小概率失效下基于主动学习Kriging模型的可靠性分析方法研究
项目介绍
AI项目解读
基本信息
- 批准号:51705433
- 项目类别:青年科学基金项目
- 资助金额:25.0万
- 负责人:
- 依托单位:
- 学科分类:E0504.机械结构强度学
- 结题年份:2020
- 批准年份:2017
- 项目状态:已结题
- 起止时间:2018-01-01 至2020-12-31
- 项目参与者:周张义; 李金城; 桑平; 王林海; 邹纪操; 刘志伟;
- 关键词:
项目摘要
Active learning Kriging (ALK) model chooses training points in a demand-oriented way. During approximating the performance function by an ALK model, much attention can be focused on the vicinity of the limit state. Therefore, reliability analysis with ALK model is capable of obtaining a highly precise result with calling the performance function as few times as possible. However, during addressing rare event estimation, ALK model suffers from several fatal challenges that: purely building the ALK model is pretty time-consuming; it lacks an efficient parallel computing strategy; system reliability analysis with multiple failure modes remains an issue. In this project, we will firstly investigate the fusion of ALK model and the importance sampling method. Two novel strategies will be proposed to build an ALK model focusing on identifying the most probable failure regions of a performance function. The ALK model will be continually updated by treating important samples as candidate points. Consequently, the computational costs purely spent on ALK model can be remarkably reduced. The superiority of ALK model in efficiency and accuracy can be inherited at the same time. Secondly, combining with an efficient strategy of adding training points in parallelization and a brand-new scheme of adding training points for a system, the proposed methods are adjusted to the parallel compute environment and system reliability analysis with multiple failure modes. Finally, reliability analysis of a rail vehicle axle will be performed to verify the effectiveness of the proposed methods. Accordingly, some advices will be offered to improve the reliability of the axle.
主动学习Kriging(ALK)模型以需求为导向挑选训练点,能够仅仅在极限状态平面附近的狭小区域对功能函数进行近似。因此,基于ALK模型的可靠性分析方法能够在保证精度的前提下尽量少地调用结构的功能函数。但是,在处理小概率失效问题时,ALK模型遭遇了巨大挑战:建立ALK模型耗费时间过长;缺乏高效的并行策略;难以适用于多模式系统可靠性分析。本课题首先开展ALK模型与重要抽样方法融合技术的研究,提出两种全新的策略,以识别功能函数的最可能失效区域为导向建立ALK模型,并以重要抽样仿真样本作为候选点继续更新ALK模型。从而在保持ALK模型高效高精度的同时,显著缩短建立ALK模型的计算时间。而后结合高效的并行加点策略和全新的系统加点策略,实现所提方法在分布式并行运算环境以及多模式系统可靠性分析中的应用。最后开展轨道车辆车轴的可靠性分析,验证所提方法高效性的同时,为提高车轴的可靠性提供参考意见。
结项摘要
研究高效高精度的计算方法是当前结构可靠性分析领域的重中之重。基于主动学习Kriging(ALK)模型的可靠性分析方法能够在保证精度的前提下尽量少地调用结构的功能函数。但是,在处理小概率失效问题时,已有方法基于ALK模型和Monte Carlo仿真,面临了难以克服的挑战。本项目针对ALK模型在处理小概率失效问题时所面临的困境,开展了融合ALK模型与多种重要抽样法的研究工作。项目研究人员根据各种重要抽样法各自的特点,提出了基于同心圆环逼近、自适应重要抽样区、多模优化算法等多种策略,高效识别最可能失效区域并添加最优训练点。此外,结合自适应截断候选区和自适应候选点的系统可靠性分析策略,实现了所提方法在多失效模式系统可靠性分析中的应用。基于上述研究,项目研究人员发表论文9篇。其中,SCI论文7篇,且多数发表在Reliability Engineering and System Safety、Structural and Multidisciplinary Optimization、International Journal for Numerical Methods In Engineering 等本领域内知名刊物上。项目研究成果为复杂工程领域,功能函数存在多失效区域、多失效模式、失效概率极小的中低维问题提供了一整套切实可行的解决方案,在航空航天、轨道交通、土木工程等领域具有较为广阔的应用前景。
项目成果
期刊论文数量(8)
专著数量(0)
科研奖励数量(0)
会议论文数量(1)
专利数量(0)
Active Learning Kriging Model Combining With Kernel-Density-Estimation-Based Importance Sampling Method for the Estimation of Low Failure Probability
主动学习克里金模型结合基于核密度估计的重要性采样方法进行低故障概率估计
- DOI:10.1115/1.4039339
- 发表时间:2018-05
- 期刊:Journal of Mechanical Design
- 影响因子:3.3
- 作者:Yang Xufeng;Liu Yongshou;Mi Caiying;Wang Xiangjin
- 通讯作者:Wang Xiangjin
基于改进Kriging模型的主动学习可靠性分析方法
- DOI:--
- 发表时间:2021
- 期刊:机械强度,2021,43(01):129-136.
