多源图像目标分析关键问题的研究
项目介绍
AI项目解读
基本信息
- 批准号:61370123
- 项目类别:面上项目
- 资助金额:75.0万
- 负责人:
- 依托单位:
- 学科分类:F0605.模式识别与数据挖掘
- 结题年份:2017
- 批准年份:2013
- 项目状态:已结题
- 起止时间:2014-01-01 至2017-12-31
- 项目参与者:毛晓艳; 夏榆滨; 巢文涵; 张亮; 郭周晓; 张慧港; 王俊秀; 杨海川; 留春钿;
- 关键词:
项目摘要
With the development of optics, electronic engineering, and computer science, digital images generated by various imaging devices have become available for many applications. On one hand, such multi-source images provide comprehensive information on scenes and targets under study. On the other hand, they also bring new challenges and difficulties to target analysis. This project will address some key problems in target analysis with multi-source image, including multi-source feature extraction and fusion, high-level structural description of target objects, construction and updating of target model, and efficient multi-source data analysis, et al. The goal is to develop novel methods for target analysis and recognition in multi-source images. This project will commence from proposing a feature selection framework based on iterative matching for the features extracted from multi-source images. Feature selection and fusion are then explored and combined to generate better discriminative feature for further analysis. Next, deep-learning and graph generative model will be used to describe structural information from multi-source image target. Hypergraph will be adopted to describe the complex relationship caused by multi-source image representation, which will facilitate the development of a novel hierarchical hashing method for efficient information processing. Finally, we will build an object model with incremental and transfer learning capabilities. This project will provide new insight into target analysis and recognition in multi-source images, and lead to solutions with improved accuracy and efficiency. Applications of this research include ground-based multispectral target analysis, multi-source remote sensing image analysis, and combined infrared and visible image analysis, et al.
