RNA单分子检测信号的碱基特征识别方法研究
项目介绍
AI项目解读
基本信息
- 批准号:61803157
- 项目类别:青年科学基金项目
- 资助金额:25.0万
- 负责人:
- 依托单位:
- 学科分类:F0305.生物、医学信息系统与技术
- 结题年份:2021
- 批准年份:2018
- 项目状态:已结题
- 起止时间:2019-01-01 至2021-12-31
- 项目参与者:李冬冬; 堵威; 宋冰; 吴粤; 江超; 杨铖宇; 林鑫辰; 黄昊杰;
- 关键词:
项目摘要
RNA single molecule nanopore detection technology has been considered as one the novelist and burgeoning detection technology in the fields of genomics. However, on account of the limitation of sensitivity and accuracy caused by existing equipment and laboratory techniques, the current variation among the basic groups of RNA is still minute and the noise is still relative high, which leads the high identification error of RNA bases and bases modifications. Therefore, it is necessary to establish a machine learning based identification method in order to enhance the performance of nanopore detection in terms of detection accuracy...Hence, in this project, we aim to solve the problem of the low signal to noise ratio of RNA single molecule nanopore detection technology and develop an novel signal-noise separation method which based on time-frequency domain transformation and wavelet analysis. Then, focusing on the mapping problem caused by large-scale, high dimension and non-linearity of nanopore genetic data, in this project, it is plan to develop an efficient nonlinear dimension reduction method based on t-distributed stochastic neighbor embedding (t-SNE). Thirdly, in order to recognize and classify the bases and bases modifications under the situations of class-imbalance in terms of features of gene bases, it is planed to propose an feature recognition and classification methods based on class-imbalance learning and long-short term memory (LSTM) network. Finally, all the proposed methods will be integrated into a multiplex cancer microRNAs detection framework, to enhance the performance of RNA single molecule nanopore detection in the filed of bases modifications identifications.
