基于低复杂度多任务稀疏贝叶斯学习的波达方向估计研究
项目介绍
AI项目解读
基本信息
- 批准号:61901482
- 项目类别:青年科学基金项目
- 资助金额:24.0万
- 负责人:
- 依托单位:
- 学科分类:F0112.雷达原理与技术
- 结题年份:2022
- 批准年份:2019
- 项目状态:已结题
- 起止时间:2020-01-01 至2022-12-31
- 项目参与者:--
- 关键词:
项目摘要
The project addresses the DOA estimation problems for multiple-input-multiple-output (MIMO) radar. Using multi-task sparse Bayesian learning theory and estimation methods based on probabilistic graphical model, the following three aspects will be studied in detail in the project, 1) DOA estimation methods based on multi-task sparse Bayesian learning, which aims to solve the problem of insufficient estimation precision under the circumstance of low signal-to-noise ratio (SNR) and small number of snapshots, using traditional methods designed for single receiving array; 2) fast Bayesian learning methods based on approximate message passing (AMP), which aims to solve the problem of prohibitively high computational complexity in traditional sparse Bayesian learning algorithms; 3) methods of dictionary optimization in AMP-based multi-task sparse Bayesian learning, which aims to solve the systematical estimation error brought by the off-grid targets. Compared with the conventional studies on DOA estimation methods, the difference of this project is that it focuses on multi-array joint DOA estimation based on sparse Bayesian learning models. It innovatively introduces AMP, a low-complexity optimization method based on probabilistic graphical model, into the framework of sparse Bayesian learning, which will significantly improve the performance of DOA estimation against complex situations. In the meanwhile, the project will also analyze the theoretical performance of the proposed methods and contribute to the current study of compressed sensing theory. In conclusion, the study in this project will provide a technical approach to improve the target localization performance of MIMO radar against complex situations in practice.
本项目以MIMO雷达目标角度估计为背景,利用多任务稀疏贝叶斯学习理论和基于概率图模型的信号估计方法,系统研究(1)基于多任务稀疏贝叶斯学习的角度估计方法,通过多接收阵列信号联合估计的方式解决传统单接收阵列MIMO雷达在低信噪比、小样本数等复杂条件下估计精度不足的问题;(2)基于近似信息传输的快速贝叶斯学习方法,解决传统的稀疏贝叶斯学习算法计算复杂度过高的问题;(3)基于近似信息传输的多任务稀疏贝叶斯学习中的字典优化方法,解决目标方向不在空域角度网格上所带来的系统性估计误差问题。与传统角度估计方法研究不同,本项目将基于稀疏贝叶斯学习的多阵列联合角度估计作为研究对象,创新性的引入近似信息传输这一概率图方法,突破传统的基于稀疏贝叶斯角度估计算法对复杂条件适应能力不足的技术瓶颈。同时,本项目还将研究算法的理论性能分析,完善当前压缩感知理论体系,为提升MIMO雷达在复杂应用环境中的效能提供技术途径。
