柱撑结构。采用催化剂表征系统原位跟踪和调控催化剂的空间尺寸、活性位点分布以及B/L酸比例,深入研究催化界面的吸附–催化协同效应,探索催化剂反应活性与选择性控制因素,阐明催化界面的传质及电子转移等界面过程和机制,揭示催化界面调控与催化效率的量化规律。以上研究将为开发廉价、绿色、高效、适用于合成双酚F的固体酸催化剂提供一定的理论依据和技术支持。
结项摘要
项目围绕固体酸催化剂的构筑、催化材料表界面结构、催化界面调控与催化性能的探讨,以及双酚F催化合成机制等方面展开研究。构筑了一系列催化材料,研究了固体酸催化剂的制备、性能、酸化和柱撑改性,探索了催化剂反应活性与选择性的控制因素,研究发现原土对异构体呈现了选择性,杂多酸固载型催化剂具有良好的催化性能和高选择性;构筑了铝柱撑蒙脱土、铝掺杂SPC介孔材料,研究发现在不同体系合成双酚F反应中酸的强弱对催化剂催化活性起到决定性作用,Brönsted和Lewis酸位点的协同作用也是获得高双酚F产率的重要因素;构筑了介孔SO4/ZrO2催化材料,发现其有优良的催化烷基化反应性能,经酸化后,Brönsted酸位点显著增加;研究了不同ZrO2晶型对催化合成双酚F活性的影响,发现不同的晶型具有不同的比表面积、酸量和酸强,对合成双酚F催化活性也不同;通过柱撑法合成磷钨酸铁柱撑蒙脱土复合催化剂,发现其是介孔结构,并且通过表面柱撑粘土后能产生更多的微孔结构;制备了ZnFe2O4-ZrO2复合催化剂、TiO2/ZnO复合空心微球催化剂、ZnIn2S4纳米催化材料、铝掺杂氧化铁修饰的MgAl层状双氢氧化物纳米薄片、Fe1-xZnxS固溶体、三元固溶体FeNiS非均相催化剂等,研究其晶体结构、表面形态和催化界面性能,揭示催化界面特性调控规律;制备了MIL-101(Cr/Al)、MIL-101(Fe)-SO3H催化剂,发现花状形貌的材料边缘能暴露更多的活性位点、催化活性高,高效的催化性能是由于材料具有高酸性和高吸附性,优化工艺条件后,双酚F的产率可达97.1%,选择性可达98.3%;研究了在Sn-MCM-41分子筛催化合成双酚F体系中反应温度、反应时间、酚醛比、催化剂用量等的影响情况,发现最佳反应温度为90℃,酚醛比为10:1,反应时间120min,催化剂用量为0.01g•g-1。研究了硅酸钙对双酚F合成过程中异构体含量分布的影响,发现硅酸钙在双酚F的合成中具有良好的催化活性,并对2,2'-异构体的合成具有特异性。以上研究为开发廉价、绿色、高效、适用于合成双酚F的固体酸催化剂提供了一定的理论依据和技术支持。发表了SCI收录论文30篇,其中影响因子5.0以上24篇;培养了博士研究生3名,硕士研究生7名。
项目成果
期刊论文数量(30)
专著数量(0)
科研奖励数量(0)
会议论文数量(0)
专利数量(2)
Hollow Microsphere TiO2/ZnO p-n Heterojuction with High Photocatalytic Performance for 2,4-Dinitropheno Mineralization
具有高光催化性能的空心微球 TiO2/ZnO 异质结用于 2,4-二硝基酚矿化
- DOI:10.1142/s179329201750076x
- 发表时间:2017-06-01
- 期刊:NANO
- 影响因子:1.2
- 作者:Liu, Yutang;Cai, Tao;Xia, Xinnian
- 通讯作者:Xia, Xinnian
