基于视-听觉认知的对话类视频压缩编码
项目介绍
AI项目解读
基本信息
- 批准号:61902401
- 项目类别:青年科学基金项目
- 资助金额:28.0万
- 负责人:
- 依托单位:
- 学科分类:F0210.计算机图像视频处理与多媒体技术
- 结题年份:2022
- 批准年份:2019
- 项目状态:已结题
- 起止时间:2020-01-01 至2022-12-31
- 项目参与者:--
- 关键词:
项目摘要
Recent years, along with the rapid development of multimedia communication technology, various video service and application appeared constantly. Dialogic video, as a main class of online videos, has ever-increasing video traffic. However, the computing resource and bandwidth resource are hard to meet the demand. In order to enhance the video communication efficiency and user experience, and solve the problems of the resource limitation, this project will research visual-audio cognition based dialogic video compression. Namely, this project will utilize the theory of human visual-audio cognition to build a perceptual model. And based on the perceptual model, a perceptual video coding method will be proposed to reduce the perceptual redundancy and improve video coding efficiency. Concretely, this project will conduct the interdisciplinary research of cognition, computer vision and signal processing. The main content of this project includes: (1) Perception database and human perceptual behavior analysis for dialogic video; (2) Visual-audio multimodal based perceptual model; (3) Knowledge distillation via instance relationship graph; (4) Perceptual model based rate-perceptual distortion coding optimization method. This project aims to not only the research of key technology but also the practical application and it devotes to facilitating the development of multimedia communication and video service technology.
近年来,随着多媒体通信技术飞速发展,各类视频服务和应用不断涌现。对话类视频作为在线视频的主要类别,视频流量不断增长,而计算资源与网络带宽资源难以完全满足此现状。为了提升对话类视频通信的效率和用户体验,解决资源受限问题,本项目研究基于视-听觉认知的对话类视频压缩编码,即充分挖掘人类视-听觉认知机理,构建视频感知模型来预测人类观看对话类视频的关注区域,并基于此建立感知视频压缩编码方法,降低视频的感知冗余、提升编码效率。具体地,本项目拟重点开展认知学、计算机视觉与信号处理交叉理论的研究,研究内容包括:(1)对话类视频感知数据库与人类观看视频的感知行为分析;(2)面向视-听觉多模态的感知模型;(3)基于样本关系图的模型蒸馏方法;(4)基于感知模型的对话类视频率-感知失真编码优化方法。本项目不仅致力于关键技术的理论研究,也向实际应用推进,促进多媒体通信和视频服务技术的发展。
