奥氏体不锈钢的基本蠕变模型:晶粒尺寸和蠕变孔洞的影响机制
项目介绍
AI项目解读
基本信息
- 批准号:51901061
- 项目类别:青年科学基金项目
- 资助金额:26.0万
- 负责人:
- 依托单位:
- 学科分类:E0103.金属材料使役行为与表面工程
- 结题年份:2022
- 批准年份:2019
- 项目状态:已结题
- 起止时间:2020-01-01 至2022-12-31
- 项目参与者:--
- 关键词:
项目摘要
Phenomenological models based on empirical equations have the shortcomings that the reliability of predicting creep rupture life at long times is low, and they are not applicable for new conditions. Unlike the phenomenological models and continuum creep damage mechanics, fundamental creep models of austenitic stainless steels based on the evolution of microstructure and defects will be proposed in this project. All parameters in the model should be well defined and there should be no adjustable parameters. Based on the previous work, the present work will focus on: 1). Grain size effects on creep rate and its basic models, where the influence of grain boundary sliding, creep cavities and creep cracks will be studied. Afterward, grain size dependent ductile creep rupture models will be established; 2). Evolution of creep cavities will be studied with the help of 3D tomography technology. By exploring the influence of grain boundaries and precipitations, creep cavitation models will be improved. By coupling the grain size dependent ductile creep rupture models with the new brittle creep rupture models, improved fundamental creep models will be established, whereby the creep rupture life of austenitic stainless steels will be predicted. This work will provide a theoretical way of predicting the creep rupture life for materials undergoing high temperatures and stresses at long times. Meanwhile, a theoretical basis will be proposed for predicting the mechanical properties based on the microstructures of materials.