- 影响因子:--
- 作者:陈哲;杨旭锋;程鑫
- 通讯作者:程鑫
A system reliability analysis method combining active learning Kriging model with adaptive size of candidate points
主动学习Kriging模型与候选点大小自适应相结合的系统可靠性分析方法
- DOI:10.1007/s00158-019-02205-x
- 发表时间:2019-02
- 期刊:Structural and Multidisciplinary Optimization
- 影响因子:3.9
- 作者:Yang Xufeng;Mi Caiying;Deng Dingyuan;Liu Yongshou
- 通讯作者:Liu Yongshou
Bounds approximation of limit-state surface based on active learning Kriging model with truncated candidate region for random-interval hybrid reliability analysis
基于主动学习克里金模型的随机区间混合可靠性分析截断候选区域的极限状态面边界逼近
- DOI:10.1002/nme.6269
- 发表时间:2020
- 期刊:International Journal for Numerical Methods in Engineering
- 影响因子:2.9
- 作者:Yang Xufeng;Wang Tai;Li Jincheng;Chen Zhang
- 通讯作者:Chen Zhang
System reliability analysis through active learning Kriging model with truncated candidate region
通过主动学习截断候选区域的克里金模型进行系统可靠性分析
- DOI:10.1016/j.ress.2017.08.016
- 发表时间:2018
- 期刊:Reliability Engineering & System Safety
- 影响因子:8.1
- 作者:Yang Xufeng;Liu Yongshou;Mi Caiying;Tang Chenghu
- 通讯作者:Tang Chenghu
数据更新时间:{{ journalArticles.updateTime }}
{{
item.title }}
{{ item.translation_title }}
- DOI:{{ item.doi || "--"}}
- 发表时间:{{ item.publish_year || "--" }}
- 期刊:{{ item.journal_name }}
- 影响因子:{{ item.factor || "--"}}
- 作者:{{ item.authors }}
- 通讯作者:{{ item.author }}
数据更新时间:{{ journalArticles.updateTime }}
