基于高维空间分析与机器学习观点的鲆鲽类养殖行为学监控及智能分析
项目介绍
AI项目解读
基本信息
- 批准号:31202036
- 项目类别:青年科学基金项目
- 资助金额:25.0万
- 负责人:
- 依托单位:
- 学科分类:C1909.养殖与渔业工程学
- 结题年份:2015
- 批准年份:2012
- 项目状态:已结题
- 起止时间:2013-01-01 至2015-12-31
- 项目参与者:何波; 于佳; 崔国霖; 杨华; 孙勇; 王扬帆; 高翔; 徐萌; 石潇龙;
- 关键词:
项目摘要
Aquaculture is one of the key marine industries in the 'Blue Economy', an effective culturing video surveillance system during the whole process is of great significance in science and practice to develop aquaculture towards an intensive, technological, information-oriented direction..Flatfish farming plays the main role in the Aquaculture in China. In the project, taking the Scophthalmus maximus as an example, the underwater video analysis as the key technique, we concentrate on the fish ethological surveillance and intellgent analysis. During a certain culturing environment and in a certain stage, high-dimensional space analysis, compressed sensing, homomorphic filtering in the wavelet domain,etc. will be adopted tohelp with better description and representation for the surveillance. On the other hand, the deep belief nets, geometrical topology manifolds, extreme learning machine will be respectively employed to learn and integrate the ethological characteristics, explore the individual features, behaviours and group activities, mine the features and rules during swimming, feeding, or the state in stress. After accumulation, the general behaviour information database for Scophthalmus maximus and one intelligent analysis system for ethology will be formed. .This research takes the viewpoint of machine learning to aid the research in fish ethology, will provide technical supports for the healthy and sustainable development of the Flatfish farming, and at the same time, promote the research in the high-dimensional space analysis for the moving object images in multiple angles and various shapes.