基于经验公式的唯象模型在预测材料长时蠕变寿命时可靠性会下降,且不同材料间普适性不足。不同于唯象模型和连续介质损伤力学模型,本项目拟研究奥氏体不锈钢的基本蠕变模型,即基于微观组织结构和缺陷的演化机制提出一种所涉及参数具有明确物理意义且无可调节参数或拟合参数的理论模型。在前期工作基础上,本项目主要研究内容包括:1)研究晶粒尺寸对蠕变速率的影响机制及其基本模型,探究晶界滑移、蠕变孔洞和蠕变裂纹对晶粒尺寸效应的影响,建立与晶粒尺寸效应有关的韧性蠕变断裂模型;2)结合三维成像技术研究蠕变孔洞的演化机制,探究晶界及析出相等对蠕变孔洞的影响,改善蠕变孔洞模型。通过耦合改善的韧性蠕变断裂模型和脆性蠕变断裂模型,建立一个完善的基本蠕变模型并预测奥氏体不锈钢的蠕变寿命。本项目将为高温承压材料的长时蠕变寿命评估和预测提供一种理论和方法,同时为从微观组织结构到宏观性能的预测提供一种理论基础。
结项摘要
长期服役蠕变寿命评估和预测是应用于火电、核电、石油化工、航空航天等领域的金属结构材料的一项重要性能指标。目前国内外广泛使用的是唯像模型,这些模型中通常有较多的拟合参数或可调节参数,不能揭示材料失效机理,且存在不合理的外推结果。为避免上述不足,本研究工作首次提出了蠕变断裂曲线的一次导数和二次导数的物理约束条件。将约束条件与唯象模型如时间温度参数法(TTP)结合,获得约束TTP模型,可以更为合理的预测和外推材料的长期服役蠕变性能。另一方面,机器学习算法正在被国内外学者广泛使用,用来预测材料的性能,包括蠕变。研究发现,常规机器学习算法预测外推蠕变断裂时,不能产生合理且一直的外推结果。为此,本研究工作首次提出了硬约束神经网络模型和软约束机器学习算法,即将物理约束条件与机器学习算法相结合,从而可以更合理、可靠、稳定的预测蠕变性能。约束TTP模型和约束机器学习算法已被成功用于预测多种金属结构材料的高温蠕变性能。该成果对目前的商用材料及新研发材料具有重要的实际应用意义。同时,为可靠、稳定的预测更长期条件下的蠕变性能,需要从基础上理解材料的失效机理,为此,本研究工作开展了基本蠕变模型的研究,首次提出了约束晶界扩散蠕变孔洞长大模型,提出并建立了有效晶界扩散系数模型。同时,首次提出了应变控制蠕变孔洞长大模型,建立了瞬时蠕变应变模型。两种模型都同时考虑到了蠕变孔洞化和晶粒尺寸对蠕变性能的影响。模型结果可以与实验数据达到较好吻合。结合通过第一性原理计算获得的溶质元素的固溶强化效应和通过MatCalc计算获得的析出强化贡献,即可定量的预测蠕变性能。基本蠕变模型有望用于预测更为长期服役条件下的安全性。该研究为重大工程用材料如汽轮机、涡轮机等材料的长期服役安全性提供了技术指导和理论支撑。
项目成果
期刊论文数量(7)
专著数量(0)
科研奖励数量(0)
会议论文数量(1)
专利数量(2)
Application of Soft Constrained Machine Learning Algorithms for Creep Rupture Prediction of an Austenitic Heat Resistant Steel Sanicro 25
应用软约束机器学习算法预测奥氏体耐热钢 Sanicro 25 的蠕变断裂
- DOI:10.1016/j.jmrt.2022.11.154
- 发表时间:2022
- 期刊:Journal of Materials Research and Technology
- 影响因子:--
- 作者:Jun-Jing He;Rolf S;ström;Jing Zhang;Hai-Ying Qin
- 通讯作者:Hai-Ying Qin
Application of Fundamental Models for Creep Rupture Prediction of Sanicro 25 (23Cr25NiWCoCu)
基本模型在 Sanicro 25 (23Cr25NiWCoCu) 蠕变断裂预测中的应用
- DOI:10.1016/j.scitotenv.2016.10.188
- 发表时间:2019
- 期刊:Crystals
- 影响因子:2.7
- 作者:Junjing He;Rolf S;ström
- 通讯作者:ström
First-principles modeling of solute effects on thermal properties of nickel alloys
溶质对镍合金热性能影响的第一性原理建模
- DOI:10.1016/j.mtcomm.2021.102551
- 发表时间:2021
- 期刊:Materials Today Communications
- 影响因子:3.8
- 作者:Jing Zhang;Pavel A. Korzhavyi;Junjing He
- 通讯作者:Junjing He
