基于动态节点运动的网络社区发现方法研究
项目介绍
AI项目解读
基本信息
- 批准号:61867005
- 项目类别:地区科学基金项目
- 资助金额:37.0万
- 负责人:
- 依托单位:
- 学科分类:F0610.交叉学科中的人工智能问题
- 结题年份:2022
- 批准年份:2018
- 项目状态:已结题
- 起止时间:2019-01-01 至2022-12-31
- 项目参与者:马海英; 陆耿; 闫芮; 谭宗元; 周健; 顾吉鹏;
- 关键词:
项目摘要
Complex networks are abstraction from the relations among real-world objectives. It is rather significant and challenging to study the structures of different complex networks. The analysis of complex network structure is essentially a problem of clustering for the vertices according to their similarities, so that community features can be detected. There exist various types of algorithms for community detection. However, most of them bear restrictions and relatively high computation complexity. Recently, our research team proposed a novel algorithm based on the motions of dynamical systems. The algorithm can achieve automatic community detection by employing the group consensus motions of the dynamic vertices within a common state space. Actually, this algorithm integrates the clustering problem of complex networks with the theory on systems dynamics and essentially belongs to a category of algorithms which are based on the exchange of information among data points. Evidently, potential theoretical and practical significances for such an algorithm are both prominent. Thus, rigorous and extensive studies aiming at relevant problems are deserved. The scheme of the current project will be tightly focused on the community detection algorithms based on motions of dynamic vertices, consisting of four major aspects: 1. research on the straight relationship between group consensus motions and the topological structures of networks; 2. research on the robustness of the community structure detected under a situation with uncertainty of the network topologies; 3. research on the algorithms under the specific scenario with the network topologies being slowly varying ; 4. research on the classification of natural languages of human based on the relevant algorithms.
复杂网络是现实中客体之间关系的抽象,对其结构和特征的研究具有意义和挑战性。复杂网络结构分析根本是根据节点的相似程度对它们进行聚类,从而实现社区发现。已有的各类社区发现算法大都有局限性,且计算复杂度普遍较高。课题组初步探讨了一种基于动态系统运动的新算法。该算法结合了系统动力学理论和复杂网络的聚类问题,能利用动态节点在状态空间中的分组趋同运动实现自动聚类。这类算法本质上属于基于网络节点之间信息交换的动态社区发现方法。相比其他算法,这种新算法具有显著的理论和现实意义,值得深入探究。本项目的计划研究内容将密切围绕基于动态系统运动的网络社区发现算法,分别在四个主要方面展开: 1. 研究基于动态系统运动的社区发现与网络拓扑结构之间的直接关联; 2. 研究网络拓扑结构具有不确定性情况下的社区结构鲁棒性; 3. 研究网络拓扑慢时变情况下的动态社区发现算法; 4. 基于社区发现算法,研究自然语言系谱分类。
结项摘要
社区发现问题本质上是对具有一定同质性的个体子系统进行归类。本项目专注于通过个体子系统的运动以及子系统之间进行信息交互动态地实现分类。趋同既是复杂多个体系统聚类运动的基础,也是聚类运动的极端情况。项目组从群镇定控制和网络能控性的角度对约束条件下的趋同控制器设计方法开展了研究。编队属于精细化的准趋同类型,实现难度更大。项目组主要基于虚拟结构法,对具有不确定性和时变性的复杂多个体系统的分组编队控制方法开展了研究。项目组还研究了如何利用动态聚类基于人类个体行为动力学针对具体社会现象进行分类的方法以及心电信号与心血管疾病表征与分类方法。研究工作取得一系列成果,发表了13篇期刊论文,2篇会议论文,培养研究生10名。
项目成果