结项摘要
近年来,随着多媒体通信技术飞速发展,各类视频服务和应用不断涌现。对话类视频作为在线视频的主要类别,视频流量不断增长,而计算资源与网络带宽资源难以完全满足此现状。为了提升对话类视频通信的效率和用户体验,解决资源受限问题,本项目研究基于视-听觉认知的对话类视频压缩编码,即充分挖掘人类视-听觉认知机理,构建视频感知模型来预测人类观看对话类视频的关注区域,并基于此建立感知视频压缩编码方法,降低视频的感知冗余、提升编码效率。具体地,本项目重点开展认知学、计算机视觉与信号处理交叉理论的研究,并按照项目研究计划按时完成了相应的研究工作,包括:(1)对话类视频感知数据库与人类观看视频的感知行为分析;(2)面向视-听觉多模态的感知模型(3)基于样本关系图的模型蒸馏方法(4)基于感知模型的对话类视频率-感知失真编码优化方法。..该项目完成所有研究内容,并达到预期指标和论文、专利的发表,具体研究成果如下:.(1) 构建对话类视频感知数据库,包含300个视频,146,529帧,5,013,980个关注点,便于相关学者进行研究和测试。对数据进行人类感知行为分析,获得了多个发现用于启发后续算法的设计;.(2)提出了一种面向视-听觉多模态的感知模型AVM-Net,预测人类观看对话类视频时的关注区域和声源定位。在测试实例上预测的显著性图与人类观看视频的真实注意力热图之间的相关系数(Correlation Coefficient, CC)已达到0.75,标准化扫描路径显著性(Normalized Scanpath Saliency, NSS)达到4.14;.(3) 提出了模型压缩与蒸馏学习方法,提出样本关系图能从高性能教师网络蒸馏出更充分的知识,并提出跨架构知识蒸馏技术,使蒸馏学习不再受限于教师与学生网络的架构差异,从而便于深度模型嵌入到计算资源受限的设备中。在模型大小减少到四分之一的情况下,精度下降未超过两个百分点; .(4) 提出一种基于感知模型的对话类视频率-失真编码优化方法,大幅减少视频感知冗余,提升编码效率。在码率一定的情况下,压缩视频的感知质量较传统方法提升了2-3dB; .(5) 在国内外重要学术期刊(如PAMI、IJCV等)与顶级会议论文(如CVPR、ICCV、ECCV等)中发表论文16篇,申请发明专利5项。
项目成果
期刊论文数量(7)
专著数量(0)
科研奖励数量(0)
会议论文数量(8)
专利数量(5)
EDP: An Efficient Decomposition and Pruning Scheme for Convolutional Neural Network Compression
EDP:一种高效的卷积神经网络压缩分解和剪枝方案
- DOI:10.1109/tnnls.2020.3018177
- 发表时间:2020-11
- 期刊:IEEE Transactions on Neural Networks and Learning Systems
- 影响因子:10.4
- 作者:Xiaofeng Ruan;Yufan Liu;Chunfeng Yuan;Bing Li;Weiming Hu;Yangxi Li;Stephen Maybank
- 通讯作者:Stephen Maybank
Web Objectionable Video Recognition Based on Deep Multi-Instance Learning With Representative Prototypes Selection
基于深度多实例学习和代表性原型选择的网络不良视频识别
- DOI:10.1109/tcsvt.2020.2992276
- 发表时间:2021-03
- 期刊:IEEE Transactions on Circuits and Systems for Video Technology
- 影响因子:8.4
- 作者:Xinmiao Ding;Bing Li;Yangxi Li;Wen Guo;Yao Liu;Weihua Xiong;Weiming Hu
- 通讯作者:Weiming Hu
基于动态稀疏和特征学习增强的模型剪枝
- DOI:10.1360/sst-2021-0088
- 发表时间:2021-09
- 期刊:中国科学
- 影响因子:--