结项摘要
目标角度估计(Direction of Arrival, DOA)问题是雷达目标定位中的重要环节。本项目针对雷达目标角度估计中存在的小样本、低信噪比、离线目标和旁瓣强干扰的问题,开展了基于低复杂度多任务稀疏贝叶斯学习的波达方向估计研究。本项目通过引入多任务稀疏贝叶斯学习方法,实现了双站雷达角度估计,以获取相较于传统单站雷达更高的角度估计精度。首先,本项目在双站雷达接收数据统计模型的基础上,利用稀疏贝叶斯学习的方法推导出了目标角度的估计算子,提出了一种联合双站雷达接收数据的多任务稀疏贝叶斯学习角度估计算法。在该算法的计算过程中,由于传统稀疏贝叶斯学习存在计算复杂度过高的问题,本项目进一步引入近似消息传递算法来解算稀疏贝叶斯学习中的后验概率密度,以此来兼顾计算精度与实时性。而为了提高以上算法对离线目标的角度估计精度,本项目还提出将字典矩阵与目标角度进行联合优化的算法,增强了稀疏贝叶斯角度估计方法的鲁棒性。最后,考虑到复杂对抗环境下常见的旁瓣强干扰现象,本项目提出了一种强干扰条件下基于稀疏恢复的主瓣目标角度估计算法,以自适应地抑制旁瓣干扰,进一步提高了整套算法实践应用的可能性。项目组成员通过仿真实验结果验证了本研究所提算法优于传统DOA估计算法的性能,又利用雷达实测数据进行实验,验证了所提算法的有效性和先进性。本项目的研究成果对从理论上解决复杂环境条件下的目标角度估计问题具有重要意义,同时也对推动稀疏恢复算法在目标角度估计领域的工程实用化具有重要作用。
项目成果
期刊论文数量(1)
专著数量(0)
科研奖励数量(1)
会议论文数量(2)
专利数量(10)
基于广义近似消息传递的快速DOA估计方法
- DOI:10.12305/j.issn.1001-506x.2022.10.01
- 发表时间:2022
- 期刊:系统工程与电子技术
- 影响因子:--
- 作者:张俊;张新禹;姜卫东;刘永祥;黎湘
- 通讯作者:黎湘
数据更新时间:{{ journalArticles.updateTime }}
{{
item.title }}
{{ item.translation_title }}
- DOI:{{ item.doi || "--"}}
- 发表时间:{{ item.publish_year || "--" }}
- 期刊:{{ item.journal_name }}
- 影响因子:{{ item.factor || "--"}}
- 作者:{{ item.authors }}
- 通讯作者:{{ item.author }}
数据更新时间:{{ journalArticles.updateTime }}
{{ item.title }}
- 作者:{{ item.authors }}
数据更新时间:{{ monograph.updateTime }}
{{ item.title }}
- 作者:{{ item.authors }}
数据更新时间:{{ sciAawards.updateTime }}
{{ item.title }}
- 作者:{{ item.authors }}
数据更新时间:{{ conferencePapers.updateTime }}
{{ item.title }}
- 作者:{{ item.authors }}
数据更新时间:{{ patent.updateTime }}
其他文献
其他文献
{{
item.title }}
{{ item.translation_title }}
- DOI:{{ item.doi || "--" }}
- 发表时间:{{ item.publish_year || "--"}}
- 期刊:{{ item.journal_name }}
- 影响因子:{{ item.factor || "--" }}
- 作者:{{ item.authors }}
- 通讯作者:{{ item.author }}
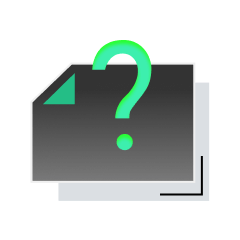
内容获取失败,请点击重试
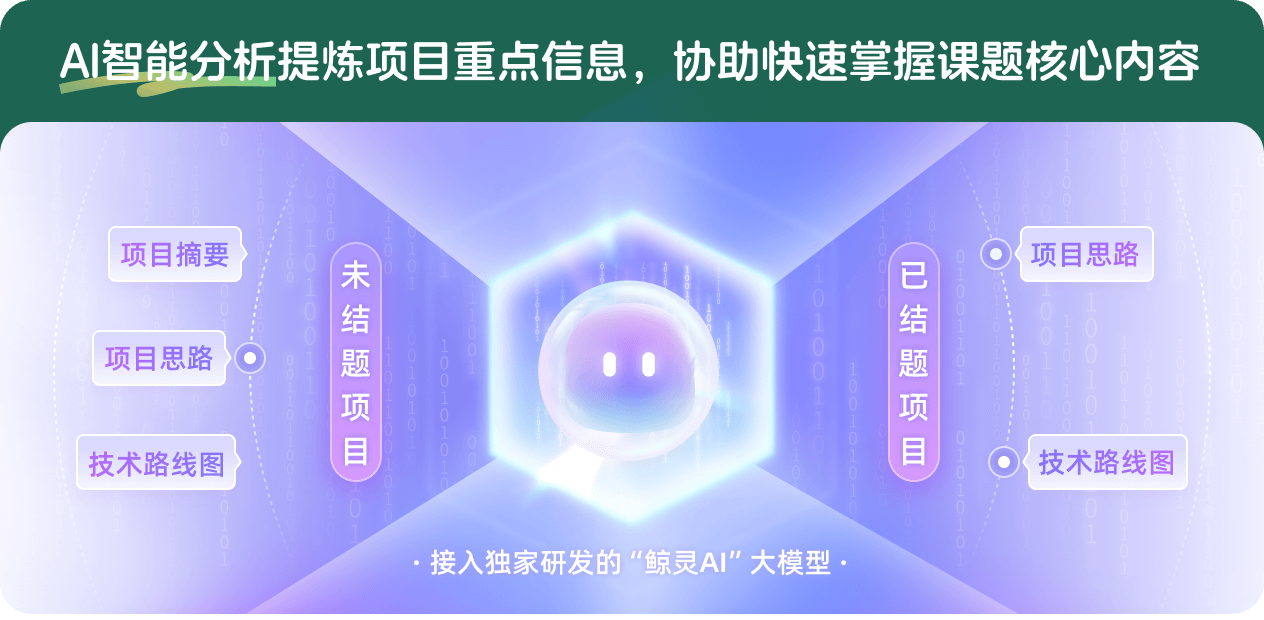
查看分析示例
此项目为已结题,我已根据课题信息分析并撰写以下内容,帮您拓宽课题思路:
AI项目摘要
AI项目思路
AI技术路线图
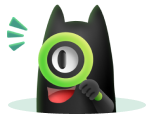
请为本次AI项目解读的内容对您的实用性打分
非常不实用
非常实用
1
2
3
4
5
6
7
8
9
10
您认为此功能如何分析更能满足您的需求,请填写您的反馈:
相似国自然基金
{{ item.name }}
- 批准号:{{ item.ratify_no }}
- 批准年份:{{ item.approval_year }}
- 资助金额:{{ item.support_num }}
- 项目类别:{{ item.project_type }}
相似海外基金
{{
item.name }}
{{ item.translate_name }}
- 批准号:{{ item.ratify_no }}
- 财政年份:{{ item.approval_year }}
- 资助金额:{{ item.support_num }}
- 项目类别:{{ item.project_type }}