Error estimates in extrapolation of creep rupture data and its application to an austenitic stainless steel
蠕变断裂数据外推的误差估计及其在奥氏体不锈钢中的应用
- DOI:10.1080/09603409.2022.2040184
- 发表时间:2022
- 期刊:Materials at High Temperatures
- 影响因子:1.3
- 作者:Rolf S;ström;Jun-Jing He
- 通讯作者:Jun-Jing He
Creep rupture prediction using constrained neural networks with error estimates
使用带有误差估计的约束神经网络进行蠕变断裂预测
- DOI:10.1080/09603409.2022.2078147
- 发表时间:2022
- 期刊:Materials at High Temperatures
- 影响因子:1.3
- 作者:Jun-Jing He;Rolf S;ström
- 通讯作者:ström
数据更新时间:{{ journalArticles.updateTime }}
{{
item.title }}
{{ item.translation_title }}
- DOI:{{ item.doi || "--"}}
- 发表时间:{{ item.publish_year || "--" }}
- 期刊:{{ item.journal_name }}
- 影响因子:{{ item.factor || "--"}}
- 作者:{{ item.authors }}
- 通讯作者:{{ item.author }}
数据更新时间:{{ journalArticles.updateTime }}
{{ item.title }}
- 作者:{{ item.authors }}
数据更新时间:{{ monograph.updateTime }}
{{ item.title }}
- 作者:{{ item.authors }}
数据更新时间:{{ sciAawards.updateTime }}
{{ item.title }}
- 作者:{{ item.authors }}
数据更新时间:{{ conferencePapers.updateTime }}
{{ item.title }}
- 作者:{{ item.authors }}
数据更新时间:{{ patent.updateTime }}
其他文献
其他文献
{{
item.title }}
{{ item.translation_title }}
- DOI:{{ item.doi || "--" }}
- 发表时间:{{ item.publish_year || "--"}}
- 期刊:{{ item.journal_name }}
- 影响因子:{{ item.factor || "--" }}
- 作者:{{ item.authors }}
- 通讯作者:{{ item.author }}
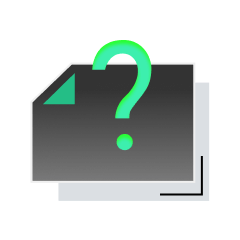
内容获取失败,请点击重试
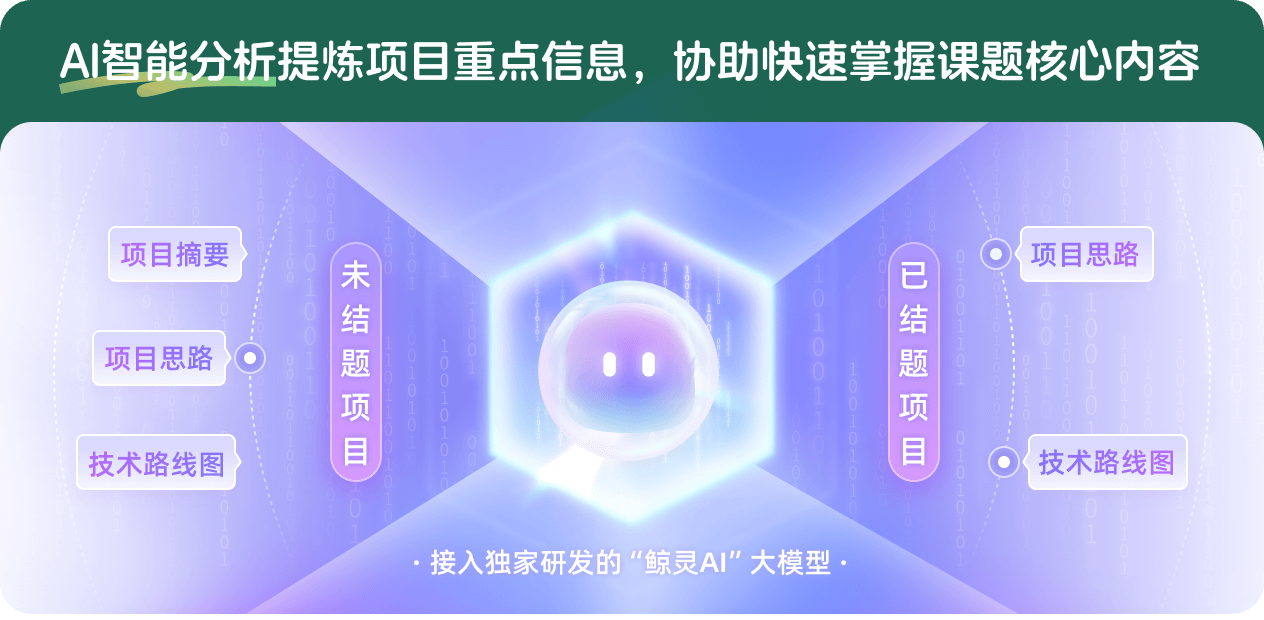
查看分析示例
此项目为已结题,我已根据课题信息分析并撰写以下内容,帮您拓宽课题思路:
AI项目摘要
AI项目思路
AI技术路线图
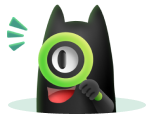
请为本次AI项目解读的内容对您的实用性打分
非常不实用
非常实用
1
2
3
4
5
6
7
8
9
10
您认为此功能如何分析更能满足您的需求,请填写您的反馈:
相似国自然基金
{{ item.name }}
- 批准号:{{ item.ratify_no }}
- 批准年份:{{ item.approval_year }}
- 资助金额:{{ item.support_num }}
- 项目类别:{{ item.project_type }}
相似海外基金
{{
item.name }}
{{ item.translate_name }}
- 批准号:{{ item.ratify_no }}
- 财政年份:{{ item.approval_year }}
- 资助金额:{{ item.support_num }}
- 项目类别:{{ item.project_type }}