- 作者:Xiaofeng Ruan;Weiming Hu;Yufan Liu;Bing Li
- 通讯作者:Bing Li
Learning to Explore Distillability and Sparsability: A Joint Framework for Model Compression
学习探索可蒸馏性和可稀疏性:模型压缩的联合框架
- DOI:10.1109/tpami.2022.3185317
- 发表时间:2022-06
- 期刊:IEEE Transactions on Pattern Analysis and Machine Intelligence (T-PAMI)
- 影响因子:--
- 作者:Yufan Liu;Jiajiong Cao;Bing Li;Weiming Hu;Stephen Maybank
- 通讯作者:Stephen Maybank
数据更新时间:{{ journalArticles.updateTime }}
{{
item.title }}
{{ item.translation_title }}
- DOI:{{ item.doi || "--"}}
- 发表时间:{{ item.publish_year || "--" }}
- 期刊:{{ item.journal_name }}
- 影响因子:{{ item.factor || "--"}}
- 作者:{{ item.authors }}
- 通讯作者:{{ item.author }}
数据更新时间:{{ journalArticles.updateTime }}
{{ item.title }}
- 作者:{{ item.authors }}
数据更新时间:{{ monograph.updateTime }}
{{ item.title }}
- 作者:{{ item.authors }}
数据更新时间:{{ sciAawards.updateTime }}
{{ item.title }}
- 作者:{{ item.authors }}
数据更新时间:{{ conferencePapers.updateTime }}
{{ item.title }}
- 作者:{{ item.authors }}
数据更新时间:{{ patent.updateTime }}
其他文献
其他文献
{{
item.title }}
{{ item.translation_title }}
- DOI:{{ item.doi || "--" }}
- 发表时间:{{ item.publish_year || "--"}}
- 期刊:{{ item.journal_name }}
- 影响因子:{{ item.factor || "--" }}
- 作者:{{ item.authors }}
- 通讯作者:{{ item.author }}
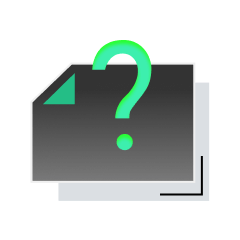
内容获取失败,请点击重试
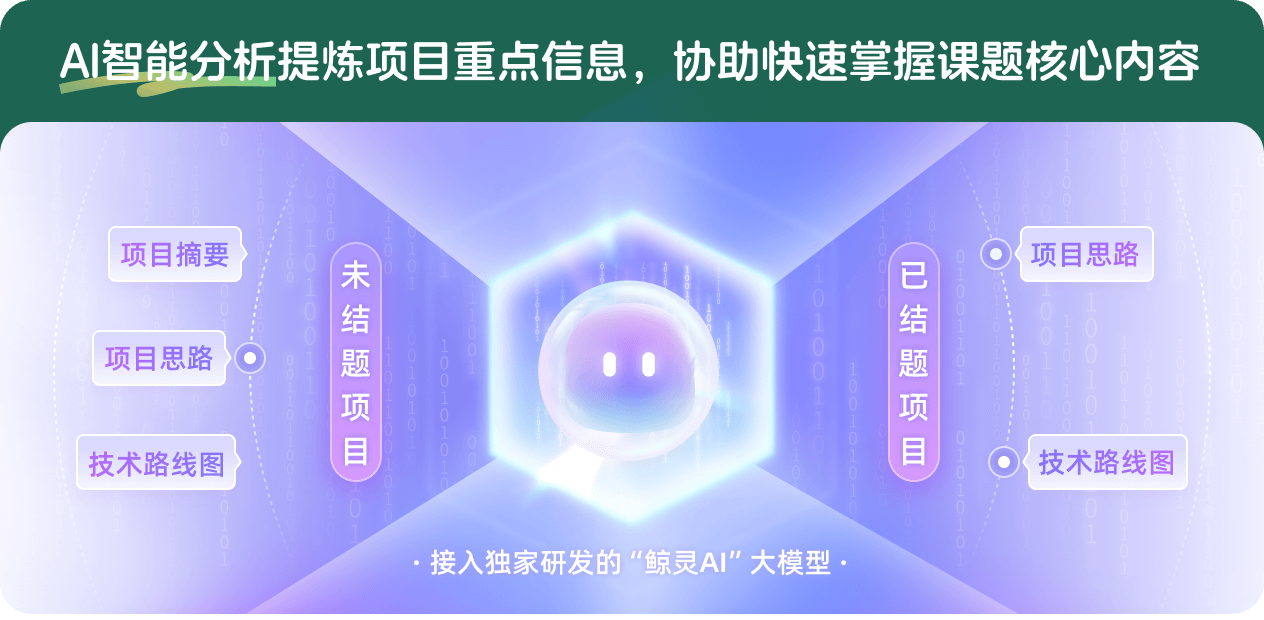
查看分析示例
此项目为已结题,我已根据课题信息分析并撰写以下内容,帮您拓宽课题思路:
AI项目摘要
AI项目思路
AI技术路线图
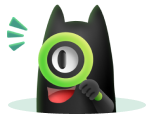
请为本次AI项目解读的内容对您的实用性打分
非常不实用
非常实用
1
2
3
4
5
6
7
8
9
10
您认为此功能如何分析更能满足您的需求,请填写您的反馈:
刘雨帆的其他基金
面向高通量互联网视频的高效内容理解与安全分析研究
- 批准号:62372451
- 批准年份:2023
- 资助金额:52.00 万元
- 项目类别:面上项目
相似国自然基金
{{ item.name }}
- 批准号:{{ item.ratify_no }}
- 批准年份:{{ item.approval_year }}
- 资助金额:{{ item.support_num }}
- 项目类别:{{ item.project_type }}
相似海外基金
{{
item.name }}
{{ item.translate_name }}
- 批准号:{{ item.ratify_no }}
- 财政年份:{{ item.approval_year }}
- 资助金额:{{ item.support_num }}
- 项目类别:{{ item.project_type }}