多元计数数据的统计过程控制方法研究
项目介绍
AI项目解读
基本信息
- 批准号:71272003
- 项目类别:面上项目
- 资助金额:48.0万
- 负责人:
- 依托单位:
- 学科分类:G0108.工业工程与质量管理
- 结题年份:2016
- 批准年份:2012
- 项目状态:已结题
- 起止时间:2013-01-01 至2016-12-31
- 项目参与者:宗福季; 周勤; 王猛; 肯特; 茅桠捷;
- 关键词:
项目摘要
Thanks to rapid advances in sensing and information technologies, automatic data acquisition techniques are now commonly used in many modern manufacturing processes,and a large amount of data and information related to quality measurements has become available. Among the numerous data information, multivariate count data arises in many disciplines: the numbers of different types of surface and interiors defects of products over time, the number of faults in parts, or different types of accidents over time. In order to simultaneously evaluating several types of quality defects, it is appropriate and necessary to apply modern multivariate quality control methodology. Usually, it is assumed that the quality characteristics of multiple counts process follow either independent Poisson or multivariate Poisson distribution. Traditional statistical quality control schemes tend to monitor each count variable separately or utilize a few quality control charts designed for multivariate Poisson distribution. These approaches either lack of deep insight into the underlying structure of the count data distribution or ignore the interrelationship between the count variables. Consequently, the false alarm rate of the control schemes will be greatly inflated which leads to excessive false alarms. Therefore, in order to provide more powerful and sensitive control schemes for monitoring multivariate count data, we aim to investigate the design of dynamic control schemes (varying sampling interval and varying sample size) of CUSUM and EWMA control charts of the univariate count data; Secondly, by utilizing various types of parametric and nonparametric multivariate statistical analysis methods, such as Poisson regression tree, multivariate generalized linear model and kernel density estimation approach, we aim to design statistical control charts for complicated multivariate count data. Last but not the least, we also consider the over-dispersed count data which is quite common in modern high-quality manufacturing industry. Poisson Log-normal distribution and Poisson Log-normal regression model are proposed to model the