{{ item.title }}
- 作者:{{ item.authors }}
数据更新时间:{{ monograph.updateTime }}
{{ item.title }}
- 作者:{{ item.authors }}
数据更新时间:{{ sciAawards.updateTime }}
{{ item.title }}
- 作者:{{ item.authors }}
数据更新时间:{{ conferencePapers.updateTime }}
{{ item.title }}
- 作者:{{ item.authors }}
数据更新时间:{{ patent.updateTime }}
其他文献
三维机织复合材料残余应力/应变多尺度分析及工艺参数优化
- DOI:--
- 发表时间:2021
- 期刊:复合材料学报
- 影响因子:--
- 作者:王琦;蒋秋梅;杨旭锋;任明法
- 通讯作者:任明法
基于螺旋相位调制的非相干全息点扩散函数研究
- DOI:--
- 发表时间:2018
- 期刊:物理学报
- 影响因子:--
- 作者:赵忠超;杨旭锋;许天旭;何九如;弓巧侠;杜艳丽;董林;袁斌;马凤英
- 通讯作者:马凤英
其他文献
{{
item.title }}
{{ item.translation_title }}
- DOI:{{ item.doi || "--" }}
- 发表时间:{{ item.publish_year || "--"}}
- 期刊:{{ item.journal_name }}
- 影响因子:{{ item.factor || "--" }}
- 作者:{{ item.authors }}
- 通讯作者:{{ item.author }}
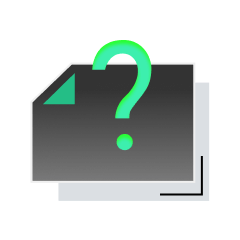
内容获取失败,请点击重试
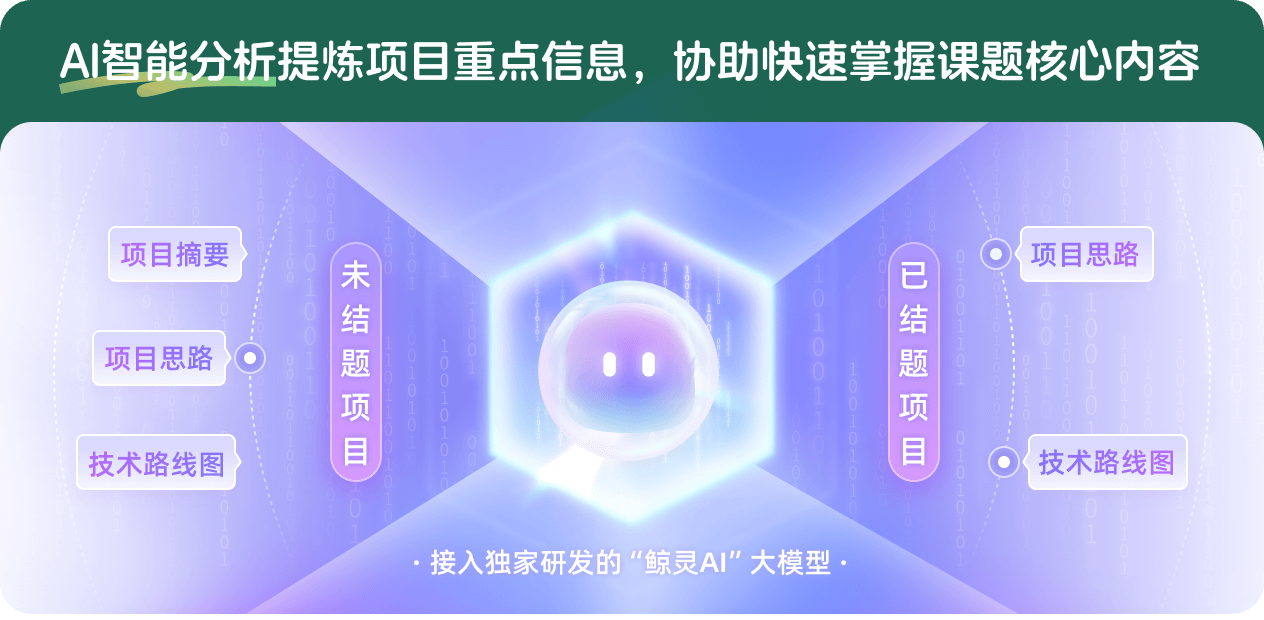
查看分析示例
此项目为已结题,我已根据课题信息分析并撰写以下内容,帮您拓宽课题思路:
AI项目摘要
AI项目思路
AI技术路线图
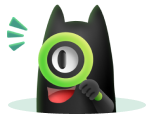
请为本次AI项目解读的内容对您的实用性打分
非常不实用
非常实用
1
2
3
4
5
6
7
8
9
10
您认为此功能如何分析更能满足您的需求,请填写您的反馈:
相似国自然基金
{{ item.name }}
- 批准号:{{ item.ratify_no }}
- 批准年份:{{ item.approval_year }}
- 资助金额:{{ item.support_num }}
- 项目类别:{{ item.project_type }}
相似海外基金
{{
item.name }}
{{ item.translate_name }}
- 批准号:{{ item.ratify_no }}
- 财政年份:{{ item.approval_year }}
- 资助金额:{{ item.support_num }}
- 项目类别:{{ item.project_type }}