水产养殖是蓝色经济的支柱产业,养殖全过程的有效监控对指导水产养殖向集约化、科技化、信息化方向发展具有科学和现实意义。.鲆鲽类养殖在我国水产养殖业中占有举足轻重的地位。本项目以大菱鲆作为研究对象,以水下视频分析技术为核心,研究养殖鱼类监控及行为学智能化分析问题。针对不同发育阶段、不同养殖环境,以高维空间分析、压缩感知原理、小波域同态滤波等发展大菱鲆监控的表示方法,以深度信念网、几何拓扑流形、极限学习机等机器学习方法分析其行为学特性,进而探索对大菱鲆个体体征、行为及群体活动的分析策略,挖掘大菱鲆游泳行为、摄食行为或应激状态的特征及规律,逐步积累,形成大菱鲆养殖行为综合信息数据库,构建大菱鲆养殖行为学智能分析系统。.该研究从机器学习的角度辅助大菱鲆养殖的行为学研究,可以为鲆鲽类养殖健康可持续发展提供技术支持,同时也将促进多视点及形态变化运动目标图像的高维空间分析机制研究。
结项摘要
海水养殖是我国蓝色经济的支柱产业。本项目针对水产品高效健康养殖需求,以高维空间理论与机器学习观点为指导,重点研究鲆鲽类养殖行为学监控及智能分析问题,其关键科学问题集中于:(1)视觉信息变化影响因素规律分析及高维空间数据的优化表示,(2)模式变化目标的全局多角度特性及典型行为学特征的提取与识别。.针对以大菱鲆为代表的鱼类视觉监控问题,本项目开展了基于单目视觉和双目视觉、静态和动态拍摄系统、多摄像头协同等水下立体视觉监控系统构建实验工作,并初步尝试以水下机器人为载体,为鲆鲽类养殖行为学观测拓展方向。基于Jaffe-McGlamery经典模型研究水下光学图像退化模型,估测水体点扩展函数和光传递函数的简化表示;针对水下成像色彩偏差、光照不均、细节模糊等问题,尝试小波域同态滤波、局部邻域中值点追踪和实时快速加权中值滤波实现水下图像增强和平滑去噪;基于压缩感知理论、词袋模型、分层独立成分分析学习框架、局部切空间排列流形学习等方法开展水下视觉监控海量高维空间数据的优化表示、采样与选择研究;以Marr视觉理论为指导开展养殖场景深度估计和三维重建研究。.针对大菱鲆行为学智能分析问题,本项目以超像素概念、黑森矩阵、水平集、Mumford-Shah模型、偏微分方程探索水下目标分割与检测方法;综合利用大菱鲆运动状态向量、色彩分布、鱼体表面认知模型等多重线索,实时追踪水下目标轨迹;以受限玻尔兹曼机、深度信念网、偶极子核函数、极限学习机等机器学习方法开展水下运动目标视觉认知与姿态估计研究;通过在线学习逐步拟合大菱鲆生长体征关系模型;从大菱鲆行为动作分解入手,探索一定视角范围内个体运动的规律和共性,提取标准动作呈现的行为学特征集合;初步尝试人工鱼群方法分析大菱鲆群体行为,估计鱼群分布密集程度;引入占有体积概念等研究有关大菱鲆运动的多摄像头协同追踪问题。.本项目积累建立大菱鲆养殖行为学特征综合信息支持平台,初步设计鱼类行为学监控V1.0软件系统,包含鱼类运动监控、生长体征设置、鱼体形态特征提取、个体和群体运动特征提取、行为学规律分析等模块,并推测可能发生的应激反应或病害感染作出报警提示。.项目执行期间共发表学术论文41篇,其中19篇被SCI收录,31篇被EI收录。项目研究成果有助于解答养殖专家或养殖户自由提出的开放性问题,为鲆鲽类养殖向科技化、信息化、数字化、智能化方向发展开展基础性工作。
项目成果
期刊论文数量(12)
专著数量(0)
科研奖励数量(0)
会议论文数量(15)
专利数量(0)
ROV-based Underwater Vision System for Intelligent Fish Ethology Research
基于ROV的水下视觉系统用于智能鱼类行为学研究
- DOI:10.5772/56800
- 发表时间:2013-09
- 期刊:International Journal of Advanced Robotic Systems
- 影响因子:2.3
- 作者:Bo He;Jia Yu;Zhenmin Bao;Yangfan Wang
- 通讯作者:Yangfan Wang
An early underwater artificial vision model in ocean investigations via independent component analysis.
通过独立成分分析建立海洋调查中的早期水下人工视觉模型
- DOI:10.3390/s130709104
- 发表时间:2013-07-16
- 期刊:Sensors (Basel, Switzerland)
- 影响因子:--
- 作者:Nian R;Liu F;He B
- 通讯作者:He B
Extreme learning machine towards dynamic model hypothesis in fish ethology research
