软件缺陷预测的度量元有效性及建模算法研究
项目介绍
AI项目解读
基本信息
- 批准号:61602534
- 项目类别:青年科学基金项目
- 资助金额:20.0万
- 负责人:
- 依托单位:
- 学科分类:F0202.系统软件、数据库与工业软件
- 结题年份:2019
- 批准年份:2016
- 项目状态:已结题
- 起止时间:2017-01-01 至2019-12-31
- 项目参与者:轩辕哲; 董崇武; 谢伟; 贾殷; 吕亚云;
- 关键词:
项目摘要
With more and more attention paid to software quality, metric-based software defect prediction has become a research hotspot in the field of software engineering. Software defect prediction employs software metrics to construct prediction models, in order to predict defect information of new software modules. The predicted defect information can both reflect the quality of software modules and help to allocate software testing resources. .Currently, there exist some problems in software defect prediction. For example, only a few metrics are efficient for constructing models and the constructed models are much worse than the ideal models. In order to solve these problems, we research on the effectiveness of metrics and model construction algorithms. Firstly, we are aiming at finding new software metrics related to defect prediction, by analyzing the relationship between metrics and defects, in order to provide data which can better distinguish defect-prone modules and defect-free modules. Secondly, we research and provide a goal-driven metric selection method based on the effectiveness and redundancy, in order to select metrics which better fit the application of models. Finally, we research on the model construction algorithms with limited available testing resources. We propose a multi-objective approach to construct software defect prediction models with classification task under the condition of limited available testing resources. We also make some improvements on the previous learning-to-rank approach, in order to construct better software defect prediction models with ranking task. The research of this project will promote the solutions of a series of key problems of software defect prediction, and lay a solid foundation for the application and popularization of defect prediction models in the practical software engineering.
随着软件质量备受关注,基于度量元的软件缺陷预测成为软件工程领域的研究热点,它利用软件模块的各种特征构造模型,预测新模块的缺陷信息。目前软件缺陷预测存在有效度量元较少、构造的模型与理想模型差距较大等问题。为解决这些问题,本课题拟针对度量元的有效性及建模算法进行研究。首先,针对软件缺陷预测有效度量元不足的现状,分析软件模块的各种因素对缺陷的影响,将尚未用于预测但对缺陷影响较大的因素作为新度量元,提供更能区分缺陷的数据。其次,结合模型的应用目的,提出基于有效性及冗余度的目标驱动度量元选择方法。最后,研究可用测试资源有限时的建模算法,提出可用测试资源有限的情况下分类任务缺陷预测的多目标算法,并针对现有缺陷预测排序学习算法的不足提出改进方案,提高预测模型的性能,并给出相应的资源分配策略。本项目的研究将推动解决软件缺陷预测的一系列关键问题,为缺陷预测模型在实际软件工程的应用和推广打下坚实的基础。