RNA单分子纳米孔检测技术作为目前基因组学中新兴的检测技术。但是,由于现有仪器和实验技术测量灵敏度的限制,碱基间电流变化微弱、噪声高使得现有碱基识别错误率较高,亟需利用机器学习手段提高纳米孔检测方法的准确率。.因此,本项目针对RNA单分子检测的低信噪比问题,拟研究基于时频域变换及小波分解的信噪分离方法;针对纳米孔检测信号大规模高维非线性问题,研究基于随机邻域嵌入的非线性映射降维方法;针对基因数据的特征数据量不平衡、碱基修饰特征无法有效识别的问题,研究基于长短期记忆网络和不平衡学习的特征识别方法,最终集成一套能够用于癌症microRNA分子碱基特征识别的机器学习综合策略,提高纳米孔检测的准确率。
结项摘要
以纳米孔检测技术及X射线衍射透镜为代表的高通量分析筛选技术(High Throughput Screen, HTS)是有机结合分子生物学、细胞生物学、计算机、自动化控制等高新技术而形成的新的技术体系。该技术使得同一时间内进行大量的并行实验成为现实。然而由于高通量筛选电学信号的产生机理和数据特征,通过人工知己分析数据具有很大的困难和挑战。同时机器学习等人工智能方法为大批次高通量的数据高效分析提供了条件。本项目围绕电学信号的低信噪比问题,针对高维信号中存在的低信噪比、数据降维和特征提取问题、时频域分析和特征识别问题进行了系统性的研究,并取得相关研究成果。
项目成果
期刊论文数量(11)
专著数量(0)
科研奖励数量(0)
会议论文数量(0)
专利数量(0)
Variable-Scale Probabilistic Just-in-Time Learning for Soft Sensor Development with Missing Data
用于具有缺失数据的软传感器开发的可变尺度概率即时学习
- DOI:10.1021/acs.iecr.9b06113
- 发表时间:2020-03-18
- 期刊:INDUSTRIAL & ENGINEERING CHEMISTRY RESEARCH
- 影响因子:4.2
- 作者:Huang, Haojie;Peng, Xin;Zhong, Weimin
- 通讯作者:Zhong, Weimin
A Knowledge Base System for Operation Optimization: Design and Implementation Practice for the Polyethylene Process
操作优化知识库系统:聚乙烯工艺的设计与实施实践
- DOI:10.1016/j.eng.2019.09.004
- 发表时间:2019-12
- 期刊:Engineering
- 影响因子:12.8
- 作者:Weimin Zhong;Chaoyuan Li;Xin Peng;Feng Wan;Xufeng An;Zhou Tian
- 通讯作者:Zhou Tian
Key performance index estimation based on ensemble locally weighted partial least squares and its application on industrial nonlinear processes
基于集合局部加权偏最小二乘的关键性能指标估计及其在工业非线性过程中的应用
- DOI:10.1016/j.chemolab.2020.104031
- 发表时间:2020-08
- 期刊:Chemometrics and Intelligent Laboratory Systems
- 影响因子:3.9
- 作者:Chen Xin;Zhong Weimin;Jiang Chao;Li Zhi;Peng Xin;Cheng Hui
- 通讯作者:Cheng Hui
Enhanced moving horizon Bayesian-based fault diagnosis for multisampling rate data in a plantwide process
增强型基于移动水平贝叶斯的故障诊断,适用于全厂过程中的多采样率数据
- DOI:10.1016/j.measurement.2020.108200
- 发表时间:2020
- 期刊:Measurement
- 影响因子:5.6
- 作者:Ying Tian;Xin Peng;Zhong Yin
- 通讯作者:Zhong Yin
Concurrent Quality-Relevant Canonical Correlation Analysis for Nonlinear Continuous Process Decomposition and Monitoring
用于非线性连续过程分解和监控的并行质量相关典型相关分析
- DOI:10.1021/acs.iecr.0c00895
- 发表时间:2020-04
- 期刊:Industrial & Engineering Chemistry Research
- 影响因子:4.2
- 作者:Peng Xin;Li Zhi;Zhong Weimin;Qian Feng;Tian Ying
