连续状态空间模型未知下的在线强化学习方法
项目介绍
AI项目解读
基本信息
- 批准号:61806004
- 项目类别:青年科学基金项目
- 资助金额:27.0万
- 负责人:
- 依托单位:
- 学科分类:F0603.机器学习
- 结题年份:2021
- 批准年份:2018
- 项目状态:已结题
- 起止时间:2019-01-01 至2021-12-31
- 项目参与者:陈业斌; 程泽凯; 邵光梅; 郭云婷; 谢宁宇; 张震;
- 关键词:
项目摘要
With the rapid development of control technology, the controlled variables become continuous and complicated. Reinforcement learning can effectively solve the optimal control problem, which makes it a hotspot in the field of machine learning and control. This project intends to make use of the latest research achievements of the theory of probably approximately correct, statistical learning technology, online learning technology and tensor theory, and we will do research in online reinforcement learning based on basic theory, key technologies, and typical examples for verification to meet the defects of reinforcement learning at present such as poor learning results, unclear exploration, low learning efficiency and so on. First, we study the algorithm framework that satisfies the theory of probably approximately correct to provide theoretical guidance to solve the control problem of continuous-state system. Secondly, based on least square, nuclear support tensor machine, and kd-tree, we construct online reinforcement learning technology for continuous and discrete action space, and study the adaptive learning of model parameters to realize model reasoning and generation. Finally, combined with the task of robot component flexible control in the Anhui province key laboratory of special overload robot, we verify the proposed key technology. Through the research above, exploring the integrating points for reinforcement learning and online learning is proved to have great significance to enrich and supplement the theory systems for reinforcement learning.
随着控制技术的迅速发展,被控变量变得连续复杂。强化学习能有效解决最优控制问题,成为当前机器学习和控制领域研究的热点。本项目以模型数据未知连续状态系统的最优控制问题为背景,针对目前强化学习方法存在的学习结果欠优、探索不明确、学习利用率低等缺点,拟利用概率近似正确理论、统计学习技术、在线技术、张量理论等方面的最新成果,从基础理论、关键技术、实例验证三个方面开展在线强化学习方法研究。首先,研究满足概率近似正确理论的算法框架,为形成连续状态系统控制问题的具体求解提供理论指导;其次,针对连续、离散动作空间,构建基于最小二乘、核支持张量机、kd树等技术的在线强化学习方法,并研究模型参数自适应学习,实现模型的推理与生成;最后,结合安徽省特种重载机器人实验室机器人部件柔性控制任务,验证提出的关键技术。通过该项目的研究,探索强化学习和在线学习的契合点,对强化学习理论体系的丰富与补充具有重要的意义。