结项摘要
基于度量元的软件缺陷预测利用软件模块的度量元信息构造预测模型,用于预测新模块的缺陷信息,这些缺陷信息不但能反映软件模块的质量,还能用于指导测试资源的分配,因此具有较好的应用前景。目前,软件缺陷预测相关的公开数据集有较多的噪音度量元,构造的预测模型与理想模型还有较大差距。因此,本课题针对度量元的有效性及建模算法进行研究。首先,针对排序任务软件缺陷预测度量元分析较为缺乏的现状,分析公开数据集的度量元有效性以及分析度量元对排序任务缺陷预测的影响,并结合模型的应用目的,提出基于有效性及冗余度的目标驱动度量元选择方法;其次,研究可用测试资源有限时的建模算法,提出可用测试资源有限的情况分类任务缺陷预测的多目标算法,并比较分类任务软件缺陷预测的多目标算法与单目标算法;最后,针对现有缺陷预测排序学习算法的不足提出改进方案,提高预测模型的性能。.具体而言,本课题主要完成了以下成果:1)提出一种目标驱动的度量元选择方法,并分析跨版本数据集的度量元有效性,找出较为有效的一些度量元;2)使用效果、代价以及二者的折中作为优化目标,更合理地比较分类任务软件缺陷预测的多目标学习算法和单目标学习算法,从而指导实际情况的算法选择;3)提出使用岭回归和lasso回归方法构造软件缺陷预测回归模型,从而更好地解决软件缺陷预测数据集中的多重共线性问题;4)提出基于排序学习方法的集成算法,从而改进现有软件缺陷预测的排序学习算法,提高预测模型的排序性能;5)提出构造软件缺陷预测模型的多目标学习算法,不但可以得到一组在排序性能、预测精度上各有优势的多样化的预测模型,以供不同的应用场景使用,并且这些模型的复杂度更低。
项目成果
期刊论文数量(1)
专著数量(0)
科研奖励数量(0)
会议论文数量(3)
专利数量(1)
Ridge and Lasso Regression Models for Cross-Version Defect Prediction
用于跨版本缺陷预测的岭回归模型和套索回归模型
- DOI:10.1109/tr.2018.2847353
- 发表时间:2018
- 期刊:IEEE Transactions on Reliability
- 影响因子:5.9
- 作者:Xiaoxing Yang;Wushao Wen
- 通讯作者:Wushao Wen
数据更新时间:{{ journalArticles.updateTime }}
{{
item.title }}
{{ item.translation_title }}
- DOI:{{ item.doi || "--"}}
- 发表时间:{{ item.publish_year || "--" }}
- 期刊:{{ item.journal_name }}
- 影响因子:{{ item.factor || "--"}}
- 作者:{{ item.authors }}
- 通讯作者:{{ item.author }}
数据更新时间:{{ journalArticles.updateTime }}
{{ item.title }}
- 作者:{{ item.authors }}
数据更新时间:{{ monograph.updateTime }}
{{ item.title }}
- 作者:{{ item.authors }}
数据更新时间:{{ sciAawards.updateTime }}
{{ item.title }}
- 作者:{{ item.authors }}
数据更新时间:{{ conferencePapers.updateTime }}
{{ item.title }}
- 作者:{{ item.authors }}
数据更新时间:{{ patent.updateTime }}
其他文献
其他文献
{{
item.title }}
{{ item.translation_title }}
- DOI:{{ item.doi || "--" }}
- 发表时间:{{ item.publish_year || "--"}}
- 期刊:{{ item.journal_name }}
- 影响因子:{{ item.factor || "--" }}
- 作者:{{ item.authors }}
- 通讯作者:{{ item.author }}
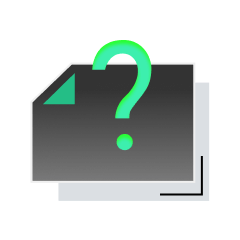
内容获取失败,请点击重试
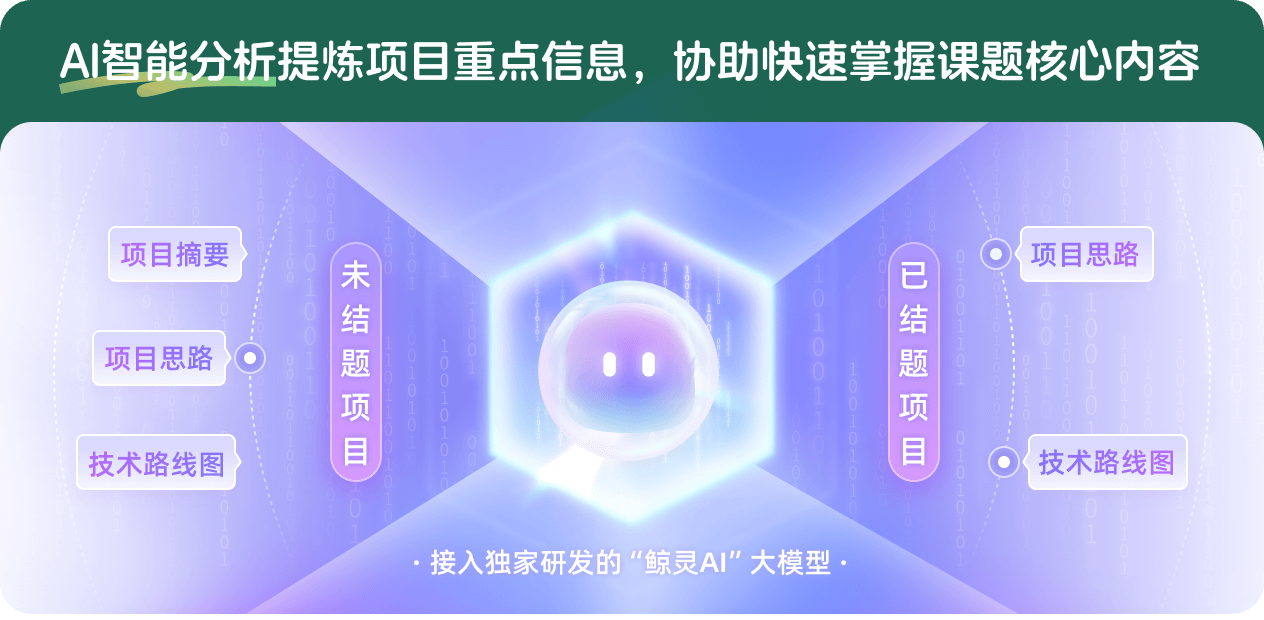
查看分析示例
此项目为已结题,我已根据课题信息分析并撰写以下内容,帮您拓宽课题思路:
AI项目摘要
AI项目思路
AI技术路线图
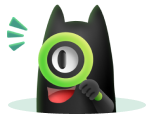
请为本次AI项目解读的内容对您的实用性打分
非常不实用
非常实用
1
2
3
4
5
6
7
8
9
10
您认为此功能如何分析更能满足您的需求,请填写您的反馈:
相似国自然基金
{{ item.name }}
- 批准号:{{ item.ratify_no }}
- 批准年份:{{ item.approval_year }}
- 资助金额:{{ item.support_num }}
- 项目类别:{{ item.project_type }}
相似海外基金
{{
item.name }}
{{ item.translate_name }}
- 批准号:{{ item.ratify_no }}
- 财政年份:{{ item.approval_year }}
- 资助金额:{{ item.support_num }}
- 项目类别:{{ item.project_type }}