多源图像在提供丰富目标信息的同时也为目标分析提出了新的挑战。本项目针对多源图像目标分析中的几个关键问题:多源图像特征融合、多源图像目标高层结构信息的提取与表示、多源目标快速分析以及目标模型的建立与更新等,提出解决多源图像目标分析与识别过程中上述问题的新方法。本项目拟首先提出一种基于迭代匹配的特征筛选机制将特征筛选与特征融合相结合进行。接着利用深度学习方法和图生成模型提取与分析多源图像目标结构信息。并利用超图刻画多源数据复杂关系,提出基于超图的层次化哈希方法高效处理多源数据。最后建立具有增量和迁移能力的目标模型,根据多源图像特点对目标进行分析。本项目的研究可以为解决多源图像目标分析的相关难点提供新思路。研究成果可以提高多源图像目标分析的准确率与效率,可应用于可见光与多光谱目标分析、多源遥感图像分析以及红外与可见光的目标分析等。
结项摘要
本项目针对多源图像目标分析中的几个关键问题:多源图像特征融合、多源图像目标高层特征提取、图像目标快速分析以及目标模型的建立与更新等开展了研究并提出了基于图结构的异源图像匹配方法、基于分类器机制的特征融合与筛选方法,解决了多源图像特征融合的问题;提出了利用图理论提取和表示多源图像目标高层结构信息的方法,并根据提取的目标表示建立了具备自学习能力和增量学习能力的目标模型;针对提取的高维多源特征,提出了快速处理高维信息的结构化哈希、在线哈希以及高维哈希方法,提升了目标分析与处理的效率。本项目所取得的成果解决了多源目标分析、海量图像分析、多源融合等领域的关键问题并做出了一定的理论贡献。. 受本项目资助,项目组成员在国际高水平期刊上如:IEEE TIP,IEEE TGRS, Pattern Recognition等上发表SCI论文16篇,在国际高水平会议IEEE CVPR, ECML/PKDD, ACCV, ICIP等发表论文14篇,共计发表论文30篇,发表专著1部获批专利2项,理论成果达到预期要求。培养硕士研究生10人,毕业6人,培养博士研究生4人,5人次获得“国家奖学金”。项目组成员赴国外参加国际会议和交流访问8人次,邀请国外专家来华访问7人次。项目负责人百晓教授于2015年被聘为博士生导师并于同年晋升为教授,2015年获评为中国电子学会“优秀科技工作者”,2016年被中国科协评为“全国优秀科技工作者”,从2017年开始担任国际模式识别领域著名期刊Pattern Recognition副主编。综上所述,本项目达到了预定目标,研究的成果可应用于多源图像目标分析、海量数据分析、多源融合等方面。
项目成果
期刊论文数量(18)
专著数量(1)
科研奖励数量(0)
会议论文数量(14)
专利数量(2)
VHR Object Detection Based on Structural Feature Extraction and Query Expansion
基于结构特征提取和查询扩展的VHR目标检测
- DOI:10.1002/capr.12185
- 发表时间:2014
- 期刊:Ieee Transactions ON Geoscience and Remote Sensing
- 影响因子:8.2
- 作者:Bai Xiao;Zhang Huigang;Zhou Jun
- 通讯作者:Zhou Jun
Quantum kernels for unattributed graphs using discrete-time quantum walks
使用离散时间量子行走的无属性图的量子核
- DOI:10.1016/j.patrec.2016.08.019
- 发表时间:2017
- 期刊:Pattern Recognition Letters
- 影响因子:5.1
- 作者:Bai Lu;Rossi Luca;Cui Lixin;Zhang Zhihong;Ren Peng;Bai Xiao;Hancock Edwin
- 通讯作者:Hancock Edwin
Band Weighting via Maximizing Interclass Distance for Hyperspectral Image Classification
通过最大化高光谱图像分类的类间距离进行波段加权
- DOI:10.1093/mnras/stad3725
- 发表时间:2016
- 期刊:Ieee Geoscience and Remote Sensing Letters
- 影响因子:--
- 作者:Yan Cheng;Bai Xiao;Ren Peng;Bai Lu;Tang Wenzhong;Zhou Jun
- 通讯作者:Zhou Jun
Differential weights-based band selection for hyperspectral image classification
用于高光谱图像分类的基于差分权重的波段选择
- DOI:10.1142/s0219691317500655
- 发表时间:2017
- 期刊:International Journal of Wavelets, Multiresolution and Information Processing
- 影响因子:--
- 作者:Liu Yun;Chen Wang;Xiao Bai;Yang Wang;Jun Zhou
- 通讯作者:Jun Zhou
An incremental structured part model for object recognition