鱼类行为学研究中动态模型假设的极限学习机
- DOI:10.1016/j.neucom.2013.03.054
- 发表时间:2014-03
- 期刊:Neurocomputing
- 影响因子:6
- 作者:Mark van Heeswijk;Qi Yu;Yoan Miche;Amaury Lendasse
- 通讯作者:Amaury Lendasse
Fast Face Recognition Via Sparse Coding and Extreme Learning Machine
通过稀疏编码和极限学习机进行快速人脸识别
- DOI:10.1007/s12559-013-9224-1
- 发表时间:2014-06-01
- 期刊:COGNITIVE COMPUTATION
- 影响因子:5.4
- 作者:He, Bo;Xu, Dongxun;Lendasse, Amaury
- 通讯作者:Lendasse, Amaury
数据更新时间:{{ journalArticles.updateTime }}
{{
item.title }}
{{ item.translation_title }}
- DOI:{{ item.doi || "--"}}
- 发表时间:{{ item.publish_year || "--" }}
- 期刊:{{ item.journal_name }}
- 影响因子:{{ item.factor || "--"}}
- 作者:{{ item.authors }}
- 通讯作者:{{ item.author }}
数据更新时间:{{ journalArticles.updateTime }}
{{ item.title }}
- 作者:{{ item.authors }}
数据更新时间:{{ monograph.updateTime }}
{{ item.title }}
- 作者:{{ item.authors }}
数据更新时间:{{ sciAawards.updateTime }}
{{ item.title }}
- 作者:{{ item.authors }}
数据更新时间:{{ conferencePapers.updateTime }}
{{ item.title }}
- 作者:{{ item.authors }}
数据更新时间:{{ patent.updateTime }}
其他文献
其他文献
{{
item.title }}
{{ item.translation_title }}
- DOI:{{ item.doi || "--" }}
- 发表时间:{{ item.publish_year || "--"}}
- 期刊:{{ item.journal_name }}
- 影响因子:{{ item.factor || "--" }}
- 作者:{{ item.authors }}
- 通讯作者:{{ item.author }}
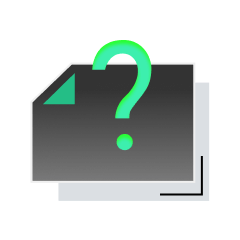
内容获取失败,请点击重试
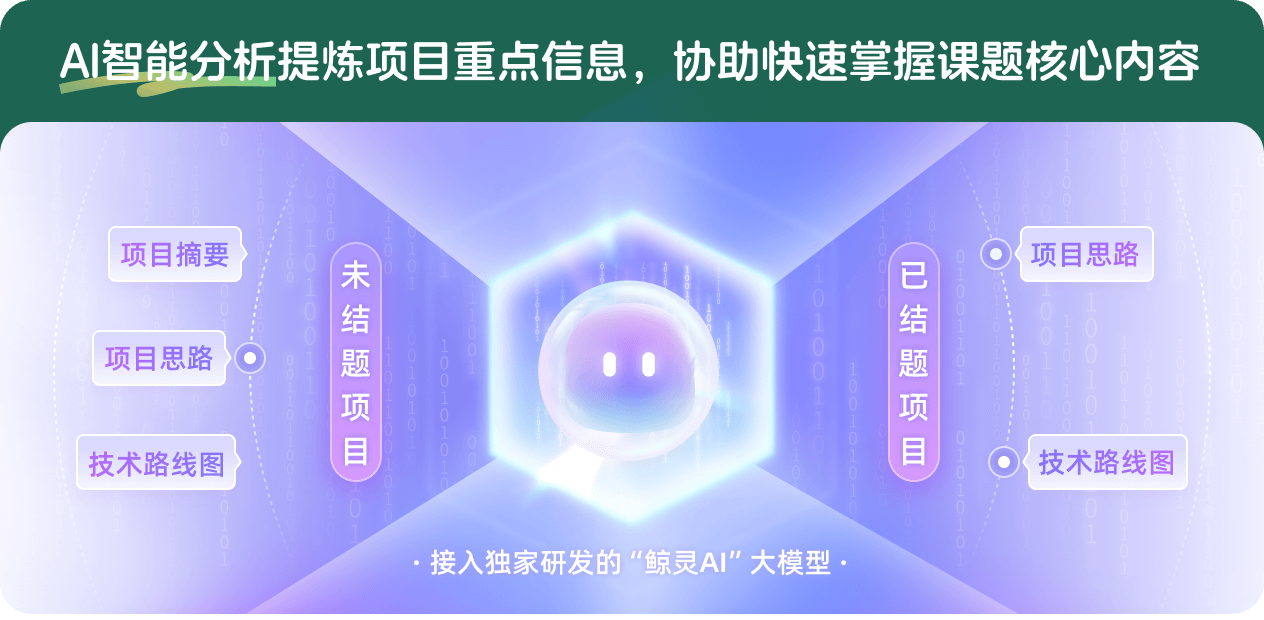
查看分析示例
此项目为已结题,我已根据课题信息分析并撰写以下内容,帮您拓宽课题思路:
AI项目摘要
AI项目思路
AI技术路线图
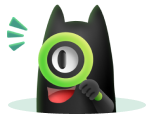
请为本次AI项目解读的内容对您的实用性打分
非常不实用
非常实用
1
2
3
4
5
6
7
8
9
10
您认为此功能如何分析更能满足您的需求,请填写您的反馈:
年睿的其他基金
南海中尺度涡AI预报及适应性观测策略研究
- 批准号:42376191
- 批准年份:2023
- 资助金额:51 万元
- 项目类别:面上项目
相似国自然基金
{{ item.name }}
- 批准号:{{ item.ratify_no }}
- 批准年份:{{ item.approval_year }}
- 资助金额:{{ item.support_num }}
- 项目类别:{{ item.project_type }}
相似海外基金
{{
item.name }}
{{ item.translate_name }}
- 批准号:{{ item.ratify_no }}
- 财政年份:{{ item.approval_year }}
- 资助金额:{{ item.support_num }}
- 项目类别:{{ item.project_type }}