期刊论文数量(13)
专著数量(0)
科研奖励数量(0)
会议论文数量(2)
专利数量(0)
Limited-Budget Output Consensus for Descriptor Multiagent Systems With Energy Constraints
具有能量约束的描述符多智能体系统的有限预算输出共识
- DOI:10.1109/tcyb.2019.2963172
- 发表时间:2020-11-01
- 期刊:IEEE TRANSACTIONS ON CYBERNETICS
- 影响因子:11.8
- 作者:Xi, Jianxiang;Wang, Cheng;Yang, Bailong
- 通讯作者:Yang, Bailong
Event-triggered finite-time consensualization for multiagent networks with limited energy budgets
能量预算有限的多智能体网络的事件触发有限时间共识
- DOI:10.1007/s11432-019-2683-3
- 发表时间:2020-04
- 期刊:Science China – Information Sciences
- 影响因子:--
- 作者:Le WANG;Jianxiang XI;Dangbo DU;Guangbin LIU
- 通讯作者:Guangbin LIU
Robust time-varying formation design for multiagent systems with disturbances: Extended-state-observer method
具有扰动的多智能体系统的鲁棒时变编队设计:扩展状态观察者方法
- DOI:10.1002/rnc.4941
- 发表时间:2020-03-01
- 期刊:INTERNATIONAL JOURNAL OF ROBUST AND NONLINEAR CONTROL
- 影响因子:3.9
- 作者:Wang, Le;Xi, Jianxiang;Liu, Guangbin
- 通讯作者:Liu, Guangbin
Output formation tracking for networked systems with limited energy and aperiodic silence
具有有限能量和非周期性静默的网络系统的输出形成跟踪
- DOI:10.1016/j.cja.2021.10.035
- 发表时间:2022-07-01
- 期刊:CHINESE JOURNAL OF AERONAUTICS
- 影响因子:5.7
- 作者:Jin, Jin;Li, Junlong;Cai, Ning
- 通讯作者:Cai, Ning
数据更新时间:{{ journalArticles.updateTime }}
{{
item.title }}
{{ item.translation_title }}
- DOI:{{ item.doi || "--"}}
- 发表时间:{{ item.publish_year || "--" }}
- 期刊:{{ item.journal_name }}
- 影响因子:{{ item.factor || "--"}}
- 作者:{{ item.authors }}
- 通讯作者:{{ item.author }}
数据更新时间:{{ journalArticles.updateTime }}
{{ item.title }}
- 作者:{{ item.authors }}
数据更新时间:{{ monograph.updateTime }}
{{ item.title }}
- 作者:{{ item.authors }}
数据更新时间:{{ sciAawards.updateTime }}
{{ item.title }}
- 作者:{{ item.authors }}
数据更新时间:{{ conferencePapers.updateTime }}
{{ item.title }}
- 作者:{{ item.authors }}
数据更新时间:{{ patent.updateTime }}
其他文献
用于加速器电源的一种矩阵变换器拓扑研究
- DOI:--
- 发表时间:2016
- 期刊:原子能科学技术
- 影响因子:--
- 作者:王斌;高大庆;刁晨;宋卫章;史春逢
- 通讯作者:史春逢
其他文献
{{
item.title }}
{{ item.translation_title }}
- DOI:{{ item.doi || "--" }}
- 发表时间:{{ item.publish_year || "--"}}
- 期刊:{{ item.journal_name }}
- 影响因子:{{ item.factor || "--" }}
- 作者:{{ item.authors }}
- 通讯作者:{{ item.author }}
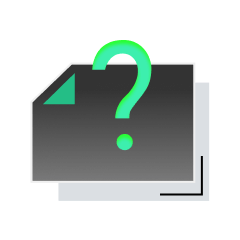
内容获取失败,请点击重试
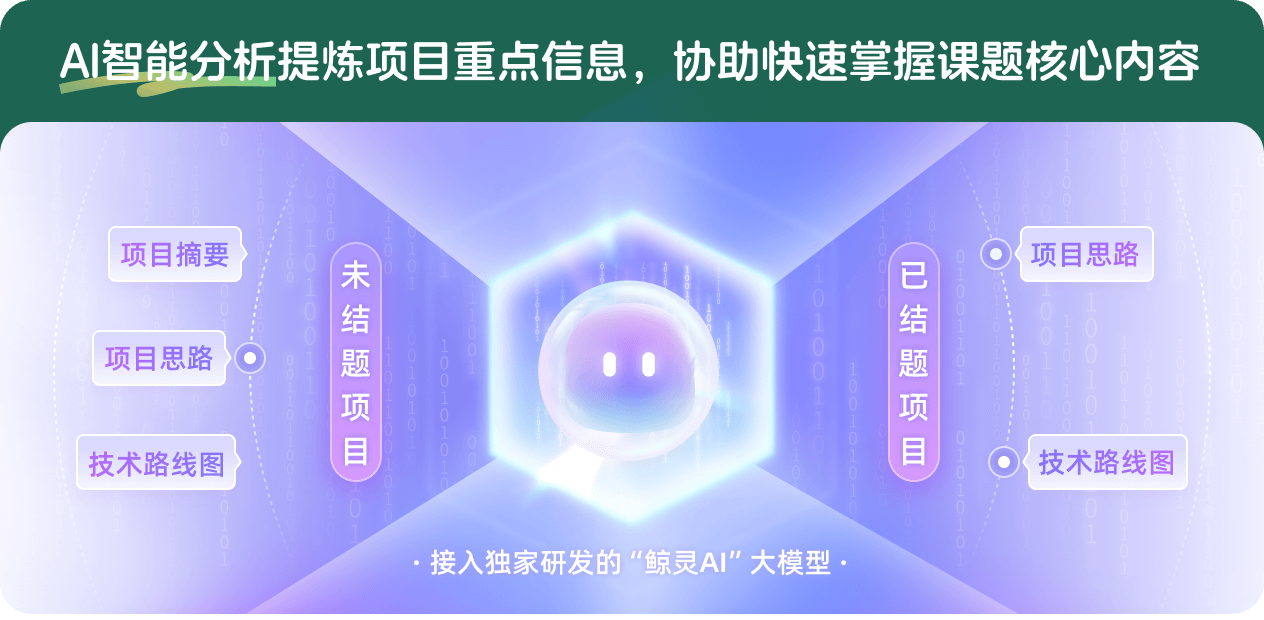
查看分析示例
此项目为已结题,我已根据课题信息分析并撰写以下内容,帮您拓宽课题思路:
AI项目摘要
AI项目思路
AI技术路线图
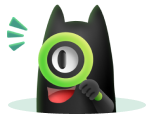
请为本次AI项目解读的内容对您的实用性打分
非常不实用
非常实用
1
2
3
4
5
6
7
8
9
10
您认为此功能如何分析更能满足您的需求,请填写您的反馈:
相似国自然基金
{{ item.name }}
- 批准号:{{ item.ratify_no }}
- 批准年份:{{ item.approval_year }}
- 资助金额:{{ item.support_num }}
- 项目类别:{{ item.project_type }}
相似海外基金
{{
item.name }}
{{ item.translate_name }}
- 批准号:{{ item.ratify_no }}
- 财政年份:{{ item.approval_year }}
- 资助金额:{{ item.support_num }}
- 项目类别:{{ item.project_type }}