用于对象识别的增量结构化零件模型
- DOI:10.1016/j.neucom.2014.12.004
- 发表时间:2015
- 期刊:Neurocomputing
- 影响因子:6
- 作者:Bai Xiao;Ren Peng;Zhang Huigang;Zhou Jun
- 通讯作者:Zhou Jun
数据更新时间:{{ journalArticles.updateTime }}
{{
item.title }}
{{ item.translation_title }}
- DOI:{{ item.doi || "--"}}
- 发表时间:{{ item.publish_year || "--" }}
- 期刊:{{ item.journal_name }}
- 影响因子:{{ item.factor || "--"}}
- 作者:{{ item.authors }}
- 通讯作者:{{ item.author }}
数据更新时间:{{ journalArticles.updateTime }}
{{ item.title }}
- 作者:{{ item.authors }}
数据更新时间:{{ monograph.updateTime }}
{{ item.title }}
- 作者:{{ item.authors }}
数据更新时间:{{ sciAawards.updateTime }}
{{ item.title }}
- 作者:{{ item.authors }}
数据更新时间:{{ conferencePapers.updateTime }}
{{ item.title }}
- 作者:{{ item.authors }}
数据更新时间:{{ patent.updateTime }}
其他文献
Object Detection via Structural Feature Selection and Shape Model
通过结构特征选择和形状模型进行物体检测
- DOI:--
- 发表时间:2013
- 期刊:IEEE Transactions on Image Processing
- 影响因子:10.6
- 作者:百晓
- 通讯作者:百晓
Asymmetric propagation based batch mode active learning for image retrieval
基于非对称传播的图像检索批量模式主动学习
- DOI:--
- 发表时间:2012
- 期刊:Signal Processing
- 影响因子:4.4
- 作者:百晓
- 通讯作者:百晓
其他文献
{{
item.title }}
{{ item.translation_title }}
- DOI:{{ item.doi || "--" }}
- 发表时间:{{ item.publish_year || "--"}}
- 期刊:{{ item.journal_name }}
- 影响因子:{{ item.factor || "--" }}
- 作者:{{ item.authors }}
- 通讯作者:{{ item.author }}
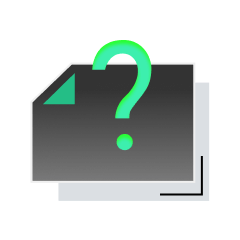
内容获取失败,请点击重试
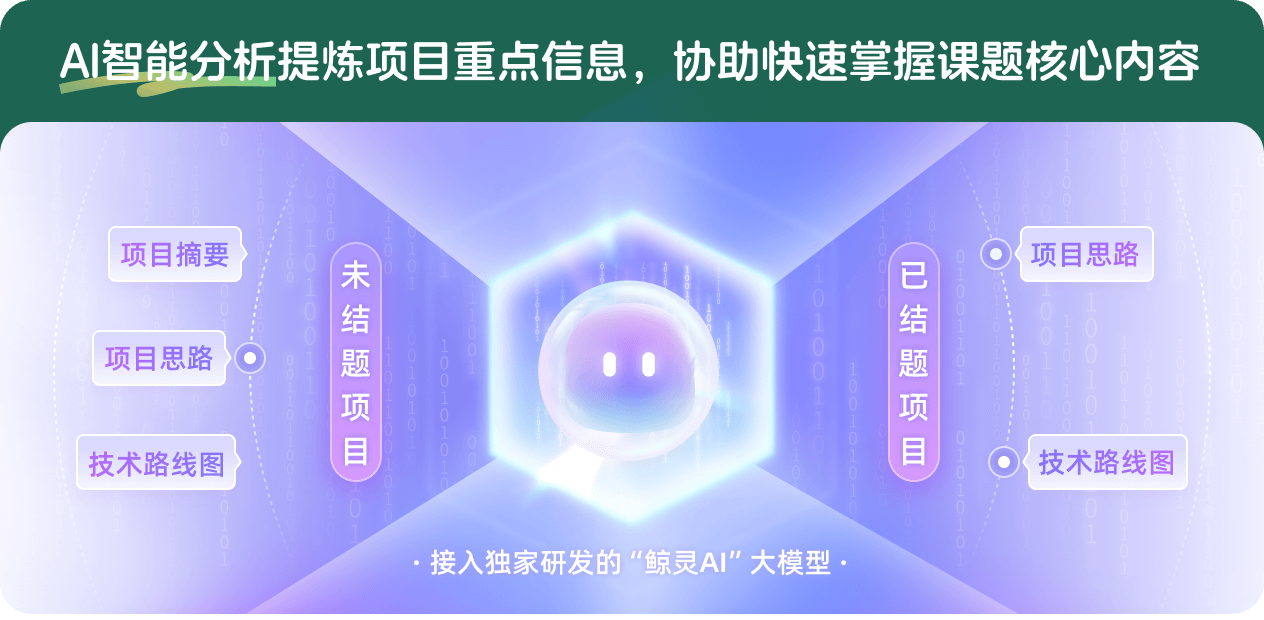
查看分析示例
此项目为已结题,我已根据课题信息分析并撰写以下内容,帮您拓宽课题思路:
AI项目摘要
AI项目思路
AI技术路线图
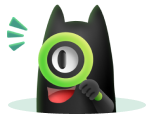
请为本次AI项目解读的内容对您的实用性打分
非常不实用
非常实用
1
2
3
4
5
6
7
8
9
10
您认为此功能如何分析更能满足您的需求,请填写您的反馈:
百晓的其他基金
面向开放世界的可解释目标分析理论和方法研究
- 批准号:
- 批准年份:2022
- 资助金额:55 万元
- 项目类别:面上项目
基于高光谱的近距离场景分析的研究
- 批准号:61772057
- 批准年份:2017
- 资助金额:65.0 万元
- 项目类别:面上项目
基于图的增量半监督图像目标分类与识别
- 批准号:61105002
- 批准年份:2011
- 资助金额:23.0 万元
- 项目类别:青年科学基金项目
相似国自然基金
{{ item.name }}
- 批准号:{{ item.ratify_no }}
- 批准年份:{{ item.approval_year }}
- 资助金额:{{ item.support_num }}
- 项目类别:{{ item.project_type }}
相似海外基金
{{
item.name }}
{{ item.translate_name }}
- 批准号:{{ item.ratify_no }}
- 财政年份:{{ item.approval_year }}
- 资助金额:{{ item.support_num }}
- 项目类别:{{ item.project_type }}