高光谱遥感图像超图迁移学习方法研究
项目介绍
AI项目解读
基本信息
- 批准号:61801336
- 项目类别:青年科学基金项目
- 资助金额:28.0万
- 负责人:
- 依托单位:
- 学科分类:F0113.信息获取与处理
- 结题年份:2021
- 批准年份:2018
- 项目状态:已结题
- 起止时间:2019-01-01 至2021-12-31
- 项目参与者:王增茂; 刘蓉; 李雪; 朱其奎; 徐永浩; 付川; 肖攀; 王勇; 程茜;
- 关键词:
项目摘要
How to effectively represent the intrinsic structure of sample distribution in feature space and achieve the feature transfer of samples with different distributions are key problems to enhance the universality of the feature learning model of hyperspectral remote sensing image (HSI). To make full use of the merits of hypergraph theory that can effectively representing the intrinsic structure relationship and transfer learning that can achieve the feature learning of samples with different distributions, this project will propose the research ideas of “adaptive hypergraph construction – graph and hypergraph collaborative representation – feature transfer learning model” to develop the efficient and robust feature learning for HSI. The main research contents include: (1) constructing adaptive hypergraph learning model to reasonably reveal the intrinsic structure relationship of HSI; (2) constructing graph and hypergraph collaborative learning model to comprehensively mining the intrinsic properties of HSI; (3) constructing hypergraph transfer learning model to achieve the feature representation of HSI with different distributions and enhance the universality of feature learning algorithm. The achievements of this research will be beneficial to improve the feature representation of HSI and can provide a theoretical foundation and implementing method in the fields of land cover classification, urban planning, precision agriculture, etc. This research has an important scientific significance and high application value.
如何有效表达特征空间中样本分布的内在结构,实现不同分布样本间的特征迁移,是增强高光谱遥感图像特征学习模型普适性的关键问题。充分利用超图理论能有效表达数据内在结构和迁移学习能实现不同分布样本特征学习的优势,本项目拟提出“自适应超图构建-图和超图协同表达-特征迁移学习模型”的研究思路,发展高效、稳健的高光谱遥感图像特征学习算法。主要研究内容包括:(1)构建自适应超图学习模型,合理揭示高光谱遥感图像的内在结构关系;(2)建立图和超图协同学习模型,全面挖掘高光谱遥感图像的内在特性;(3)构建超图迁移学习模型,实现不同分布的高光谱遥感图像特征表达,增强特征学习算法的普适性。本研究成果有望提升高光谱遥感图像的特征表达能力,可为地物分类、城市规划、精细农业等领域的研究提供理论依据和具体方法,具有较大的科学意义和实用价值。
