复杂网络上多尺度动力学粗粒化方法的发展与应用
项目介绍
AI项目解读
基本信息
- 批准号:11475003
- 项目类别:面上项目
- 资助金额:80.0万
- 负责人:
- 依托单位:
- 学科分类:A2503.统计物理与复杂系统
- 结题年份:2018
- 批准年份:2014
- 项目状态:已结题
- 起止时间:2015-01-01 至2018-12-31
- 项目参与者:郝庆一; 李军; 陈含爽; 王林; 龚奇娟; 段兰; 张超;
- 关键词:
项目摘要
Recently, complex network has been one of the most active research topics in statistical physics and closely related disciplines. The dynamics of network and its topology usually associate with multiscale processes spanning from microscopic to mesoscopic, and to macroscopic level. It is known that brute-force simulations are quite expensive and sometimes even become impossible. While phenomenological models, such as mean-field description, may capture certain properties of the system, but often ignore microscopic details and fluctuation effects that may be important near critical points. Therefore a promising way is to develop multiscale theory and approaches, aiming at significantly reducing the degree of freedom while properly preserving the microscopic information of interest. Now, several coarse-grained methods to multiscale dynamics of complex network have been proposed, however these methods can not deal with the multiscale problem of dynamics evolution. In addition, there are also some limits in coping with the strongly correlated network or highly complex network, for instance, network of network, multiplex network and so on. In this proposal, we will apply the statistical mechanics theory to develop mesoscopic approaches on complex networks, including coarse-grained method to multiscale dynamics based on high order approximation, hybrid multiscale coarse-grained method and spatiotemporal coarse-grained method, and bridge the gap between the microscopic details and system level behaviors. We will design a series of computer programs and perform extensive numerical simulations on different network topologies to study the equilibrium and nonequilibrium phase transition, fluctuations, finite-size effects, synchronization and epidemic spreading dynamics. Our study may provide a new perspective and approach to understand the underlying mechanism between multiscale dynamics and network topology.