- 通讯作者:Tian Ying
数据更新时间:{{ journalArticles.updateTime }}
{{
item.title }}
{{ item.translation_title }}
- DOI:{{ item.doi || "--"}}
- 发表时间:{{ item.publish_year || "--" }}
- 期刊:{{ item.journal_name }}
- 影响因子:{{ item.factor || "--"}}
- 作者:{{ item.authors }}
- 通讯作者:{{ item.author }}
数据更新时间:{{ journalArticles.updateTime }}
{{ item.title }}
- 作者:{{ item.authors }}
数据更新时间:{{ monograph.updateTime }}
{{ item.title }}
- 作者:{{ item.authors }}
数据更新时间:{{ sciAawards.updateTime }}
{{ item.title }}
- 作者:{{ item.authors }}
数据更新时间:{{ conferencePapers.updateTime }}
{{ item.title }}
- 作者:{{ item.authors }}
数据更新时间:{{ patent.updateTime }}
其他文献
大鼠尾椎间盘退变不同时期Hippo信号差异性研究
- DOI:10.3969/j.issn.1004-406x.2019.12.07
- 发表时间:2019
- 期刊:中国脊柱脊髓杂志
- 影响因子:--
- 作者:张聪;王锋;彭鑫;吴小涛
- 通讯作者:吴小涛
靶向同源重组修复的抗肿瘤研究进展
- DOI:10.16438/j.0513-4870.2020-0624
- 发表时间:2020
- 期刊:药学学报
- 影响因子:--
- 作者:钟振兴;彭鑫;孔德新
- 通讯作者:孔德新
基于图卷积网络的多标签食品原材料识别
- DOI:--
- 发表时间:2019
- 期刊:南京信息工程大学学报
- 影响因子:--
- 作者:李辉;闵巍庆;王致岭;彭鑫
- 通讯作者:彭鑫
基于适应性构件模型的软件产品线设计和实现(英文)
- DOI:--
- 发表时间:--
- 期刊:计算机科学与探索
- 影响因子:--
- 作者:彭鑫;赵文耘;杨益明
- 通讯作者:杨益明
基于应用描述的 Android代码自动生成
- DOI:--
- 发表时间:2016
- 期刊:计算机应用与软件
- 影响因子:--
- 作者:江逸舟;沈立炜;彭鑫;赵文耘
- 通讯作者:赵文耘
其他文献
{{
item.title }}
{{ item.translation_title }}
- DOI:{{ item.doi || "--" }}
- 发表时间:{{ item.publish_year || "--"}}
- 期刊:{{ item.journal_name }}
- 影响因子:{{ item.factor || "--" }}
- 作者:{{ item.authors }}
- 通讯作者:{{ item.author }}
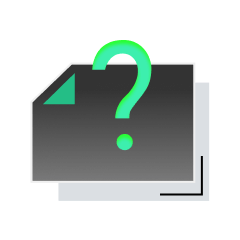
内容获取失败,请点击重试
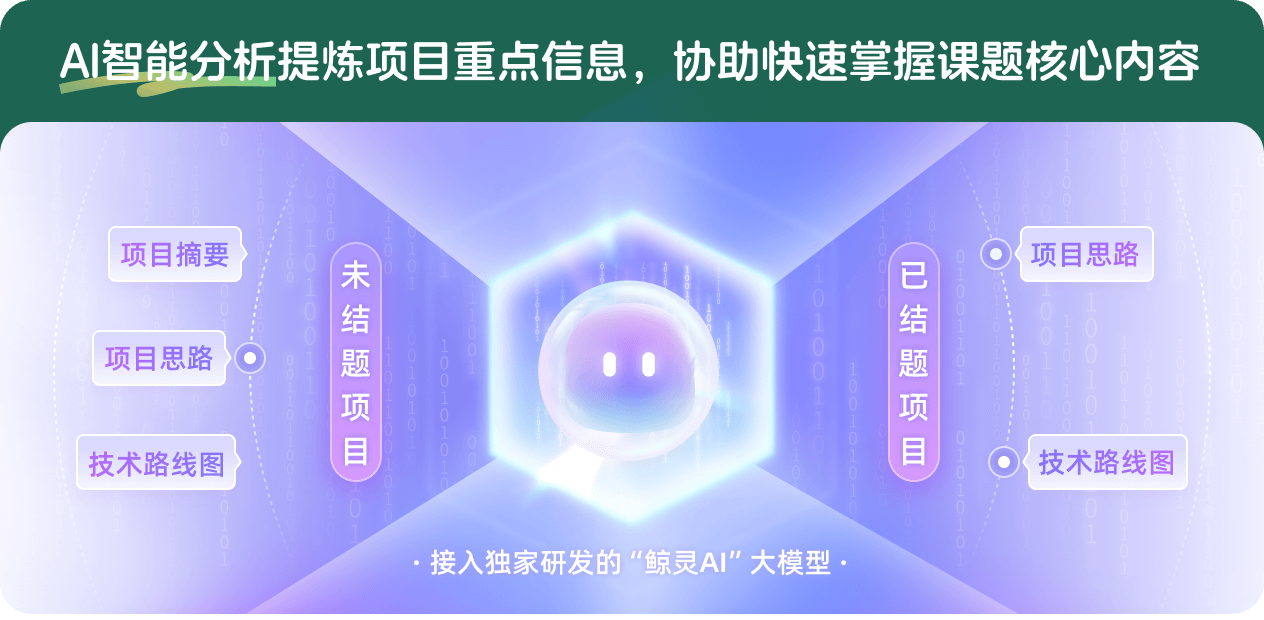
查看分析示例
此项目为已结题,我已根据课题信息分析并撰写以下内容,帮您拓宽课题思路:
AI项目摘要
AI项目思路
AI技术路线图
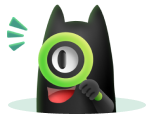
请为本次AI项目解读的内容对您的实用性打分
非常不实用
非常实用
1
2
3
4
5
6
7
8
9
10
您认为此功能如何分析更能满足您的需求,请填写您的反馈:
彭鑫的其他基金
化工园区污水系统负荷优化与运行工况评价方法研究
- 批准号:
- 批准年份:2021
- 资助金额:57 万元
- 项目类别:面上项目
相似国自然基金
{{ item.name }}
- 批准号:{{ item.ratify_no }}
- 批准年份:{{ item.approval_year }}
- 资助金额:{{ item.support_num }}
- 项目类别:{{ item.project_type }}
相似海外基金
{{
item.name }}
{{ item.translate_name }}
- 批准号:{{ item.ratify_no }}
- 财政年份:{{ item.approval_year }}
- 资助金额:{{ item.support_num }}
- 项目类别:{{ item.project_type }}