结项摘要
高光谱图像含有丰富的地物光谱信息,可实现地物的精细化分类。由于高光谱图像光谱波段间间隔狭小、波段数多,使其存在大量的冗余信息,进而干扰地物分类精度。图学习能有效揭示数据的内在复杂结构,挖掘数据的内在鉴别特征,因而本项目围绕高光谱图像图学习方法展开研究,解决高光谱数据内在复杂特征提取问题,主要研究内容包括:(1) 由于高光谱图像复杂的成像方式和环境,导致高光谱数据间存在复杂的内在高阶关系,因而本项目利用稀疏约束的自适应性,提出自适应超图学习方法用于揭示高光谱图像复杂的内在关系,提升特征表达性能;(2) 由于高光谱图像拥有成百个通道,内在结构非常复杂,单一结构难以反映高光谱图像的内在结构,因而本项目考虑了高光谱图像多种结构信息,建立了图和超图协同学习方法,全面表达高光谱图像的内在结构特征,提升分类效果;(3) 由于高光谱图像的成像范围广,难以获取新区域大量样本的类别信息,因而本项目充分利用已知区域的类别信息,构建跨区域的超图学习模型,实现不同区域高光谱图像之间的特征迁移,拓展高光谱图像的应用范围。本项目的研究成果增强了高光谱图像的特征表达力,提升了地物分类精度,进一步促进了高光谱图像在城市规划、精细农业、军事侦察等领域的应用。目前,在该项目的资助下,项目组已发表科研论文18篇,其中SCI检索论文16篇,国际会议论文2篇,培养博士、硕士研究生6名。
项目成果
期刊论文数量(16)
专著数量(0)
科研奖励数量(0)
会议论文数量(2)
专利数量(0)
Hyperspectral image compression based on simultaneous sparse representation and general-pixels
基于同时稀疏表示和通用像素的高光谱图像压缩
- DOI:10.1016/j.patrec.2018.09.013
- 发表时间:2018-12
- 期刊:Pattern Recognition Letters
- 影响因子:5.1
- 作者:Fu Chuan;Yi Yaohua;Luo Fulin
- 通讯作者:Luo Fulin
Learning Structurally Incoherent Background and Target Dictionaries for Hyperspectral Target Detection
学习用于高光谱目标检测的结构不相干背景和目标字典
- DOI:10.1109/jstars.2020.3002549
- 发表时间:2020
- 期刊:IEEE Journal of Selected Topics in Applied Earth Observations and Remote Sensing
- 影响因子:5.5
- 作者:Guo Tan;Luo Fulin;Zhang Lei;Zhang Bob;Tan Xiaoheng;Zhou Xiaocheng
- 通讯作者:Zhou Xiaocheng
Review on graph learning for dimensionality reduction of hyperspectral image
高光谱图像降维的图学习综述
- DOI:10.1080/10095020.2020.1720529
- 发表时间:2020-01
- 期刊:Geo-spatial Information Science
- 影响因子:6
- 作者:Zhang Liangpei;Luo Fulin
- 通讯作者:Luo Fulin
Sparse-Adaptive Hypergraph Discriminant Analysis for Hyperspectral Image Classification
高光谱图像分类的稀疏自适应超图判别分析
- DOI:10.1109/lgrs.2019.2936652
- 发表时间:2020-06-01
- 期刊:IEEE GEOSCIENCE AND REMOTE SENSING LETTERS
- 影响因子:4.8
- 作者:Luo, Fulin;Zhang, Liangpei;Yin, Tailang
- 通讯作者:Yin, Tailang
A High-Quality Photon-Counting CT Technique Based on Weight Adaptive Total-Variation and Image-Spectral Tensor Factorization for Small Animals Imaging
基于权重自适应全变分和图像谱张量分解的高质量光子计数CT技术用于小动物成像
- DOI:10.1109/tim.2020.3026804
- 发表时间:2021-01-01