近年来,复杂网络已成为统计物理及其交叉学科研究的一个热点。复杂网络拓扑和动力学行为演化涉及微观、介观,乃至宏观的多个时间和空间拓扑尺度。微观模拟适用尺度相对较小,宏观唯象理论(如平均场方法)又缺乏对微观机制的理解,因此需要发展有效的多尺度理论方法。目前复杂网络上多尺度粗粒化方法研究取得了进展,但面临两个问题:一是不能有效解决动力学时间多尺度问题,二是对于关联较强或高度复杂网络,如最近报道的网络的网络、多层网络等也有局限。本项目拟运用统计力学原理,建立复杂网络上高阶近似下多尺度动力学粗粒化、杂化多尺度粗粒化和时空粗粒化理论与模拟方法,实现不同尺度理论方法的有机衔接;开发相应的计算程序;研究复杂网络上平衡和非平衡相变、临界现象、涨落、尺度效应以及同步、病毒传播的动力学规律,从而揭示介观乃至宏观现象的微观机理和调控机制,为深入理解复杂网络上多尺度动力学过程与拓扑结构之间的深层次联系提供新的启示。
结项摘要
复杂网络拓扑和动力学行为演化往往涉及微观、介观,乃至宏观的多个时间和空间拓扑尺度。微观模拟适用尺度相对较小,宏观唯象理论又缺乏对微观机制的理解,因此需要发展有效的多尺度理论方法。本项目运用统计力学原理,提出基于节点强度合并的动力学粗粒化、多尺度动力学前向流采样方法、杂化多尺度粗粒化和人体动作识别的粗粒化理论与模拟方法,并开发相应的计算程序;应用这些方法研究了复杂网络上平衡和非平衡相变、临界现象、涨落、尺度效应以及传播动力学等。项目执行期间,共发表基金标注SCI论文19篇。.1. 基于统计力学一致性,提出复杂网络上基于节点强度合并和格点合并的动力学粗粒化理论和模拟方法,高效地研究了平衡和和非平衡体系的相变和临界现象。.2. 发展复杂网络上多尺度动力学前向流采样方法,研究了复杂网络上成核相变动力学与网络拓扑、尺度的关系,以及耦合双稳体系涨落活化转变和非平衡majority-vote模型相变和临界噪声。.3. 提出复杂网络上动力学杂化多尺度粗粒化方法,对重点部分采用微观精细模拟,而对剩余部分粗粒化处理,并以平衡态Ising模型和非平衡SIS流行病传播模型为例,建立微观与粗粒化的衔接机制。该方法能很好地重复微观模型的相变和临界现象,大大加速动力学估计,还具有一般性,只要采用合适的微观模拟和粗粒化方法就可以应用在许多其他复杂网络体系。.4. 提出机器学习人体动作识别的粗粒化理论与模拟方法,即根据人体骨骼数据的统计量特征将动作按粗粒度大小进行分层,层内再根据人体运动的时空特征,进行细粒度的分类,设计自选择最佳分类器。通过公共数据集(MSR Action3D) 测试我们的方法具有较高的识别效率,并具有可行性、鲁棒性和可扩展性。.5. 提出多层网络上多尺度意见渗流模型,发现增强子网络间的相关性,可以促进具有少数信念的节点之间形成稳定的集群,并且具有少数意见的节点需要较少的初始支持便可以在多层网络中存活。
项目成果
期刊论文数量(19)
专著数量(0)
科研奖励数量(0)
会议论文数量(0)
专利数量(0)
Phase Transitions of Majority-Vote Model on Modular Networks
模块化网络上多数投票模型的相变
- DOI:--
- 发表时间:2015
- 期刊:Chinese Physics Letters
- 影响因子:3.5
- 作者:Feng Huang;Hanshuang Chen;Chuansheng Shen
- 通讯作者:Chuansheng Shen
What can AI learn from bionic algorithms
人工智能可以从仿生算法中学到什么
- DOI:--
- 发表时间:2019
- 期刊:Physics of Life Reviews
- 影响因子:11.7
- 作者:Chang-Bing Tang;Yan Zhang;Lin Wang;Zhao Zhang
- 通讯作者:Zhao Zhang
Discontinuous phase transition in an annealed multi-state majority-vote model
退火多态多数表决模型中的不连续相变
- DOI:10.1088/1742-5468/2016/07/073403
- 发表时间:2016-07-01
- 期刊:JOURNAL OF STATISTICAL MECHANICS-THEORY AND EXPERIMENT
- 影响因子:2.4
- 作者:Li, Guofeng;Chen, Hanshuang;Shen, Chuansheng
- 通讯作者:Shen, Chuansheng
Heterogeneous nucleation on complex networks with mobile impurities