underlying distribution of the multivariate count data. Monte Carlo Markov chain algorithm is proposed to provide efficient and recursive estimation of the parameters of the model, based on which various types of control charts are designed and investigated, including profile monitoring control charts for the surveillance the coefficients of the Poisson Log-normal regression model and robust multivariate control charts for monitoring the mean vector and covariance matrices of the underlying distribution.
在现代生产制造业中,借助发达的数据采集设备和计算机辅助系统,可以同时对多个质量指标进行记录和监控,其中包括众多计数型质量数据。基于此类质量数据,利用统计过程控制技术对生产质量和过程进监控一直是学术界和企业界长期研究的重点课题。目前,对计数型数据的统计质量控制方法的研究还不尽完善。传统质量控制的方法或是在每个质量指标应用传统的属性控制图,或是应用少数几种基于多元泊松分布的联合控制图。此类方法对计数数据的分布和模型缺乏深入研究,极有可能忽视或误判多元质量变量之间的相关性,从而大大增加统计过程监控方法的误报率,降低整体检验功效。本课题主要针对电子制造业中的计数型质量数据,提出一元计数型控制图的动态设计方法;基于泊松回归树、广义线性模型和核密度估计等方法,解决复杂多变量情况下的多元计数数据的质量监控问题。除此以外,对电子制造业中普遍存在的过离散型多元计数数据,提出新的建模方法和监控思路。
结项摘要
本项目针对多工序制造过程中广泛存在的离散型和混合型数据,研究新的建模、参数估计、以及质量预测、监控与故障诊断方法。本项目具体从下面三个方面展开研究:第一,离散型和混合型质量数据模型的研究;第二,基于离散型和混合型质量数据模型的过程监控方案;第三,基于离散型和混合型质量数据模型的故障诊断方法。通过上述三个任务的完成,本研究给出多工序制造过程中离散型和混合型质量数据的理论建模方法,同时提供统计过程控制以及故障诊断方案。本人在该项目的研究工作中目前在该项目的研究工作中,第一作者发表国际知名SCI 期刊论文4 篇(IIE Transactions,International Journal of Productions Research,Renewable energy,Applied Energy);和本人研究生合作,第二作者SCI 论文1 篇,第四作者SCI 论文1 篇(IEEE Intelligent Systems Special Issue on System Informatics)。培养硕士研究生9 名,本科生11名。
项目成果
期刊论文数量(8)
专著数量(0)
科研奖励数量(0)
会议论文数量(0)
专利数量(0)
高维数据均值的统计监测
- DOI:--
- 发表时间:2015
- 期刊:数理统计与管理
- 影响因子:--
- 作者:茅桠捷;李艳婷
- 通讯作者:李艳婷
Projection-Based Process Monitoring and Empirical Divergence
基于投影的过程监控和经验分歧
- DOI:--
- 发表时间:2015
- 期刊:IEEE Intelligent Systems
- 影响因子:6.4
- 作者:Wei, Qingming;Huang, Wenpo;Jiang, Wei;Li, Yanting
- 通讯作者:Li, Yanting
Forecasting the daily power output of a grid-connected photovoltaic system based on multivariate adaptive regression splines
基于多元自适应回归样条的并网光伏系统日发电量预测
- DOI:10.1016/j.apenergy.2016.07.052
- 发表时间:2016-10-15
- 期刊:APPLIED ENERGY
- 影响因子:11.2
- 作者:Li, Yanting;He, Yong;Shu, Lianjie
- 通讯作者:Shu, Lianjie
An ARMAX model for forecasting the power output of a grid connected photovoltaic system
用于预测并网光伏系统功率输出的 ARMAX 模型
- DOI:10.1016/j.renene.2013.11.067
- 发表时间:2014-06-01
- 期刊:RENEWABLE ENERGY
- 影响因子:8.7
- 作者:Li, Yanting;Su, Yan;Shu, Lianjie
- 通讯作者:Shu, Lianjie
高维泊松数据流的统计过程控制图
- DOI:--
- 发表时间:--
- 期刊:International Journal of Quality Engineering and Reliability