Rice husk derived double network hydrogel as efficient adsorbent for Pb(II), Cu(II) and Cd(II) removal in individual and multicomponent systems
稻壳衍生的双网络水凝胶作为单组分和多组分系统中 Pb(II)、Cu(II) 和 Cd(II) 去除的高效吸附剂
- DOI:10.1016/j.biortech.2019.121793
- 发表时间:2019-10-01
- 期刊:BIORESOURCE TECHNOLOGY
- 影响因子:11.4
- 作者:Ma, Jianhong;Li, Tong;Chen, Hui
- 通讯作者:Chen, Hui
Recent advances in round-the-clock photocatalytic system: Mechanisms, characterization techniques and applications
全天候光催化系统的最新进展:机理、表征技术和应用
- DOI:10.1016/j.jphotochemrev.2019.03.002
- 发表时间:2019-06-01
- 期刊:JOURNAL OF PHOTOCHEMISTRY AND PHOTOBIOLOGY C-PHOTOCHEMISTRY REVIEWS
- 影响因子:13.6
- 作者:Cai, Tao;Liu, Yutang;Zeng, Guangming
- 通讯作者:Zeng, Guangming
Ultrafine Ag@AgI nanoparticles on cube single-crystal Ag3PO4 (100): An all-day-active Z-Scheme photocatalyst for environmental purification
立方体单晶 Ag3PO4 上的超细 Ag@AgI 纳米颗粒 (100):用于环境净化的全天活性 Z 型光催化剂
- DOI:10.1016/j.jcis.2018.08.074
- 发表时间:2019
- 期刊:Journal of Colloid and Interface Science
- 影响因子:9.9
- 作者:Cai Tao;Liu Yutang;Wang Longlu;Zhang Shuqu;Dong Wanyue;Chen Hui;Ma Jianhong;Liu Chengbin;Luo Shenglian
- 通讯作者:Luo Shenglian
Mechanisms for photo assisted Fenton of synthesized pyrrhotite at neutral pH
中性pH下合成磁黄铁矿的光辅助芬顿反应机理
- DOI:10.1016/j.apsusc.2018.09.007
- 发表时间:2019-01-01
- 期刊:APPLIED SURFACE SCIENCE
- 影响因子:6.7
- 作者:Gao, Jing;Liu, Yutang;Dong, Wanyue
- 通讯作者:Dong, Wanyue
数据更新时间:{{ journalArticles.updateTime }}
{{
item.title }}
{{ item.translation_title }}
- DOI:{{ item.doi || "--"}}
- 发表时间:{{ item.publish_year || "--" }}