结项摘要
连续状态系统的最优控制问题是强化学习领域研究的热点。针对目前强化学习方法存在的学习结果欠优、探索不明确、学习利用率低等缺点,本项目应用统计方法、在线学习、张量等技术,对连续状态-连续动作和连续状态-离散动作强化学习方法的分析、在线学习算法的设计与实现、基于“探索-利用”和稀疏学习的最小二乘策略迭代在线算法构建、基于kd树划分连续状态空间的离散动作细化、高估现象下的集成Q学习网络构建等问题进行了研究,探索了在线学习与强化学习相互作用的契合点,对强化学习技术体系的丰富与补充具有一定的意义。
项目成果
期刊论文数量(14)
专著数量(0)
科研奖励数量(0)
会议论文数量(2)
专利数量(1)
Low-Rank Approximation and Multiple Sparse Constraint Modeling for Infrared Low-Flying Fixed-Wing UAV Detection
红外低空飞行固定翼无人机检测的低秩逼近和多重稀疏约束建模
- DOI:10.1109/jstars.2021.3069032
- 发表时间:2021
- 期刊:IEEE Journal of Selected Topics in Applied Earth Observations and Remote Sensing
- 影响因子:5.5
- 作者:Wei Xue;Jiahao Qi;Guoqing Shao;Zixuan Xiao;Yu Zhang;Ping Zhong
- 通讯作者:Ping Zhong
Weighted feature-task-aware regularization learner for multitask learning
用于多任务学习的加权特征任务感知正则化学习器
- DOI:10.1007/s10044-019-00781-8
- 发表时间:2019-02
- 期刊:Pattern Analysis and Applications
- 影响因子:3.9
- 作者:Wei Xue
- 通讯作者:Wei Xue
HITS centrality based on inter-layer similarity for multilayer temporal networks
基于多层时间网络层间相似性的 HITS 中心性
- DOI:10.1016/j.neucom.2020.10.040
- 发表时间:2021-01
- 期刊:Neurocomputing
- 影响因子:6
- 作者:Laishui Lv;Kun Zhang;Dalal Bardou;Xun Li;Ting Zhang;Wei Xue
- 通讯作者:Wei Xue
Two-Objective Filtering for Takagi–Sugeno Fuzzy Hopfield Neural Networks with Time-Variant Delay
具有时变延迟的 Takagi–Sugeno 模糊 Hopfield 神经网络的双目标滤波
- DOI:10.1007/s11063-021-10580-0
- 发表时间:2021-07
- 期刊:Neural Processing Letters
- 影响因子:3.1
- 作者:Qi Hu;Lezhu Chen;Jianping Zhou;Zhen Wang
- 通讯作者:Zhen Wang
Eigenvector-based centralities for multilayer temporal networks under the framework of tensor computation
张量计算框架下基于特征向量的多层时序网络中心性
- DOI:10.1016/j.eswa.2021.115471
- 发表时间:2021-12
- 期刊:Expert Systems With Applications
- 影响因子:8.5
- 作者:Laishui Lv;Kun Zhang;Ting Zhang;Xun Li;Qi Sun;Lilinqing Zhang;Wei Xue
- 通讯作者:Wei Xue
数据更新时间:{{ journalArticles.updateTime }}
{{