- 期刊:IEEE TRANSACTIONS ON INSTRUMENTATION AND MEASUREMENT
- 影响因子:5.6
- 作者:Wu, Weiwen;Hu, Dianlin;Luo, Fulin
- 通讯作者:Luo, Fulin
数据更新时间:{{ journalArticles.updateTime }}
{{
item.title }}
{{ item.translation_title }}
- DOI:{{ item.doi || "--"}}
- 发表时间:{{ item.publish_year || "--" }}
- 期刊:{{ item.journal_name }}
- 影响因子:{{ item.factor || "--"}}
- 作者:{{ item.authors }}
- 通讯作者:{{ item.author }}
数据更新时间:{{ journalArticles.updateTime }}
{{ item.title }}
- 作者:{{ item.authors }}
数据更新时间:{{ monograph.updateTime }}
{{ item.title }}
- 作者:{{ item.authors }}
数据更新时间:{{ sciAawards.updateTime }}
{{ item.title }}
- 作者:{{ item.authors }}
数据更新时间:{{ conferencePapers.updateTime }}
{{ item.title }}
- 作者:{{ item.authors }}
数据更新时间:{{ patent.updateTime }}
其他文献
基于监督稀疏流形嵌入的高光谱遥感影像分类
- DOI:--
- 发表时间:2015
- 期刊:光子学报
- 影响因子:--
- 作者:黄鸿;杨娅琼;罗甫林;冯海亮
- 通讯作者:冯海亮
融合加权均值滤波与流形重构保持嵌入的高光谱影像分类
- DOI:--
- 发表时间:2016
- 期刊:光子学报
- 影响因子:--
- 作者:黄鸿;郑新磊;罗甫林
- 通讯作者:罗甫林
高光谱图像稀疏流形学习方法研究
- DOI:--
- 发表时间:2017
- 期刊:测绘学报
- 影响因子:--
- 作者:罗甫林
- 通讯作者:罗甫林
应用相关近邻局部线性嵌入算法的高光谱遥感影像分类
- DOI:--
- 发表时间:2014
- 期刊:光学精密工程
- 影响因子:--
- 作者:刘嘉敏;罗甫林;黄鸿;刘亦哲
- 通讯作者:刘亦哲
融合夹角度量的局部线性嵌入算法
- DOI:--
- 发表时间:2013
- 期刊:光电工程
- 影响因子:--
- 作者:刘嘉敏;罗甫林;黄鸿;杨璧泽
- 通讯作者:杨璧泽
其他文献
{{
item.title }}
{{ item.translation_title }}
- DOI:{{ item.doi || "--" }}
- 发表时间:{{ item.publish_year || "--"}}
- 期刊:{{ item.journal_name }}
- 影响因子:{{ item.factor || "--" }}
- 作者:{{ item.authors }}
- 通讯作者:{{ item.author }}
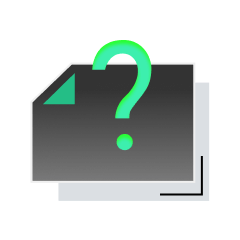
内容获取失败,请点击重试
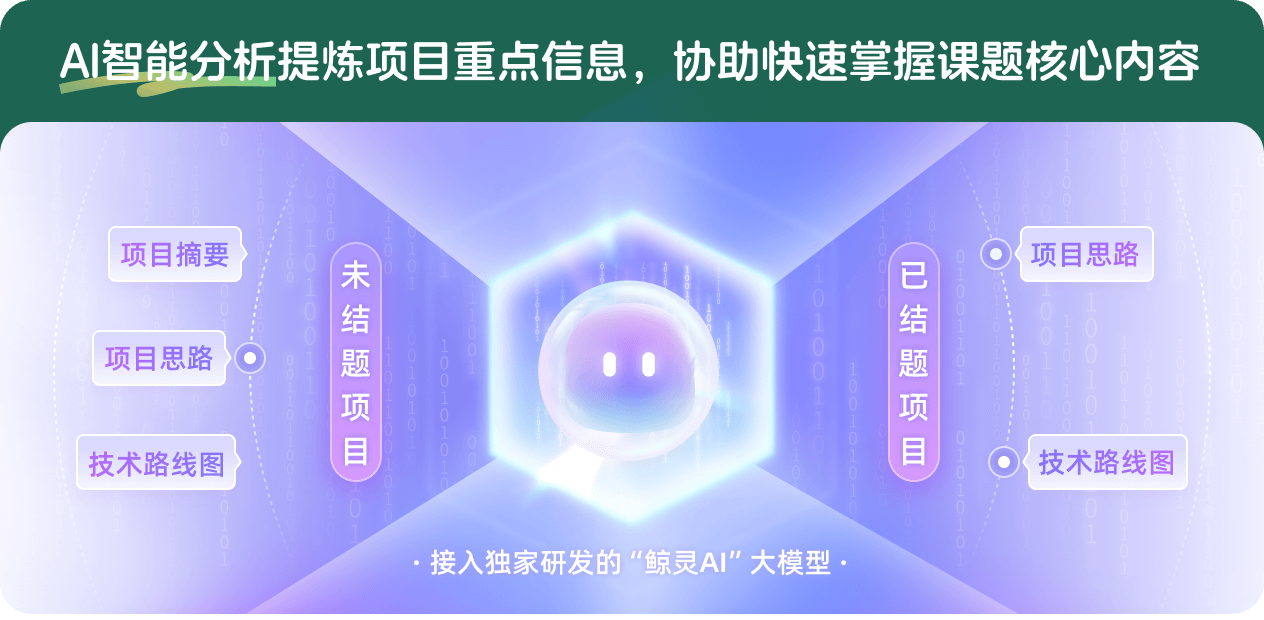
查看分析示例
此项目为已结题,我已根据课题信息分析并撰写以下内容,帮您拓宽课题思路:
AI项目摘要
AI项目思路
AI技术路线图
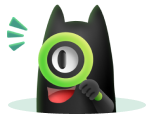
请为本次AI项目解读的内容对您的实用性打分
非常不实用
非常实用
1
2
3
4
5
6
7
8
9
10
您认为此功能如何分析更能满足您的需求,请填写您的反馈:
罗甫林的其他基金
多视图超图卷积网络的高光谱图像无监督学习方法研究
- 批准号:
- 批准年份:2020
- 资助金额:63 万元
- 项目类别:面上项目
相似国自然基金
{{ item.name }}
- 批准号:{{ item.ratify_no }}
- 批准年份:{{ item.approval_year }}
- 资助金额:{{ item.support_num }}
- 项目类别:{{ item.project_type }}
相似海外基金
{{
item.name }}
{{ item.translate_name }}
- 批准号:{{ item.ratify_no }}
- 财政年份:{{ item.approval_year }}
- 资助金额:{{ item.support_num }}
- 项目类别:{{ item.project_type }}