- 影响因子:--
- 作者:Zhiyuan Wang;Yanting LI
- 通讯作者:Yanting LI
数据更新时间:{{ journalArticles.updateTime }}
{{
item.title }}
{{ item.translation_title }}
- DOI:{{ item.doi || "--"}}
- 发表时间:{{ item.publish_year || "--" }}
- 期刊:{{ item.journal_name }}
- 影响因子:{{ item.factor || "--"}}
- 作者:{{ item.authors }}
- 通讯作者:{{ item.author }}
数据更新时间:{{ journalArticles.updateTime }}
{{ item.title }}
- 作者:{{ item.authors }}
数据更新时间:{{ monograph.updateTime }}
{{ item.title }}
- 作者:{{ item.authors }}
数据更新时间:{{ sciAawards.updateTime }}
{{ item.title }}
- 作者:{{ item.authors }}
数据更新时间:{{ conferencePapers.updateTime }}
{{ item.title }}
- 作者:{{ item.authors }}
数据更新时间:{{ patent.updateTime }}
其他文献
Finite extension of generalized Bessel sequences to generalized frames
广义贝塞尔序列到广义框架的有限推广
- DOI:--
- 发表时间:2018
- 期刊:Acta Mathematica Scientia
- 影响因子:1
- 作者:李登峰;李艳婷
- 通讯作者:李艳婷
面向未知自相关过程的Bootstrap控制图设计
- DOI:--
- 发表时间:2018
- 期刊:工业工程
- 影响因子:--
- 作者:娄璐;李艳婷
- 通讯作者:李艳婷
基于SVD和ARIMA的时空序列分解和预测
- DOI:10.19678/j.issn.1000-3428.0056885
- 发表时间:2020
- 期刊:计算机工程
- 影响因子:--
- 作者:杨立宁;李艳婷
- 通讯作者:李艳婷
基于CNN-集成学习的多风机故障诊断
- DOI:--
- 发表时间:--
- 期刊:工业工程
- 影响因子:--
- 作者:叶祎旎;李艳婷
- 通讯作者:李艳婷
不同O2O模式下供应链服务质量控制
- DOI:10.12068/j.issn.1005-3026.2019.10.024
- 发表时间:2019
- 期刊:东北大学学报. 自然科学版
- 影响因子:--
- 作者:李艳婷;张翠华;李春雨;吕茹霞
- 通讯作者:吕茹霞
其他文献
{{
item.title }}
{{ item.translation_title }}
- DOI:{{ item.doi || "--" }}
- 发表时间:{{ item.publish_year || "--"}}
- 期刊:{{ item.journal_name }}
- 影响因子:{{ item.factor || "--" }}
- 作者:{{ item.authors }}
- 通讯作者:{{ item.author }}
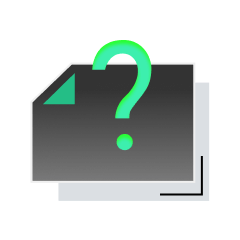
内容获取失败,请点击重试
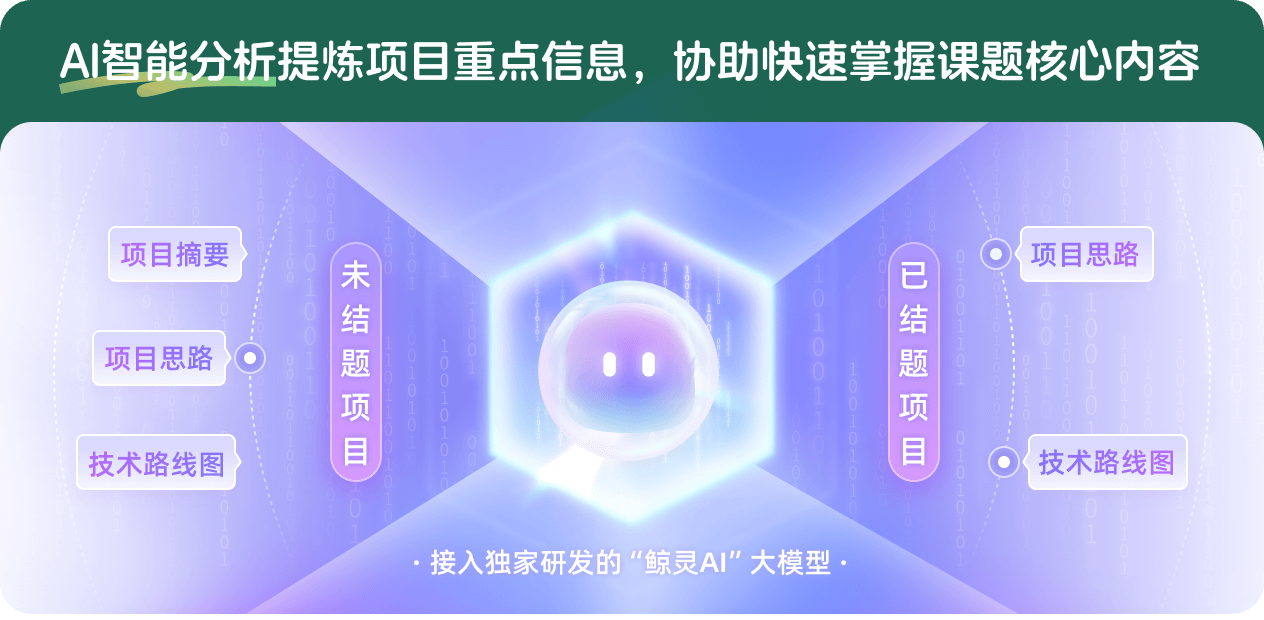
查看分析示例
此项目为已结题,我已根据课题信息分析并撰写以下内容,帮您拓宽课题思路:
AI项目摘要
AI项目思路
AI技术路线图
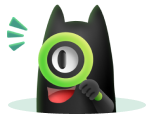
请为本次AI项目解读的内容对您的实用性打分
非常不实用
非常实用
1
2
3
4
5
6
7
8
9
10
您认为此功能如何分析更能满足您的需求,请填写您的反馈:
李艳婷的其他基金
复杂数据环境下大规模风力发电场的功率预测、状态监控和故障诊断
- 批准号:
- 批准年份:2020
- 资助金额:48 万元
- 项目类别:面上项目
多元复杂时空数据建模与监控方法研究
- 批准号:71672109
- 批准年份:2016
- 资助金额:48.0 万元
- 项目类别:面上项目
多工序制造过程中离散型和混合型质量数据的统计过程监控方法研究
- 批准号:70902070
- 批准年份:2009
- 资助金额:18.0 万元
- 项目类别:青年科学基金项目
相似国自然基金
{{ item.name }}
- 批准号:{{ item.ratify_no }}
- 批准年份:{{ item.approval_year }}
- 资助金额:{{ item.support_num }}
- 项目类别:{{ item.project_type }}
相似海外基金
{{
item.name }}
{{ item.translate_name }}
- 批准号:{{ item.ratify_no }}
- 财政年份:{{ item.approval_year }}
- 资助金额:{{ item.support_num }}
- 项目类别:{{ item.project_type }}