item.title }}
{{ item.translation_title }}
- DOI:{{ item.doi || "--"}}
- 发表时间:{{ item.publish_year || "--" }}
- 期刊:{{ item.journal_name }}
- 影响因子:{{ item.factor || "--"}}
- 作者:{{ item.authors }}
- 通讯作者:{{ item.author }}
数据更新时间:{{ journalArticles.updateTime }}
{{ item.title }}
- 作者:{{ item.authors }}
数据更新时间:{{ monograph.updateTime }}
{{ item.title }}
- 作者:{{ item.authors }}
数据更新时间:{{ sciAawards.updateTime }}
{{ item.title }}
- 作者:{{ item.authors }}
数据更新时间:{{ conferencePapers.updateTime }}
{{ item.title }}
- 作者:{{ item.authors }}
数据更新时间:{{ patent.updateTime }}
其他文献
振动式蓝莓采摘机槽型凸轮传动装置的设计与分析
- DOI:10.13759/j.cnki.dlxb.2017.10.019
- 发表时间:2017
- 期刊:东北林业大学学报
- 影响因子:--
- 作者:王景巍;王海滨;李志鹏;薛伟;曹国昕
- 通讯作者:曹国昕
含苯并三嗪酮的1,4-戊二烯-3-酮衍生物的合成及抑菌活性
- DOI:10.7503/cjcu20170785
- 发表时间:2018-04
- 期刊:高等学校化学学报
- 影响因子:--
- 作者:张菊平;李普;王一会;张橙;陈丽娟;汤旭;贺鸣;薛伟
- 通讯作者:薛伟
非均相催化剂催化5-羟甲基糠醛氢解制备2,5-二甲基呋喃研究进展
- DOI:10.16085/j.issn.1000-6613.2020-0710
- 发表时间:2021-02
- 期刊:化工进展
- 影响因子:--
- 作者:王彤;安华良;李芳;薛伟;王延吉
- 通讯作者:王延吉
由苯一步制备环己醇的催化过程
- DOI:--
- 发表时间:--
- 期刊:石油学报(石油加工)
- 影响因子:--
- 作者:魏珺芳;王冬冬;王延吉;刘媛;薛伟
- 通讯作者:薛伟
热休克蛋白与植物病毒组分的互作机制研究进展
- DOI:--
- 发表时间:2006
- 期刊:应用与环境生物学报
- 影响因子:--
- 作者:王勇;赵晓珍;包兴涛;任亚峰;李冬雪;薛伟;胡德禹;陈卓
- 通讯作者:陈卓
其他文献
{{
item.title }}
{{ item.translation_title }}
- DOI:{{ item.doi || "--" }}
- 发表时间:{{ item.publish_year || "--"}}
- 期刊:{{ item.journal_name }}
- 影响因子:{{ item.factor || "--" }}
- 作者:{{ item.authors }}
- 通讯作者:{{ item.author }}
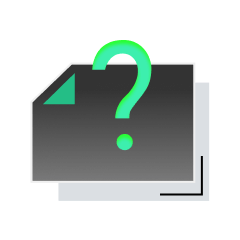
内容获取失败,请点击重试
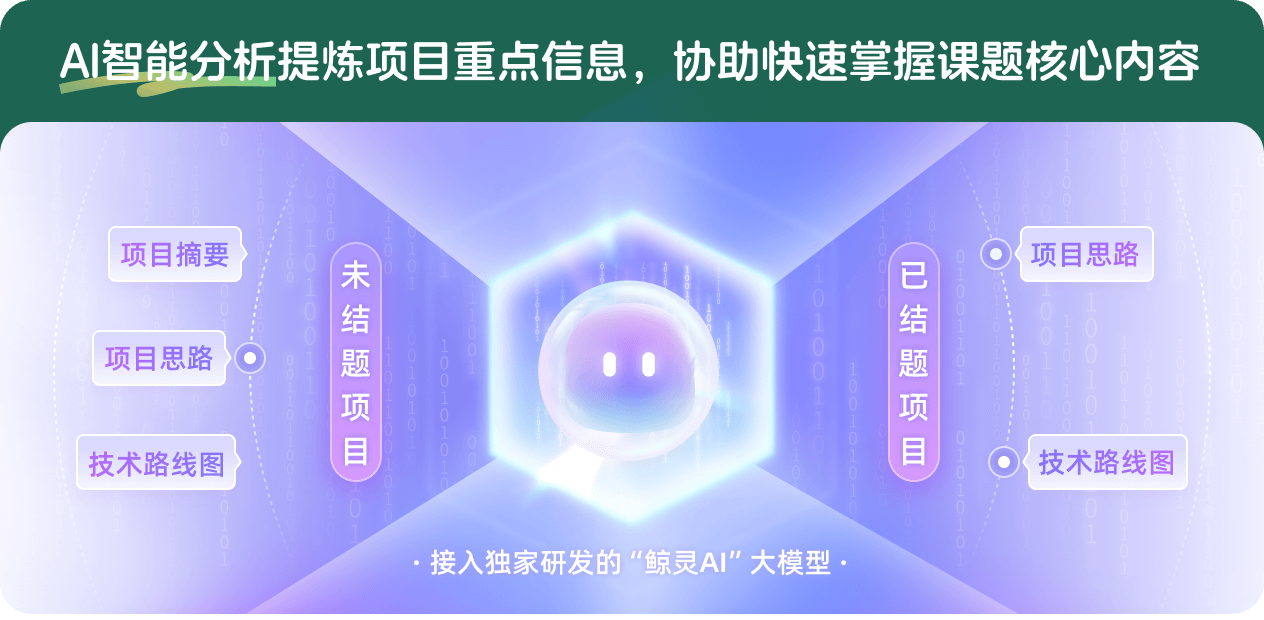
查看分析示例
此项目为已结题,我已根据课题信息分析并撰写以下内容,帮您拓宽课题思路:
AI项目摘要
AI项目思路
AI技术路线图
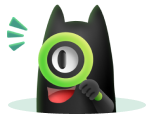
请为本次AI项目解读的内容对您的实用性打分
非常不实用
非常实用
1
2
3
4
5
6
7
8
9
10
您认为此功能如何分析更能满足您的需求,请填写您的反馈:
相似国自然基金
{{ item.name }}
- 批准号:{{ item.ratify_no }}
- 批准年份:{{ item.approval_year }}
- 资助金额:{{ item.support_num }}
- 项目类别:{{ item.project_type }}
相似海外基金
{{
item.name }}
{{ item.translate_name }}
- 批准号:{{ item.ratify_no }}
- 财政年份:{{ item.approval_year }}
- 资助金额:{{ item.support_num }}
- 项目类别:{{ item.project_type }}