具有移动杂质的复杂网络上的异质成核
- DOI:--
- 发表时间:2015
- 期刊:Europhysics Letters
- 影响因子:--
- 作者:Chuansheng Shen;Hanshuang Chen;Zhonghuai Hou
- 通讯作者:Zhonghuai Hou
Large deviation induced phase switch in an inertial majority-vote model
惯性多数表决模型中的大偏差引起的相位切换
- DOI:10.1063/1.4993520
- 发表时间:2017-08-01
- 期刊:CHAOS
- 影响因子:2.9
- 作者:Chen, Hanshuang;Shen, Chuansheng;Kurths, Juergen
- 通讯作者:Kurths, Juergen
数据更新时间:{{ journalArticles.updateTime }}
{{
item.title }}
{{ item.translation_title }}
- DOI:{{ item.doi || "--"}}
- 发表时间:{{ item.publish_year || "--" }}
- 期刊:{{ item.journal_name }}
- 影响因子:{{ item.factor || "--"}}
- 作者:{{ item.authors }}
- 通讯作者:{{ item.author }}
数据更新时间:{{ journalArticles.updateTime }}
{{ item.title }}
- 作者:{{ item.authors }}
数据更新时间:{{ monograph.updateTime }}
{{ item.title }}
- 作者:{{ item.authors }}
数据更新时间:{{ sciAawards.updateTime }}
{{ item.title }}
- 作者:{{ item.authors }}
数据更新时间:{{ conferencePapers.updateTime }}
{{ item.title }}
- 作者:{{ item.authors }}
数据更新时间:{{ patent.updateTime }}
其他文献
Ne-HF体系的相互作用势及散射截面的密耦计算
- DOI:--
- 发表时间:--
- 期刊:物理学报
- 影响因子:--
- 作者:申传胜;余春日;程新路;杨向东;宋晓书
- 通讯作者:宋晓书
其他文献
{{
item.title }}
{{ item.translation_title }}
- DOI:{{ item.doi || "--" }}
- 发表时间:{{ item.publish_year || "--"}}
- 期刊:{{ item.journal_name }}
- 影响因子:{{ item.factor || "--" }}
- 作者:{{ item.authors }}
- 通讯作者:{{ item.author }}
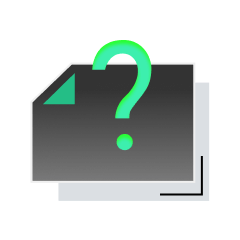
内容获取失败,请点击重试
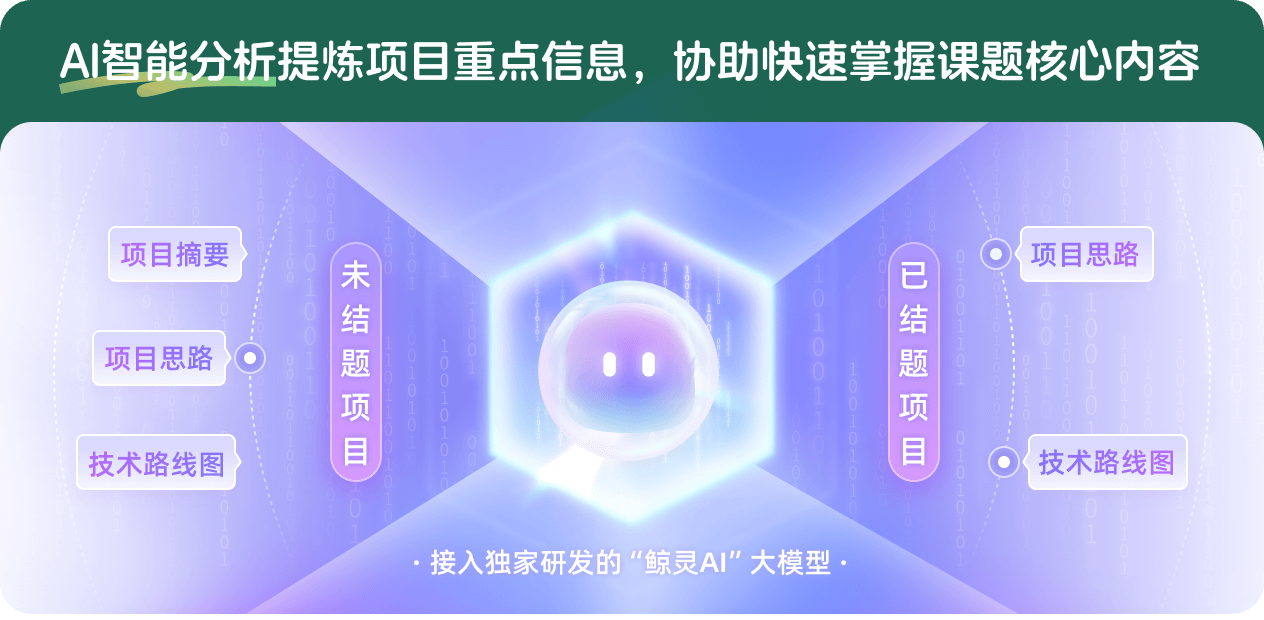
查看分析示例
此项目为已结题,我已根据课题信息分析并撰写以下内容,帮您拓宽课题思路:
AI项目摘要
AI项目思路
AI技术路线图
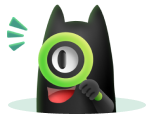
请为本次AI项目解读的内容对您的实用性打分
非常不实用
非常实用
1
2
3
4
5
6
7
8
9
10
您认为此功能如何分析更能满足您的需求,请填写您的反馈:
申传胜的其他基金
网络上动力学过程的大偏差理论及其在生态系统中的应用
- 批准号:
- 批准年份:2020
- 资助金额:10 万元
- 项目类别:国际(地区)合作与交流项目
复杂生物种群网络多稳性和引爆点的机理与预测研究
- 批准号:11975025
- 批准年份:2019
- 资助金额:60 万元
- 项目类别:面上项目
相似国自然基金
{{ item.name }}
- 批准号:{{ item.ratify_no }}
- 批准年份:{{ item.approval_year }}
- 资助金额:{{ item.support_num }}
- 项目类别:{{ item.project_type }}
相似海外基金
{{
item.name }}
{{ item.translate_name }}
- 批准号:{{ item.ratify_no }}
- 财政年份:{{ item.approval_year }}
- 资助金额:{{ item.support_num }}
- 项目类别:{{ item.project_type }}