- 期刊:{{ item.journal_name }}
- 影响因子:{{ item.factor || "--"}}
- 作者:{{ item.authors }}
- 通讯作者:{{ item.author }}
数据更新时间:{{ journalArticles.updateTime }}
{{ item.title }}
- 作者:{{ item.authors }}
数据更新时间:{{ monograph.updateTime }}
{{ item.title }}
- 作者:{{ item.authors }}
数据更新时间:{{ sciAawards.updateTime }}
{{ item.title }}
- 作者:{{ item.authors }}
数据更新时间:{{ conferencePapers.updateTime }}
{{ item.title }}
- 作者:{{ item.authors }}
数据更新时间:{{ patent.updateTime }}
其他文献
含活性基团马来酰亚胺的RAFT聚合
- DOI:--
- 发表时间:--
- 期刊:湖南大学学报(自然科学版)
- 影响因子:--
- 作者:卢彦兵;孙丽丽;孙荣欣;夏新年;徐伟箭
- 通讯作者:徐伟箭
其他文献
{{
item.title }}
{{ item.translation_title }}
- DOI:{{ item.doi || "--" }}
- 发表时间:{{ item.publish_year || "--"}}
- 期刊:{{ item.journal_name }}
- 影响因子:{{ item.factor || "--" }}
- 作者:{{ item.authors }}
- 通讯作者:{{ item.author }}
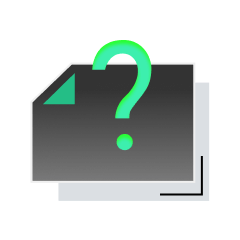
内容获取失败,请点击重试
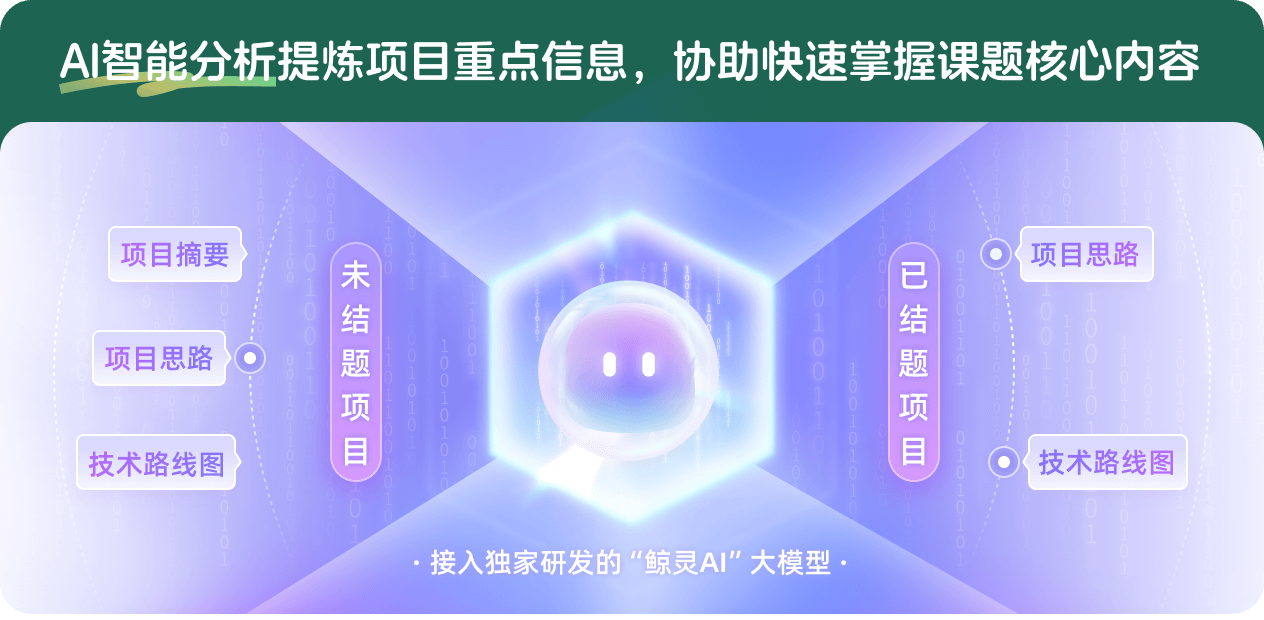
查看分析示例
此项目为已结题,我已根据课题信息分析并撰写以下内容,帮您拓宽课题思路:
AI项目摘要
AI项目思路
AI技术路线图
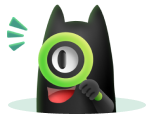
请为本次AI项目解读的内容对您的实用性打分
非常不实用
非常实用
1
2
3
4
5
6
7
8
9
10
您认为此功能如何分析更能满足您的需求,请填写您的反馈:
夏新年的其他基金
基于晶面可控修饰过渡金属硫化物光催化生物质转化协同高效析氢研究
- 批准号:
- 批准年份:2021
- 资助金额:58 万元
- 项目类别:面上项目
相似国自然基金
{{ item.name }}
- 批准号:{{ item.ratify_no }}
- 批准年份:{{ item.approval_year }}
- 资助金额:{{ item.support_num }}
- 项目类别:{{ item.project_type }}
相似海外基金
{{
item.name }}
{{ item.translate_name }}
- 批准号:{{ item.ratify_no }}
- 财政年份:{{ item.approval_year }}
- 资助金额:{{ item.support_num }}
- 项目类别:{{ item.project_type }}